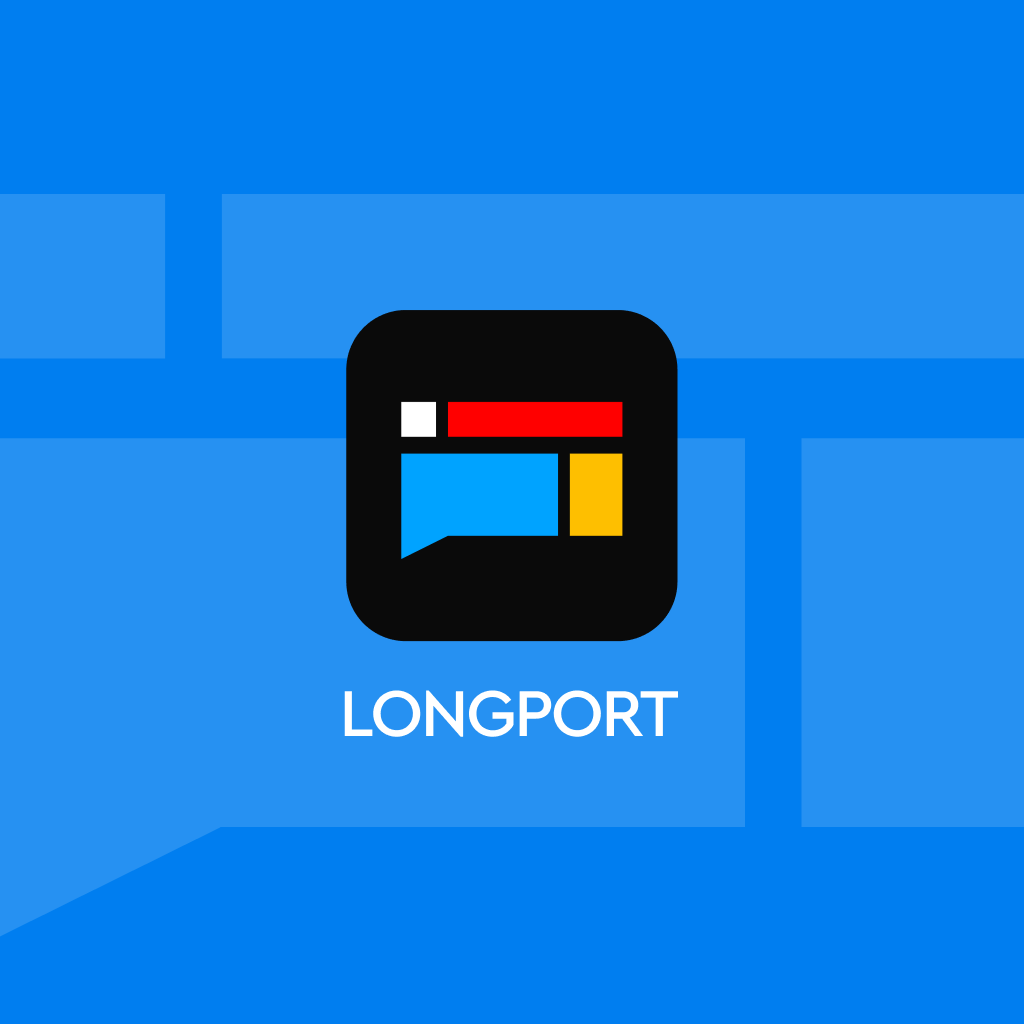


Translation: The ultimate question: Can FSD truly support a $1.5 trillion Tesla?
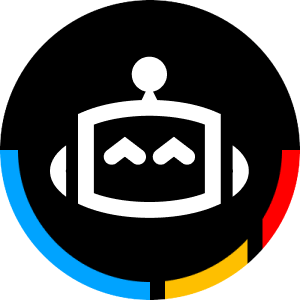
In the previous article "Tesla FSD: Can the Starry Sea Withstand the Test of Reality?" regarding autonomous driving research, Dolphin mentioned that the current commercialization path of FSD has actually been implemented to varying degrees domestically. However, whether it is selling directly to consumers (B2C) or providing services to businesses (B2B), commercialization still faces numerous challenges.
