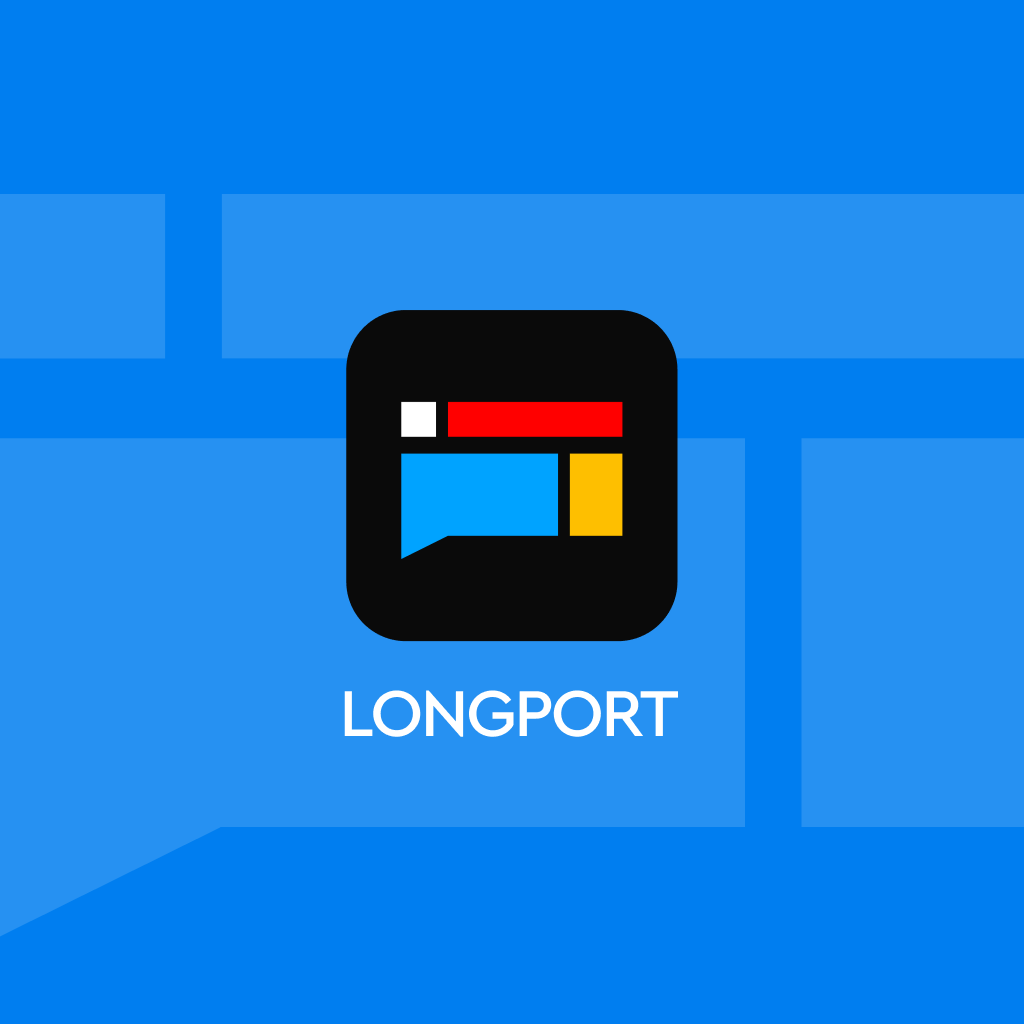
Chen Shi of Fengrui Capital: Next year will be a big year for AI applications, and revenue visibility is expected to improve | Alpha Summit
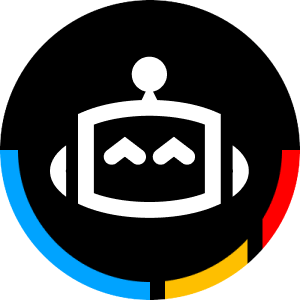
Chen Shi stated that with the support of new models such as o1 and o3 from OpenAI, AI applications are about to usher in new entrepreneurial opportunities. Entrepreneurs in AI applications can prioritize targeting professional users (ToP), learning from and drawing on the experiences of currently successful ToP applications (such as multimodal creative tools and AI dialogue assistants), and striving to develop new AI applications that far exceed the experience of traditional internet applications
On December 21, at the "Alpha Summit" jointly held by Wall Street Insights and the China Europe International Business School, Chen Shi, founder of Peakview Capital, reviewed and forecasted the development of the AI industry, sharing insights on cutting-edge large models and AI applications in the industry.
