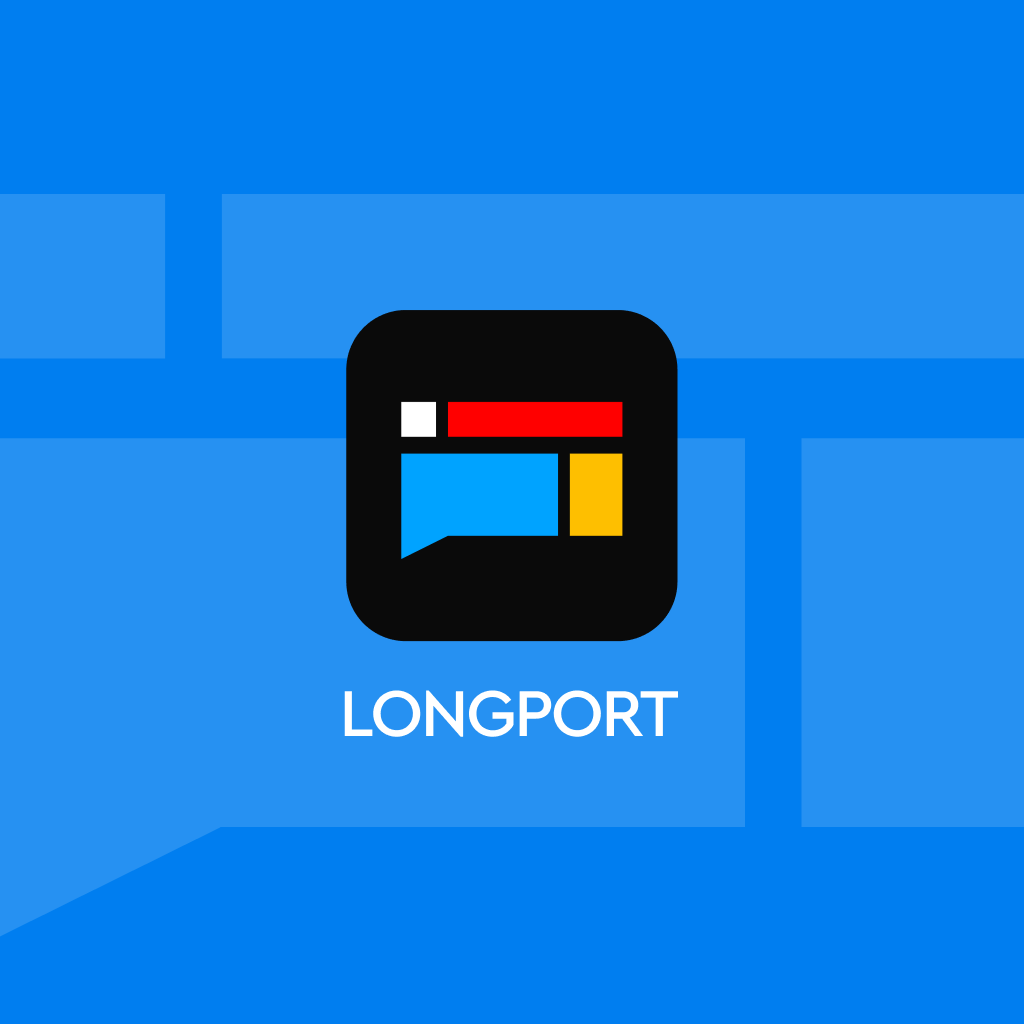
DeepSeek is trending, is NVIDIA finished?