In this study, Dolphin will attempt to answer the following questions that investors are concerned about:
What is the core point for the fully autonomous ride-hailing model to be viable?
What advantages does Tesla have in Robotaxi compared to Loquat Fast Run?
After the Robotaxi business matures, how much improvement can the UE model achieve?
How much valuation can the Robotaxi business contribute to Tesla?
Where are the real opportunities after L4 autonomous driving matures? Is the current valuation of $Tesla(TSLA.US) overvalued?
Which players can still enjoy the opportunities as autonomous driving gradually matures?
1. What is the core point for the Robotaxi model to be viable?
To analyze whether a business model can be viable, we can first look at 1. the economic feasibility (in terms of the UE model) of achieving breakeven for fully autonomous ride-hailing, and what the main limiting factors are; and 2. whether it can be expanded and rolled out on a large scale nationwide;
Since Loquat Fast Run has already started commercial operations in Wuhan, we will first analyze the UE model of Loquat Fast Run in Wuhan to see what the core factors are for the commercialization of autonomous ride-hailing to be viable.
We will compare the UE model of Loquat Fast Run in Q1 2024 with traditional ride-hailing to identify the main factors affecting the breakeven point of Loquat Fast Run and the subsequent improvement space:
First, let's look at the revenue side:
① Daily total revenue: The daily total revenue per vehicle for Loquat Fast Run in Q1 2024 is only 15% of that of traditional ride-hailing.
In the first quarter of 2024, the daily total revenue per vehicle for Loquat Fast Run was only 15% of that of traditional ride-hailing. Since daily total revenue = daily order volume * average kilometers per order * actual price per kilometer after discount, we will break down these core factors of revenue to compare with ride-hailing:In the era of ride-hailing, the ride-hailing capacity (supply side) is nearing saturation, and the average daily order volume is more significantly influenced by demand-side factors. In a homogeneous competitive environment, price incentives usually lead to an increase in daily order volume (exchanging price for volume). As a form of short-distance urban transportation, the average kilometers driven per order remain relatively stable.
However, comparing the key driving factors of revenue between 1Q24's Luobo Kuai Pao and ride-hailing, Dolphin Jun identified two obvious issues:
The average kilometers per order for Luobo Kuai Pao is significantly lower than that of ride-hailing, at only about 50% of ride-hailing's average;
The actual unit price per kilometer for Luobo Kuai Pao is also lower, at only about 40% of ride-hailing's price.
Typically, during the introduction phase of a new transportation platform, the platform offers substantial subsidies to cultivate user habits, with passenger subsidies accounting for as much as 20%-30% of GTV. However, during the introduction phase in 1Q24, Luobo Kuai Pao's subsidies to passengers accounted for 70% of each order's GTV, and the excessively high subsidy discount led to a significantly low actual unit price.
At the same time, Luobo Kuai Pao's "exchanging price for volume" advantage is not obvious, with the average daily order volume per vehicle reaching only about 75% of ride-hailing's volume.
However, since the product offered by Luobo Kuai Pao has not fundamentally changed compared to ride-hailing, both being point-to-point passenger services, Dolphin Jun believes the issue lies in the "defects" of Luobo Kuai Pao's product side.
First, considering the core factors for users when hailing a ride: 1. Efficiency and convenience of hailing a ride; 2. Safety (from the user's perception); 3. Comfort of passenger experience; these form the core triangle of ride-hailing service products. When the differentiation of the ride-hailing service products is not significant, pricing becomes the core competitive factor.
However, from the perspective of the ride-hailing service products currently offered by Luobo Kuai Pao, it does not hold an advantage in any of the core triangle factors identified by Dolphin Jun, and there are even significant "bugs" in efficiency and convenience:
Low success rate of hailing a ride, long waiting times for users, slow travel times (order completion times are nearly twice that of human-driven taxis), and the requirement to get on and off at designated stops (similar to buses), which undermines the efficiency and convenience that distinguish ride-hailing services from public transportation.