Here are some highlights from the speech:
Besides AMD and Intel, many major tech companies, model vendors, and startups in the U.S. are developing their own computing chips, hoping to carve out a share of the huge demand for AI large model computing power, especially in the area of inference chips. In terms of applications, the miniaturization and edge deployment of models is a clear trend. Running larger models on terminals or calling cloud-based large models via remote APIs can be slow and cost-inefficient, so this is essentially a trend.
Currently, there are two types of AI mobile applications that are the most profitable. One type is AI+ image / video, which includes multimodal creative tools for creating marketing content such as commercial videos and photo editing, accounting for 53% of the market share. The other type is AI+Chatbot, which includes large model assistants like ChatGPT and AI companionship chat assistants like Character.ai, with a market share of around 30%.
In the AI industry supply chain, large cloud vendors act as risk absorbers, but conversely, they also control the AI commercial ecosystem, holding resources, talent, and a cloud computing market worth hundreds of billions of dollars. Therefore, I believe that the current AI industry supply chain leader is the large tech cloud vendors, not the model vendors.
Currently, pre-trained models like the GPT series respond to questions in a "give the answer at once" manner, lacking the ability to think through steps, backtrack, or iterate. In contrast, models like OpenAI's o1/o3 first "think deeply" upon receiving a question, searching for possible thought chain spaces involved in solving the problem before outputting an answer. This is similar to the complex thinking process of humans, and it is more suitable for complex reasoning, which previous models could not achieve.
Users are increasingly expecting immediate feedback from AI models, insights into personal needs, and tailored personalized answers. This poses significant challenges for model performance and product planning, but once such products are developed, they have the potential to outperform traditional code-based mobile internet products.
Currently, the camp of large model vendors has basically formed, with the 5 companies in the camp being: Google, OpenAI, Anthropic, xAI, and Meta. This means that the infrastructure for AI is already in place, and the subsequent application development will not face significant issues.
The year 2025 is expected to be a big year for AI applications, and the balance sheets of the supply chain may gradually improve, thanks to the accumulation of early investments. Additionally, the visibility of client-side revenues will also increase The following is the transcript of the speech:
Thank you everyone, and thank you to the Alpha Summit for the invitation. I also shared on the topic of AI last year, mentioning some topics such as generative AI's multimodality, complex reasoning, embodied intelligence, and the self-iteration of models. Over the past year, I have observed that AI technology has developed rapidly, and practical products have emerged. In 2024, Peakview Capital has invested in nearly 30 projects, most of which are related to AI, such as applications, hardware, chips, embodied intelligence, and AI-enabled scientific research. Therefore, today I would like to share with you our investment practices and industry thoughts for 2024.
My speech is divided into three parts: the first part is the overall situation of the industry, including the supply chain; the second part is an in-depth explanation of models and applications; the third part is an outlook on the AI industry in 2025 and its future trends.
Let's quickly review the development of AI in 2024, which can be divided into two parts. The upper part is about the original driving forces of the AI industry, such as large models and their infrastructure, while the lower part focuses on the application side of AI.
In terms of large models, we see three leading players emerging globally in the closed-source foundational model field: Anthropic's Claude 3.5, Google's Gemini 1.5, and OpenAI's GPT-4o. These three models are on par with each other, reaching the state-of-the-art (SOTA) level in the industry.
However, in the second half of this year, many people may wonder if the AI industry is facing a downturn. They have observed that the scale law seems to no longer be effective, and OpenAI has not released particularly good new models for a while. Of course, OpenAI later lived up to expectations by releasing the o1 model in September and the o3 model in December, which is almost the hope of our entire AI industry.
Why do I say this? Once closed-source models reach the level of GPT-4, the existing pre-training methods actually find it difficult to achieve significant improvements, unless their infrastructure is increased several times or even tenfold. It is said that the computing power required to train the next generation of models is ten times that of the current models. The new o1 and o3 models represent a new training paradigm that can greatly enhance complex reasoning and self-iteration capabilities, which I will briefly introduce later.
In terms of multimodality, there has also been significant progress in 2024, from OpenAI's video generation model Sora at the beginning of the year to Google's Veo2 model at the end of the year, as well as the release of GPT-4o in May (where "o" stands for omni, meaning all-encompassing). Its input supports multimodality, and its output also supports multimodality, especially in real-time voice conversations, which is quite impressive.
