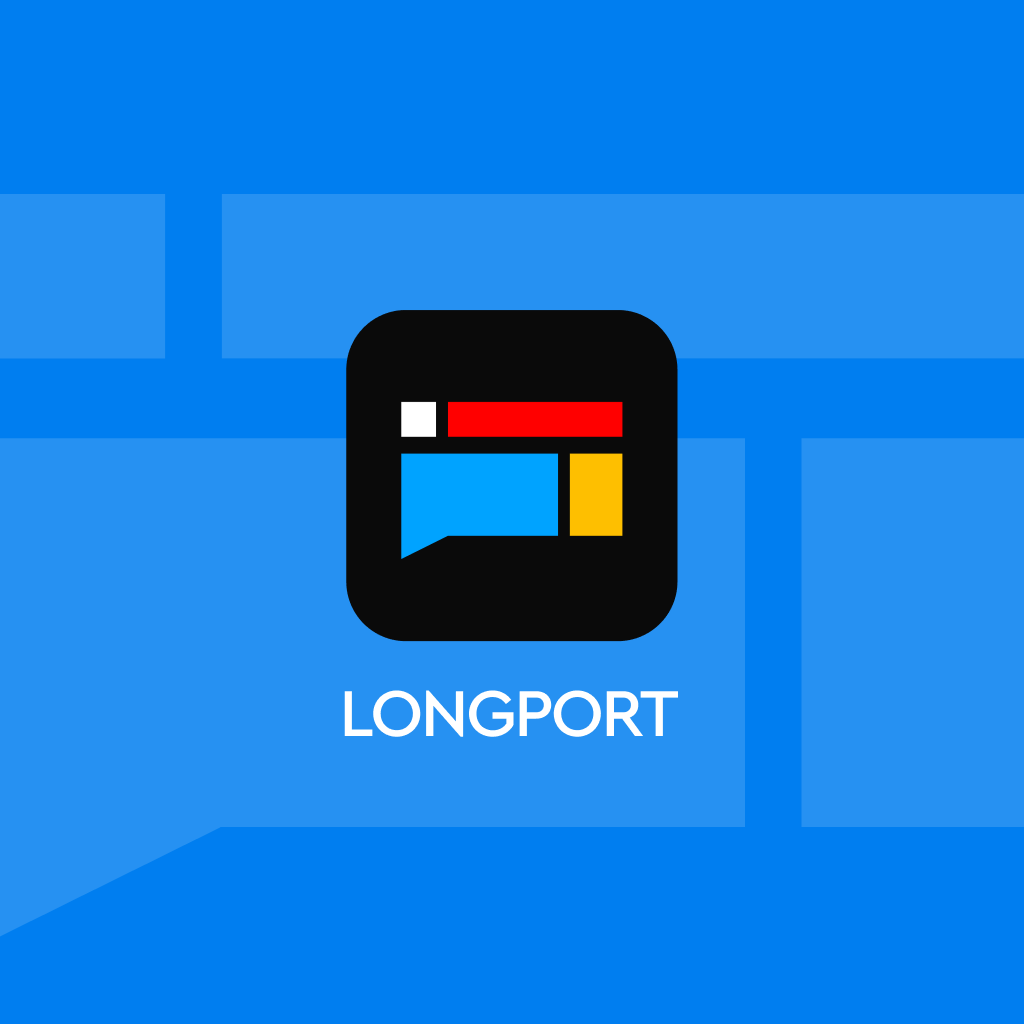
AI progress slowing down, encountering bottlenecks? Leading companies like OpenAI: Not true!
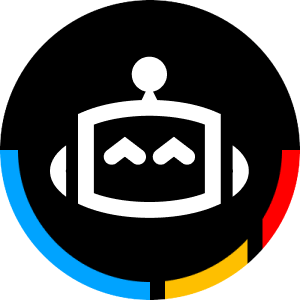
The media widely questions the challenges faced by AI, such as technological upgrades and the exhaustion of training data, but tech giants like Sam Altman insist that there are no bottlenecks! Many leading AI companies remain optimistic about the industry's prospects and believe that AI will continue to advance through the development of new data sources, increasing model inference capabilities, and applying synthetic data
“OpenAI's new generation large model Orion is not such a big leap”, “Anthropic delays the release of the new Claude model”, “Google's upcoming new version of Gemini falls short of expectations”...
