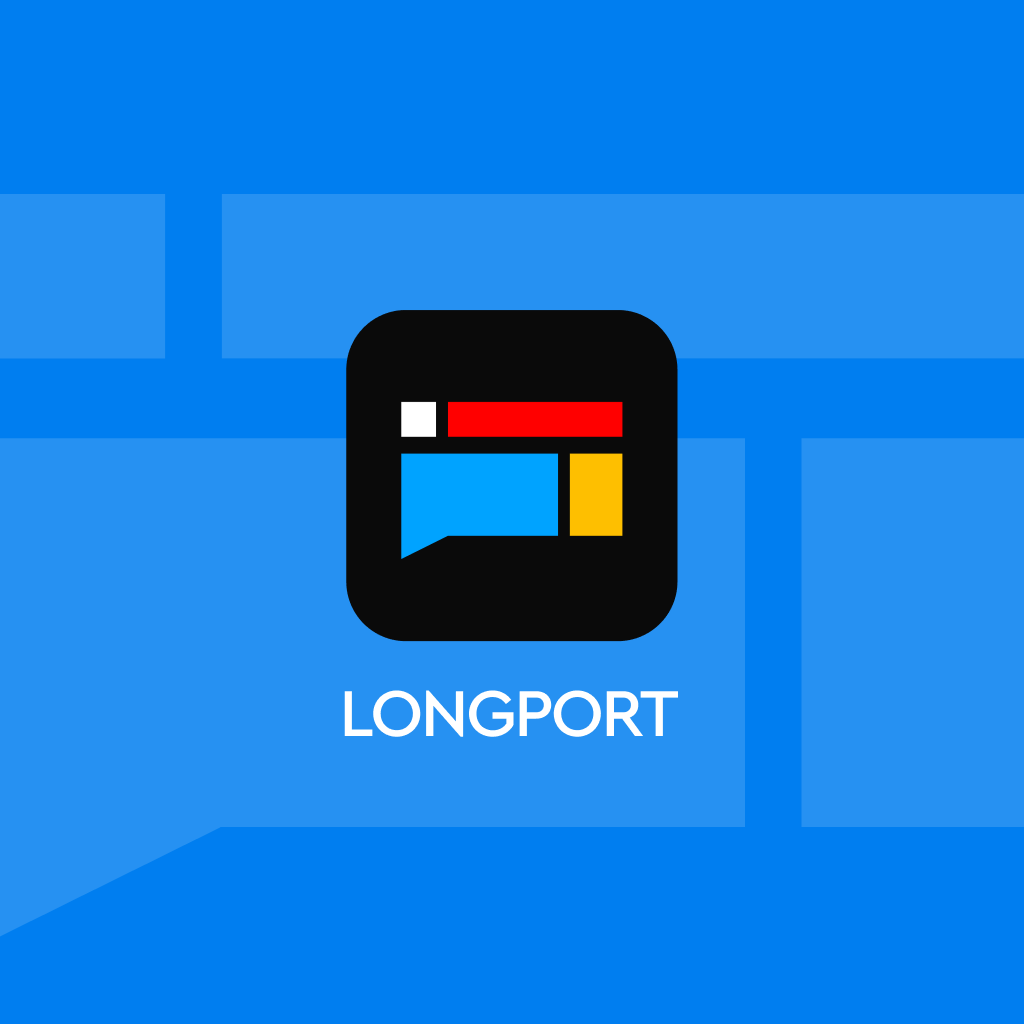
What does o3 mean? The "scaling law" continues in 2025, with costs becoming more expensive and less controllable
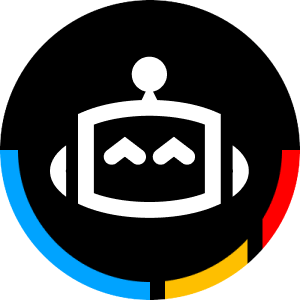
Anthropic co-founder Jack Clark believes that next year, the AI field will combine "testing-time scaling" with traditional pre-training scaling methods to further explore the potential of AI models. However, while the o3 model has renewed confidence in the progress of AI scaling laws, it uses unprecedented computational resources, which means that the cost per answer has increased, and it may also mean that o3 might not become a tool for everyday use
The AI scaling laws have entered their second generation, and of course, costs have as well.
Recently, AI development seems to have entered the "Second Era of Scaling Laws," with some analysts pointing out that the established methods for improving AI models are showing diminishing returns. Currently, a new and promising approach is "test-time scaling," which is the method adopted by OpenAI's o3 model and is also the reason for o3's outstanding performance.
It is important to note that while the o3 model has rekindled belief in the progress of AI scaling laws, it is not without its flaws: o3 utilizes an unprecedented amount of computational resources, which means that the cost per answer has increased, and it also means that o3 cannot become a tool for everyday use.
Specifically, "test-time scaling" means that OpenAI uses more computational resources during the reasoning phase of ChatGPT—the time between when the user presses the generate button and when the AI provides an answer. OpenAI may be using more computational chips to answer user questions, possibly using more powerful reasoning chips, or even running these chips for a longer duration. After all, in some cases, o3 takes 10 to 15 minutes to provide an answer.