In the open-source area, I believe Meta is a very smart company. With the top three in the closed-source field being difficult to surpass, Meta's choice to adopt an open-source ecological niche is very wise. A large number of developers, industry applications, and industry models will use Meta's Llama3 open-source model because open-source models provide them with more space for secondary development, model fine-tuning, and capability expansion. Of course, we are pleased to see that competitive open-source models such as Qwen and DeepSeek have also been launched domestically When domestic companies are researching and developing industry or enterprise models and applications, they generally prefer domestic open-source models.
In terms of infrastructure, I feel that the changes are not significant; NVIDIA still holds a dominant position. However, we see that, apart from AMD and Intel, many American tech giants, model vendors, and startups are developing their own computing chips, hoping to carve out a share of the huge AI computing market from NVIDIA, especially in the area of inference chips. China also has many such chip companies, including those invested by us at Fengrui Investment, actively engaged in research and production in this area.
From the perspective of user-side applications, the miniaturization and edge deployment of models is a clear trend, because running larger models on terminals or calling large cloud models remotely is slow and costly. The Apple Intelligence feature released by Apple at the end of October uses a self-developed edge model with 3 billion parameters, which can be deployed and run on current phones, iPads, and laptops. However, recent media reports indicate that Apple Intelligence occasionally makes mistakes, including hallucinations and incorrect news headlines, which may also be related to the smaller model parameters, indicating room for further improvement.
In addition, the technology of AI and large models is expected to break new ground in 2024. The first is basic science; this year, the Nobel Prizes in Physics and Chemistry were awarded to scientists and engineers in the AI field. The second is in autonomous driving, where Tesla's FSD algorithm, as well as domestic new energy manufacturers or smart driving companies, have made significant progress in algorithms and models, all relying on foundational models and AI technology. The third area empowered by AI is embodied intelligence, which is also a hot track in the AI direction; this year, we at Fengrui have invested in several companies in this field.
The last point is the implementation of AI applications. In my presentation at the Alpha Summit in 2023, I also mentioned that AI applications need to start developing vigorously, but unfortunately, the development of AI applications in 2024 is not as expected. I believe that next year AI applications will yield relatively good results, and I will provide further analysis on the specifics later.
What is the current state of the AI industry? Recently, Sequoia Capital in the U.S. stated, "The foundation of AI has been firmly established." This means that the global camp of the five major model vendors has basically formed. There may be some minor changes in the future, such as whether Apple will enter the market, but it currently appears that these five companies are in a leading position, including Google, OpenAI, Anthropic, xAI, and Meta. Each of these five has its strengths, and with new models like o1 and o3 continuing to develop, the overall model capabilities have formed a solid foundation to support the implementation of AI applications.
Now let's take a look at the input-output of the AI industry, which is also the most criticized aspect of the AI industry; specifically, there is huge investment but meager output. The four leading tech giants—Meta, Google, Microsoft, and Amazon—had capital expenditures of $52.9 billion in the second quarter of 2024, with most of the investment directed towards AI In addition, AI startups invested in by VC and tech giants have also reached new highs in investment amounts. The number of AI data centers operated by the aforementioned four giants has expanded from 500 in 2020 to nearly 1,000 by 2024, and these data centers are all high-level, GPU-centric, large-scale computing power-intensive data centers.
Leading AI computing power chip provider NVIDIA's revenue for Q2 of fiscal year 2025 reached USD 30 billion, primarily from investments in computing power in the AI industry, along with significant investments in talent.
The industry believes that compared to the various investments mentioned above, the output of the AI industry needs to reach USD 600 billion to achieve a reasonable level of input-output balance. However, the actual output of the AI industry today is at the level of several billion dollars, with precise figures being difficult to quantify, but it is estimated to be around 30 billion dollars, still a significant gap from 600 billion dollars.
Another statistic shows that currently, there are fewer than 100 AI startups globally with annual recurring revenues reaching USD 10 million, indicating relatively low overall revenue. Among the revenue data of several leading companies I listed, OpenAI is likely the highest earner, claiming to achieve USD 3.7 billion in revenue by 2024, along with others including Microsoft's GitHub Copilot and Anthropic. Additionally, according to a chart released by Sensor Tower, the revenue from AI applications on mobile devices is approximately USD 3.3 billion in 2024, with two types of AI mobile applications being the most profitable. One type is AI+ image / video, which includes multimodal creative tools such as video and image creation and editing, accounting for 53% of the market share. The other type is AI+Chatbot, including large model assistants like ChatGPT and AI companionship chat assistants like Character.ai, which holds a market share of 29%, while other types of applications have relatively lower revenues. From a national market perspective, Europe and the United States account for about two-thirds, so going overseas is also a major demand, and most of the AI application companies we invest in are focused on international markets.