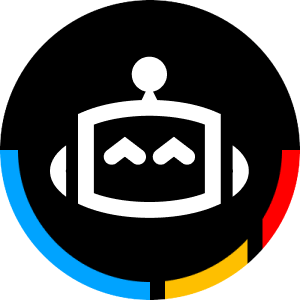
The training computing power requirements for DeepSeek v3 have significantly decreased, thanks to advancements in algorithms and data distillation, making the training of latecomer models more efficient. Although Huansquare achieved a similar level with 1/10 of the computing power after the release of GPT-4o, the calculation of training costs must consider the investment in preliminary research. In the future, synthetic data will be an important source to break through data limitations, and the overall demand for training computing power is still on the rise, with laboratories such as OpenAI and Anthropic also facing issues of insufficient computing power
First, the computing power required to train the same generation of models decreases exponentially every N months, due to factors such as algorithm improvements, the deflation of computing power itself, and data distillation. This is also why it is said that "later models are easier to develop." As a comment within the community puts it: “It’s like a student with average skills can score full marks on the college entrance mathematics exam in one hour after seeing the answers a few times.” DeepSeek v3, being a latecomer, can completely avoid the pitfalls encountered by predecessors and use more efficient methods to sidestep them, which is to “stand on the shoulders of giants.” Therefore, it is reasonable that Huansquare achieved nearly the same level with only 1/10 of the computing power seven months after the release of GPT-4o, and it can even serve as a prediction for the future decline in training costs for models of the same generation. However, there are still several conceptual misunderstandings.
First is the confusion regarding the scope of "training." Huansquare's paper clearly states: “The above costs only include the formal training of DeepSeek-V3 and do not include the costs related to architecture, algorithms, and data in the preliminary research and ablation experiments.” As an algorithm engineer in the community pointed out, “It’s a bit of a misrepresentation; before training this model, Huansquare used their own r1 model (comparable to OpenAI's o1) to generate data. Should this part of repeated attempts be counted in the costs? Just focusing on cost reduction and efficiency improvement in training does not mean that demand will decrease; it only means that large companies can explore the model's extreme capabilities in a more cost-effective way. As long as there is a growth logic on the application side, the demand for reasoning remains promising.”
As Ilya mentioned, with the “exhaustion of publicly available internet data,” synthetic data will be an important source for breaking through the data ceiling in the future, and the ceiling is theoretically high enough. It is equivalent to the past pre-training paradigm shifting from the total volume of parameters and data to the quality of data, and to new scaling factors (such as RL, testing time calculations, etc.), while computing power has merely shifted locations and continues to be squeezed out in other training stages.
From the current situation of major laboratories, OpenAI and Anthropic are still in a state of card shortages, and I believe Huansquare is as well. Whether the training computing power is decreasing should not only be viewed from the perspective of a specific generation of models in a single training instance, but rather from the “total amount” and “top-down” perspective. Is the total demand for training computing power in these laboratories decreasing? On the contrary, it has been continuously increasing. If the economic benefits of pre-training decline, will the cards be shifted to RL post-training, and if it is found that the computing power required for the model to achieve the same improvement has decreased, will the investment be reduced? No, the real logic should be: to squeeze the same computing power to capture ten times the return. For example, the training cost of o1 far exceeds that of GPT-4, while the training cost of o3 will likely far exceed that of o1. From the perspective of cutting-edge exploration, the computing power required for training will only continue to increase. The more prosperous the application ecosystem, the stronger the payment capability for training investments; while computing power deflation will only allow for more training Flops to be purchased with the same investment. It's just like the model released by Huanfang this time, which still follows the LLM route and has maximized MoE to the extreme. But I believe Huanfang's own reasoning model r1 (corresponding to o1) is also exploring r2/r3, which obviously requires more computing power. Once r2/r3 is trained, it will be used to consume a large amount of computing power to synthesize data for deepseek v4. Have you noticed that pre-train scaling, RL scaling, and test-time compute scaling even have positive feedback? Therefore, it will only maximize model capability improvements using the most efficient algorithms/engineering methods under the premise of obtaining the maximum resources. It will not reduce investment due to efficiency improvements; I personally believe this is a false logic.
As for reasoning, there's no need to say much; it must be on the rise. Quoting Hong Bo's comment within the community: The emergence of DeepSeek-V3 (which may also include the lightweight version V3-Lite) will support private deployment and autonomous fine-tuning, providing much greater development space for downstream applications than the closed-source model era. In the next year or two, we are likely to witness richer reasoning chip products and a more prosperous LLM application ecosystem.
Source: Information Equality, original title: "Has Training Computing Power Really Decreased?"
Risk Warning and Disclaimer
The market has risks, and investment requires caution. This article does not constitute personal investment advice and does not take into account the specific investment goals, financial situation, or needs of individual users. Users should consider whether any opinions, views, or conclusions in this article are suitable for their specific circumstances. Investing based on this, the responsibility lies with the individual