Additionally, Anthropic co-founder Jack Clark and other analysts point out that o3's outstanding performance in the ARC-AGI benchmark test marks progress in AI models, but passing this test does not mean that AI models have achieved Artificial General Intelligence (AGI). After all, o3 still fails at some very simple tasks that humans can easily complete—clearly, o3 and "test-time scaling" have not yet resolved the hallucination problem of large language models.
AI's progress in 2025 will be faster than in 2024, and o3 is evidence of this
Clark stated in a blog post on Monday that the o3 model indicates that leveraging the currently powerful foundational models and implementing "test-time scaling" during reasoning can yield significant returns. Clark predicts that the most likely next development is that reinforcement learning (RL) and foundational models will be scaled simultaneously, leading to even more dramatic performance improvements.
"This is big news because it suggests that AI's progress in 2025 should accelerate further compared to 2024."
Clark added that there have been many strange reports recently claiming that "scaling has hit a bottleneck." In response, Clark argued:
"In a narrow sense, this is true because larger models achieve smaller score improvements when tackling challenging benchmarks compared to their predecessors, but in a broader sense, this statement is incorrect, because the technology behind o3 means that scaling is still ongoing... By 2025, we will see a combination of existing methods (large model scaling) and new methods (RL-based 'test-time scaling,' etc.)." Clark also added that next year, the AI field will combine "test-time augmentation" with traditional pre-training augmentation methods to further explore the potential of AI models.
Outstanding o3
Many people view the o3 model released by OpenAI as proof that the AI augmentation process has not "stalled" — o3 performed excellently in benchmark tests, scoring far above all other models in a general capability test called ARC-AGI, with one attempt reaching a score of 88%, while o1's best performance was only 32%. Additionally, o3 achieved a score of 25% on a difficult math test, with no other AI model scoring above 2%.
Noam Brown, a co-creator of the o series models, stated last Friday that OpenAI released the o3 model just three months after announcing the o1 model, and the speed of improvement in AI performance is impressive:
"We have every reason to believe that this trajectory of development will continue."
Expensive o3
Although the o3 model has renewed confidence in the progress of AI augmentation laws, it is not without its flaws: o3 uses an unprecedented amount of computation, which means the cost per answer is higher.
Clark wrote in his blog:
"Perhaps the only point to note is that o3 performs better partly because its operational costs during inference are higher — being able to leverage 'test-time augmentation' means that for certain problems, you can get better answers by increasing computational resources. This is interesting because it makes the cost of running AI systems more unpredictable — previously, you could estimate the cost of running a generative model just by looking at the model itself and the cost of generating a certain output."
Returning to this chart, aside from the extremely high score achieved by o3 on the vertical axis, o3 also stands out on the horizontal axis — the high-scoring version of o3 used over $1,000 in computational resources for each task, while o1 used only about $5 per task, and o1-mini used just a few cents per task.
Francois Chollet, the creator of the ARC-AGI benchmark test, wrote in his blog:
"OpenAI used approximately 170 times more computational resources to generate the 88% score than the efficient version of o3, while the efficient version's score was only 12% lower than the high-scoring version." Chollet continued to add:
"o3 is a system that can adapt to tasks it has never encountered before, and it can be said that its performance in the ARC-AGI field is already close to human levels. Of course, the cost of this generality is very high, and it currently lacks economic viability."
However, it is still too early to discuss specific pricing, as the prices of AI models have dropped significantly over the past year, and OpenAI has not yet announced the actual cost of o3. What is more worth exploring is that the high computational price of o3 shows how much computational power is needed to break through the performance threshold of current leading AI models.
o3 Still Has Limitations
Although o3 performs excellently in various tests, it is indeed not perfect.
Analysts point out that o3 or its successor models will not become "everyday tools" like GPT-4 or Google Search, as these models use too many computational resources and cannot answer everyday small questions, such as "How can the Cleveland Browns have a chance to enter the 2024 playoffs?"
Yes, AI models that use "expanded testing time computation" may only be suitable for more macro questions, such as "How can the Cleveland Browns become Super Bowl champions in 2027?" However, it may only be worth incurring such high computational costs when you are the general manager of the Cleveland Browns making significant decisions.
As Wharton School professor Ethan Mollick pointed out, only financially strong institutions are likely to afford o3, at least in the initial stages.
Currently, OpenAI has released a $200 subscription tier for users to access the high-computation version of o1, but reports indicate that OpenAI is also considering launching a $2,000 subscription tier—after seeing the computational resources used by o3, it is understandable why OpenAI would consider this.
Additionally, while o3's outstanding performance in the ARC-AGI benchmark test marks progress in AI models, passing this test does not mean that the AI model has achieved artificial general intelligence (AGI), as o3 still fails at some very simple tasks that humans can easily complete—clearly, o3 and "testing time expansion" have not yet solved the hallucination problem of large language models.