Earlier, I mentioned the mismatch between investment and output in the AI industry, so who bears the risks in this industry? Sequoia Capital in the U.S. pointed out that the current AI supply chain is in a "fragile balance" state. Looking at the layers, the lowest tier of foundries is profitable, such as TSMC; the next tier of semiconductor manufacturers is also profitable, such as NVIDIA; the cloud providers in the middle are operating at a loss; the model providers above them are also likely operating at a loss, with their investments coming from cloud providers or venture capital; at the top are the customer layers, which are the revenue from application layers like ChatGPT and GitHub Copilot. So where is the risk? The risk mainly lies with the large cloud providers. Large cloud providers invest significant capital expenditures and act as risk absorbers. From another perspective, I believe that large cloud providers actually control the commercial ecosystem of AI, holding resources and talent, as well as a cloud computing market worth hundreds of billions of dollars. The chain master of the AI supply chain is the large cloud providers, and this situation applies in both China and the United States So the industry needs to think about how to approach model entrepreneurship. Can large model startups develop independently?
The leading language model camp in the United States has basically converged, mainly with tech giants pairing up with top models, as mentioned earlier with these five model vendors. Potential challengers, such as Character.ai, Inflection, and ADEPT, have also been acquired by these major companies, further validating the control of large companies over the AI supply chain. The language model camp in China is also rapidly converging, with major cloud vendors like Alibaba, ByteDance, Tencent, and Baidu not only developing their own models but also actively investing in model startups. Among startups, China's "six little tigers" of models have taken the lead, but they have also faced significant pressure this year. There are a few other competitive followers. Returning to our earlier discussion, I believe that in the future, Chinese cloud vendors will also control the AI supply chain, and independent large models will still be quite difficult. Of course, there is a special factor in China, which is the national team. I believe there will be a national team emerging, or startups supported by the national team.
There is a chart from EpochAI showing that the time gap between open-source models and closed-source models is 5-22 months— for example, after the release of GPT-4, Llama 3.1 took about 16 months to catch up to the level of GPT-4 at that time. You can consider open-source as representing the industry benchmark, so this is a brutal survival battle, that is, our three major closed-source models have at most 22 months to develop users and capture the market, otherwise the industry will catch up.
Therefore, if the open-source strategies of models like Llama and Qwen remain unchanged, both domestic and foreign closed-source foundational models will face significant competitive pressure. The positioning of open-source models is quite good, capturing developers in the industry and enterprises, including some large companies, vigorously forming a cooperative ecosystem, and becoming good followers of closed-source models. Of course, domestic closed-source model vendors are in a more difficult position, whether they are large companies or startups, because while being vigilant against the pursuit of open-source models at home and abroad, they also need to invest heavily to continuously catch up with the world's leading models, with a shorter window period. It is said that the training cost of the GPT-4 foundational model requires 100 million USD, while the training cost for GPT-5 or the next generation foundational model will reach 1 billion USD. Even for China's large companies, coming up with 1 billion USD to train a model will face challenges. Of course, there are also uncertain factors in the future, such as whether Meta will still be willing to open-source its next-generation model if it spends 1 billion USD on training. This is also an unknown factor, so there are actually many uncertainties in this industry.
Having discussed models, let's talk about applications. Why do we feel that AI applications are not meeting expectations? In addition to the low revenue mentioned earlier, there are two other parts. On one hand, the top two AI applications, which have the largest user base, are ChatGPT and Character.ai. ChatGPT's traffic, after experiencing a steep rise in the early stages, suddenly leveled off in the summer of 2023, and it is expected to show an upward trend again in the summer of 2024, likely coinciding with the release of GPT-4o. The subsequent data has not yet been updated and remains to be observed Character.ai's traffic began to decline in the second half of 2023 and has not shown any signs of recovery. So in terms of user growth, leading companies are facing some challenges. On the other hand, comparing the current leading AI applications with the leading applications of the internet/mobile internet era, it is found that the user engagement metrics of the former are far inferior to those of the latter, which is also a less than ideal situation.
Of course, this is just the current state, and there are individual reasons, but as an emerging industry, if leading enterprises cannot continue to develop rapidly, the entire industry will face some pressure. I guess the main issue may still be the insufficient model capabilities, which makes our AI applications unable to differentiate themselves from traditional applications. If we can have new models that unlock more powerful capabilities, it may be possible to create applications that far exceed the current experience, and perhaps there will be an opportunity to bypass the growth trap.