Recently, multiple media outlets have reported that AI companies are facing widespread technological upgrade bottlenecks, with terms like “delay”, “doubt”, and “falling short of expectations” frequently appearing in the reports. As AI becomes increasingly accessible, these AI companies seem to be trapped in an upgrade dilemma.
According to a report by Business Insider on November 27, the progress of AI technology is slowing down. The main challenges facing this field include bottlenecks in large model performance improvement, a shortage of training data, issues with data quality, and obstacles in enhancing reasoning capabilities.
However, several leading companies, including OpenAI and Google, insist that AI has not encountered so-called “barriers” and “bottlenecks.” They remain optimistic about the future of AI and believe that by developing new data sources, increasing model reasoning capabilities, and applying synthetic data, AI models will continue to make progress.
OpenAI's CEO Sam Altman was one of the first to speak out, stating on social media this month: “There is no wall.” The CEOs of Anthropic and NVIDIA also indicated that the progress of AI has not slowed down.
AI Dilemma
Currently, some individuals, including Marc Andreessen, question the significant improvement in AI model performance, noting a trend towards homogenization. For the tech industry, this is a trillion-dollar question, as a diminishing return on existing AI model training methods could impact the investment boom in startups, products, and data centers.
According to Business Insider's analysis, the widespread challenges in the AI field include training data depletion and obstacles to performance improvement.
In the early stages of AI research and development, companies may encounter two main bottlenecks: computing power and training data. First, the ability to obtain specialized chips (such as GPUs) is limited, affecting large model training. Second, the bottleneck of training data is gradually becoming apparent, as publicly available data resources on the internet are becoming increasingly depleted. Research institution Epoch AI predicts that by 2028, the data available for training may be exhausted.
Data quality has also become a significant issue. In the past, researchers could afford to have lower quality requirements during the pre-training phase, but now there is a greater need to focus on data quality, not just quantity.
The enhancement and breakthrough of reasoning capabilities are considered the next key direction for AI development. Ilya Sutskever, former chief scientist at OpenAI, stated to the media this month that the scale expansion of models during the pre-training phase has reached a plateau, and “everyone is looking for the next breakthrough.” At the same time, the cost of upgrading AI is continuously increasing. As the scale of models expands, the costs of computation and data processing have significantly risen. According to the CEO of Anthropic, a complete training process in the future may require an investment of up to $100 billion, which includes the enormous costs of GPUs, energy, and data processing.
Major companies are breaking through barriers
In the face of skepticism, major AI companies have successively proposed their plans to address the bottlenecks in AI development.
Currently, multiple companies are exploring the use of multimodal data and private data to tackle the issue of insufficient public data. Multimodal data involves inputting visual and audio data into AI systems, while private data is obtained through licensing agreements with publishers. At the same time, improving data quality has also become a focus of research, with generating synthetic data (data generated by artificial intelligence) emerging as a possible solution.
In addition, companies like Microsoft and OpenAI are working to empower AI systems with stronger reasoning capabilities, enabling them to conduct deeper analyses when faced with complex problems.
- OpenAI: is collaborating with organizations like Vox Media and Stack Overflow to obtain private data for model training. Additionally, they have launched a new model o1, attempting to improve reasoning capabilities through "thinking."
- NVIDIA: is overcoming supply constraints to ensure the supply of GPUs to support AI model training.
- Google DeepMind: The company's AI lab is adjusting its strategy, no longer solely pursuing the expansion of model scale but focusing on specialization in specific tasks through more efficient methods.
- Microsoft: In the recent Ignite event, CEO Satya Nadella mentioned that they are researching a new "test-time computation" model that allows models to spend more time addressing complex problems, thereby enhancing reasoning capabilities.
- Clarifai and Encord: are exploring the use of multimodal data to break through the public data bottleneck. Multimodal data combines visual and audio information, providing a more diverse data source for AI systems.
- Aindo AI and Hugging Face: are researching synthetic data to improve data quality