According to statistics from American a16z, among the top 50 applications and apps in global user access rankings, 52% are creative tools, such as image and video editing tools, which is clearly the largest category. The second largest category is AI + Chatbot, such as large model assistants like ChatGPT and AI companionship chat assistants like Character.ai. Other category changes are minimal, so in 2024 the leading AI applications have not produced significant changes in categories.
After an overall overview of the industry, let's delve into the progress of models. We will first focus on OpenAI's o1, which represents a new paradigm of models that enhances complex reasoning capabilities through a chain of thought. Reasoning refers to the use of rational thinking and cognitive processes to infer new knowledge from existing knowledge. This is a very powerful ability of humans, including common sense reasoning, mathematical reasoning, symbolic reasoning, causal reasoning, and so on.
So what is a chain of thought? A chain of thought refers to a series of intermediate reasoning steps. When a person thinks about a complex problem, there is a chain or even a tree or diagram of thought in their mind, collectively referred to as a chain of thought. During the thinking process, if one finds that a step does not work, they can revert to the previous step for further exploration. However, our current pre-trained models, such as GPT-4, do not have the ability to revert; their working mode is like "word relay," producing one token at a time. If you take ten steps and find that there was an error in a previous token, there is no way to go back; you can only make corrections afterward, but this may not be fixable. This is just an inaccurate simple analogy, but it helps us understand why the current foundational models are not as capable as humans in complex reasoning and other aspects.
Today, o1 has relatively strong complex reasoning capabilities. If we ask a question, it will first think rather than immediately provide an answer, conducting a search or traversal of the chain of thought during the thinking process. After the traversal is complete, it will begin to state the conclusion. In my demonstration, after it provides the conclusion, there is also a summary called the chain of thought steps, where it summarizes nine thinking steps. However, it actually has an implicit complex chain of thought; according to OpenAI's paper, taking the nine thinking steps as an example, the implicit thought process consists of about 600 lines of text, each line resembling a form of self-talk It is "I did this, I guess what it might be like, and found it wasn't me, then I reverted back to some previous point." This process is very similar to human thinking processes and is closer to complex reasoning abilities.
What are the assessment criteria for complex reasoning? One is AIME, the American Mathematics Invitational Examination; another is Codeforces, a very difficult programming competition; and there is also GPQA, a doctoral-level scientific question. The responses from o1 clearly surpass those of previous models, with some assessments exceeding human experts. Meanwhile, o3 has significantly improved its capabilities based on o1. OpenAI released five levels of capability for its foundational models in July this year, believing that the current o1 or o3 has reached the second level, known as "reasoner," which indicates a strong reasoning ability and the capacity to perform basic tasks, equivalent to a human with a doctoral degree who has no tools.
Simply put, I believe the learning of AI models can be divided into two steps. The first step is the pre-training of GPT-type models, which uses a large amount of human text data for training, akin to "imitation learning," mimicking how humans choose words, construct sentences, and think. However, at the current stage, the internet data available for imitation has nearly been exhausted. At this point, models like o1 and o3 begin to choose " reinforcement learning ," generating data through active exploration and self-play, training and reasoning the model based on methods like thinking chains, and achieving "test time computation."
To make an analogy, it's like a martial arts master who, when young, learns well from a mentor, but after surpassing the mentor, what if they can't find a better teacher? They can only learn by themselves and explore forward.
The previous AI models for playing Go (AlphaGo and AlphaZero) were similar. AlphaGo initially trained using human game records, but after reaching a certain level, its strength plateaued. Then AlphaZero emerged, completely discarding human game records and relying on self-play to train itself, achieving a higher realm. This is also one of the cases of the gradual progression from imitation learning to reinforcement learning. Therefore, many concepts in technology are actually interconnected.
The reinforcement learning difficulty for AI models like o1 or o3 is greater than that of Go, because Go has simple assessment criteria (such as win or lose), while AI models often do not receive accurate assessment signals in most cases. However, a clever point is that this time they brought in a helper, namely the pre-trained models like GPT that were trained through imitation learning, which can help generate better assessment signals, thereby assisting the reinforcement learning training of o1 or o3.
Recently, there has been a discussion about Terence Tao and Mark Chen. Terence Tao is a renowned mathematician and Fields Medal winner, while Mark Chen is the Vice President of R&D at OpenAI. Terence Tao said that AI is not good at finding the right questions, but it can handle very narrow specific parts within a larger project, similar to generating reasoning with sparse data, so this ability is powerful and comes from intuition and experience. Mark Chen stated that we are currently working on test time computation, which he believes can surpass current reasoning capabilities and achieve human-like intuitive reasoning under sparse data conditions I think both sides have their merits. At that time, Terence Tao was only using GPT-4, utilizing AI for data research. The pre-trained model of GPT-4 indeed only had that capability. However, Mark Chen also made a valid point, as new models like o3 do have the ability to reach that level.
Since o3 was released today (December 21st, Beijing time) at 4 AM, I specifically added a slide to the PPT. One of the three main capabilities of the model is coding and programming, with dataset evaluation scores improved to over 70% compared to o1. We invested in a company that develops AI coding applications, and the founder told me that if the model's evaluation score on high-difficulty programming test datasets exceeds 70%, it is basically considered usable, because we can think of other ways to reduce the difficulty of practical applications, allowing the 70% model capability to approach 100% application capability. Therefore, a model with over 70% capability is generally sufficient. Additionally, o3's scores in the American mathematics competitions and scientific questions are also significantly higher than those of o1.
I would also like to point out that according to OpenAI's official statement, o1 is a large reasoning model trained as a language model using reinforcement learning, while o3 merely further expands the scale of reinforcement learning. However, achieving such a significant improvement in o3 relative to o1 in just three months is quite surprising. This may only be the first step, and there should be further room for improvement and optimization in the future. Of course, the operational cost of o3 is very high; according to unofficial estimates, the cost of o3 answering a question at the highest configuration can reach $2,500. But I believe that the cost issue can be gradually resolved in the future.
Last year, I also mentioned multimodality here. At that time, the industry believed that video would achieve breakthroughs in 2024, and this year, there indeed have been breakthroughs. The standard for breakthroughs is that we see some companies starting to use these video generation tools to produce original materials for advertisements or film and television works. Multimodality is actually just a human concept. From the perspective of AI models, various modalities are vectors in a high-dimensional space in its "mind." For example, the vector of the GPT-3.5 model is 12,288 dimensions, which was later reduced and optimized. Therefore, whether it is text, images, or video, they are all vectors for AI models. Vectors can be calculated with each other. For instance, when we previously talked about the vector of "king," subtracting a "man" vector and adding a "woman" vector gives us a "queen" vector. The training and reasoning of AI models essentially involve similar vector calculations. However, since AI models need to communicate with humans, they still need to recognize our multimodal data and also need to output multimodal data, which requires a process of "deconstruction" and "reconstruction," relying on certain algorithms. The algorithms we see today, including the well-known diffusion models and neural radiance fields (NeRF), are all very interesting algorithms.
Taking AI drawing as an example, a typical human artist would start with a blank sheet of paper, roughly outline the image, gradually add details, then color it, and finally make small adjustments, producing a portrait step by step. However, AI drawing does not follow such a process Taking the Diffusion Model as an example, it first generates an original image (which is actually a randomly generated noise image, specifically an isotropic Gaussian distribution noise image), which is the image in the upper left corner of the PPT. Then, guided by the prompt, the AI model generates a denoised image (which is also a noise image) each time, removing this denoised image from the original image. This operation loops, and after dozens or hundreds of steps of denoising, it produces the portrait of a lady wearing a hat. This operation is very counterintuitive to us humans; our intuition does not suggest that this is how images can be created, but AI understands and creates images this way, which may even be more efficient than humans. These algorithms are quite complex, and there is no need for everyone to understand them in detail, but they are indeed very magical.
Today's multimodal still takes text as the main modality, as other modalities are translated or mapped through the text modality. This “translation” principle has a simple analogy: if AI sees an image, it will first perform “image writing,” writing a long essay to describe the image, and then map the text of this essay as a whole into a high-dimensional space of language, forming a high-dimensional vector, which is the vector of this image. Therefore, it relies on text as a carrier to map into high-dimensional space.
The concept of modality can be extended, not just limited to the current categories of text, images, and videos. For example, the three-dimensional structures of proteins generated by AlphaFold and podcasts in the form of two-person dialogues can also be considered a type of modality. One of the companies we invested in at Peakview Capital is called Top view.AI, which aims to create commercial videos for merchants on TikTok or Instagram, but it can complete most of the work with little human intervention. We only need to provide the link to the product detail page, and it can automatically scrape text, images, and videos, integrate them with specified digital personas, and then automatically carry out a series of tasks such as script creation, voiceover, music, and video editing and synthesis, ultimately completing the video.
This year, a term has become particularly popular, called “world model.” What exactly is it? First, let’s talk about why we need a world model. I just mentioned that text is the main modality, and other modalities are mapped into this high-dimensional space through text, but text is difficult to accurately express the physical world. For example, complex spatial relationships are hard to express in writing, and physical properties, such as a cup breaking when it falls, are also difficult to describe. Therefore, people think that perhaps we should create another model that inherently possesses some visual capabilities, which we call perception. For example, when I stand on the podium and look forward, I can quickly perceive what the scene of the Alpha Summit at the China Europe International Business School looks like, having an overall perception without needing to map other modalities through text. Moreover, after perceiving, I can also predict, and after predicting, I can interact with the physical world. These belong to the basic concept of a “world model.” In summary, large language models form a text-based “world model,” and text is an abstraction that has loss, so we need to create a “visual” world model Yann LeCun's proposed "world model" and Fei-Fei Li's "spatial intelligence" both contain similar concepts.
Gary Bradski, a well-known AI expert known as the father of OpenCV, proposed a "WHAT-WHERE-WHY" framework that can be used to simply explain what a "world model" is. "What" refers to what I can see at a glance today, who is present, what items are there, and what events are happening; "Where" refers to the location, as well as the spatial relationships between them; "Why" refers to the causal relationships or purposes behind the events. For example, in today's AI sharing, the audience consists of leaders in the financial industry who want to understand the development of the AI industry, which is an instance of cause and effect. This model is relatively simple and helps us understand the basic concept of a "world model."
Having discussed the algorithms of the model, let's talk about computing power. The cluster built by Musk, with 100,000 cards, is one of the largest clusters in the world. Currently, other companies are catching up and facing significant competitive pressure. In numerical terms, the four giants' capital expenditures in 2024 will exceed $200 billion, most of which may be invested in data center construction. It is said that the computing power needed to train the next generation of models requires ten times more, and some say that the importance of physical construction of data centers in the next phase will surpass scientific discoveries.
Next, let's discuss data. It is well known that algorithms, computing power, and data are generally regarded as the three major production materials for models. When building a large model in the AI field, most of the data has already been used in the pre-training phase, and the remaining human data is relatively scarce, necessitating a large amount of frontier data for training. Currently, the capability boundary of pre-trained models is data; where data cannot reach, the model cannot imitate. Therefore, it is necessary to construct some data along its capability boundary to help the model generate corresponding capabilities. Thus, the importance of frontier data is highlighted. What is currently lacking are high-quality data such as complex reasoning, professional knowledge, and human thinking patterns.
However, we still have a path, which is the so-called algorithmically synthesized data, including the reinforcement learning and self-play mentioned today. These are new methods, but conversely, reinforcement learning also requires new data to train its capabilities, so these three are very coupled. We have invested in a company that does data engineering, combining human and machine efforts to label data, and actively using algorithms to synthesize data. This company is currently also actively expanding overseas. The leading company in this field is called Scale AI, which has strong profitability and high valuation.
Now, let's talk about AI applications. I believe AI applications are different from traditional internet applications. In the past, we generally divided applications into two categories: one called ToB and the other called ToC. However, I believe that today in the AI industry, there should be a new category called ToP ( Prosumer , professional users), which currently shows excellent performance in user growth and commercialization. Prosumer includes content creators, which is the creator economy, with an estimated 100 million practitioners. It also includes some professional practitioners, technical experts, deep users, etc., all of whom are the super individuals of the future People in this category have clear needs, love to learn, and can actively learn to master a powerful but not easy-to-use AI tool. I believe these professional users are currently the most ideal users and payers of AI applications. Today, ChatGPT is often considered ToC, but I think it is ToP, because to be honest, there are very few people around me who can truly use AI tools like ChatGPT, Doubao, and Kimi effectively. Recently, while writing an article, I intensively used ChatGPT in various aspects such as organizing thoughts, constructing frameworks, forming drafts, and text polishing, and I felt a significant improvement in both writing efficiency and quality. This process allowed me to deeply experience the value of such AI applications for professional users.
This is just one case, illustrating that when we truly want to use AI as a deep productivity tool, we will first face a steep learning curve, and not everyone can learn it. After mastering it, we also need to tolerate its mistakes, because despite AI's power, it can easily make errors and generate hallucinations, so we must have the ability to judge and not accept blindly. There are currently not many people with such abilities, but I believe everyone here can be such professional users, provided they widely try and deeply use AI tools.
I also want to encourage AI application entrepreneurs to first target the ToP market, seeking professional users from various industries, providing them with a powerful tool that offers a significantly better experience than traditional internet applications. Occasional instability and errors are acceptable. These tools should start from ToP, and later there may be opportunities to extend to ToB or ToC. The multimodal creative tools we mentioned earlier mostly belong to ToP, and ChatGPT is essentially also ToP. Currently, ToP applications are clearly dominant, with good user growth and strong revenue capabilities.
The second is ToB, providing services to enterprises. Because human workflows are very complex, and with the difficulty of human-machine collaboration, it is not easy for AI applications to penetrate. Therefore, I think it should first enter through some independent business modules or standard skill modules.
The third is ToC. For ToC, it feels like the disruptive moment has not yet arrived, and I think the core reason is that the model's capabilities are still insufficient. For example, we have previously seen some projects where AI writes promotional articles on social media like Xiaohongshu to earn money, which can generate some income; however, we later found that the articles it wrote did not effectively increase followers, hindering the further development of such applications. Why? I believe that today's language models can produce above-average content, but to create articles that attract followers, the pre-trained models' capabilities are still inadequate and may require significant human involvement and guidance. Will models like o1 and o3 improve the situation? It remains unknown. Currently, many ToC AI applications are similar to the aforementioned cases, where the functionality is good, but the competitive advantage over traditional software is not significant Entering the final part—prospects and challenges. Regarding challenges, a significant issue is the slow product rollout and the long technology application cycle. The core reason may be that everyone realizes that AI must compete with traditional mobile internet, and the product experience cannot be compromised; initial losses can be tolerated, and costs can be gradually reduced later. However, due to insufficient model capabilities, product quality is difficult to reach a score of 80 or 90; it may only achieve a score of 60 or even fail.
Another point is that users are increasingly hoping AI to become a thoughtful assistant. When I ask a question, AI should accurately determine my intent and directly provide the feedback I need, rather than giving me a bunch of search results or requiring multiple interactions. Future AI applications must serve users for a long time, have a deep understanding of user habits (“context”), and possess long-term memory. When users ask a question, AI should know the underlying needs behind the user's question, thus providing accurate answers directly, even offering responses that users themselves may not have considered. This is what AI applications in the era should look like. If such products are developed, I believe they can definitely outperform existing traditional applications based on mobile internet. However, this places high demands on the model and puts significant pressure on product design, construction, and planning.
Regarding industry expectations for 2025, there are several points. First, models will gradually mature (especially with the support of new models like o1 and o3), and AI applications will achieve phased results. I believe 2025 may become a big year for AI applications, and the balance sheets of AI supply chains may gradually be repaired .
Second, regarding model optimization, such as the advancement of integrating “world models” with the physical world, I believe this will greatly help both autonomous driving and intelligent robotics. Third, multimodal integration can be further advanced. Fourth, the interpretability and safety of models; we call it interpretability because it is a black box, and you do not know what it is thinking. AI models represent high-level intelligence, and their capabilities will surpass humans in the future. We need to understand what they are thinking, but this is an extremely challenging task, and there are currently no mature methods. However, this is what we desire; otherwise, it is really difficult to control a model with such strong capabilities that does not listen to you.
Human labor is divided into physical labor and mental labor, with mental labor centered on knowledge, intelligence, and creativity. However, today, I believe AI is deconstructing human labor, and in the future, AI will also possess such labor capabilities, even surpassing humans. AI has one advantage over humans: it is very difficult for humans to cultivate a top scientist like Einstein, but once AI trains a top scientist, it can quickly replicate that capability in bulk. Therefore, the mental labor capabilities that humans take pride in may be possessed by AI in the future, and after large-scale replication, they will ultimately be provided at low cost in a software form. If embodied intelligent robots are added, physical labor may also be widely replaced So, the future of labor may become software-based, just like plug-and-play tools to acquire. Of course, I don't think everyone needs to be overly anxious; this is still a distant matter, and we humans will find ways to coexist with AI. Returning to the present, I believe the most important thing is that all of us here should use AI tools more, understand their capabilities, and recognize their shortcomings. In this process, we can also have some new thoughts and progress, which will greatly promote our own careers, work, and lives. I hope everyone will take the opportunity to seriously use current AI and equip themselves with AI tools to become the "super individuals" of the future. Thank you all!
The Alpha Summit is proudly presented by the all-new Lincoln Aviator
Set off now and navigate the 2025 global investment journey with ease