Dolphin Jun summarizes the core reasons leading to the product-side issues of Luobo Kuai Pao into three aspects:
1. The maturity of technology is still relatively low: weak system generalization ability, insufficient humanization and flexibility.
a. Poor interaction and game-theoretic ability with other road participants: not proactively changing lanes to overtake, maintaining a large following distance from the vehicle in front, leading to frequent overtaking and queue jumping by vehicles behind, waiting for all pedestrians/electric vehicles to pass at intersections before proceeding, and exhibiting point braking or even emergency braking when encountering vehicles preparing to change lanes or pedestrians too close;
b. Weak generalization ability of the intelligent driving system: encountering complex road conditions—such as construction zones, solid lines or obstacles in the lane (even plastic bags, woven materials, etc.), narrow sections, long-tail scenarios, and adverse weather (rain and snow can affect the perception capabilities of cameras and LiDAR)—can lead to stalling situations, requiring manual takeover from the backend, and even suspending ride-hailing services in extreme weather conditionsBoth points a & b jointly affect the travel time, users' perception of safety, and passenger experience comfort.
c. Low system flexibility: Passengers can only get on and off at designated stations and cannot freely choose routes or change destinations, which mainly affects the convenience of hailing a ride.
2. Limited number of vehicles for Luobo Kuai Pao: In Q1 2024, only 300 Luobo Kuai Pao vehicles were deployed in Wuhan, resulting in insufficient capacity, which mainly affects the success rate of hailing a ride and user waiting time;
The limited number of vehicles is mainly due to the UE model not being fully operational, still facing significant losses. However, with technological advancements and regulatory relaxations leading to reduced losses, Baidu has been increasing the number of deployed vehicles (planning to deploy 1,000 Luobo Kuai Pao vehicles in Wuhan by the end of 2024). If the UE model is fully operational, the fleet production and supply of Robotaxi in Wuhan can quickly expand.
3. Restricted operational areas for Luobo Kuai Pao: According to Wuhan's policy regulations, the actual operational areas are still mainly in non-core urban areas and non-congested road sections (outside the second ring road). The city center and other bustling areas, as well as complex terrain areas, have not yet opened for operation, which mainly affects the success rate of hailing a ride.
The essence of the regulatory restrictions on operational areas still lies in the low maturity of Luobo Kuai Pao's technology, which cannot interact well with surrounding road participants, potentially leading to safety risks.
In summary, Dolphin believes that the main issue with Luobo Kuai Pao's revenue side still lies in insufficient technology maturity:
① The average distance per order is too low: This is mainly due to the current conservative compliance strategy and weak competitive ability, resulting in longer order durations (close to twice that of taxis). Users primarily engage in short-distance travel and are not in a hurry, leading to low efficiency for long-distance travel;
② Providing significant subsidies to passengers, but with limited impact on daily order volume increase: This is mainly due to "defects" on the product side, and the solution still lies in improving technology maturity.
From the cost side, the two largest cost components in the Luobo Kuai Pao UE model are vehicle depreciation and safety personnel costs:
② Vehicle depreciation:
From the cost perspective in Q1 2024, vehicle depreciation is the largest cost component for Luobo Kuai Pao, accounting for approximately 1.1 times the total GTV for the quarter, which is 3-4 times the depreciation cost of ordinary ride-hailing vehicles.
Baidu has already launched the sixth-generation Luobo Kuai Pao vehicle RT6, with costs reduced from nearly 500,000 yuan for RT5 to about 200,000 yuan for RT6, mainly achieved through front-loading mass production and reducing costs in the lidar supply chain.
Looking ahead to 2025, Dolphin believes that the room for further cost reduction at the vehicle end is limited in the short term. Currently, the cost difference between RT6 and ordinary ride-hailing vehicles mainly lies in the hardware costs for intelligent driving. L4 autonomous ride-hailing is a B2B business, where passengers entrust their personal safety to the operator and cannot intervene in the driving process. Even if there are technological advancements, it still requires more hardware redundancy compared to B2C passenger vehicles to ensure safety, and further cost reductions will still rely on natural reductions in the supply chain
③ Cost of Safety Operators:
When Robotaxi autonomous driving technology is not yet mature, equipping safety operators is still a cost that must be borne by the Robotaxi operation side, and it is the second largest cost on the cost side of LuoBoKuaiPao.
However, the cost of safety operators is currently mainly restricted by regulatory requirements. With the advancement of autonomous driving technology, commercial operations have already transitioned from in-vehicle safety operators to remote safety operators in some cities. According to the "Guidelines for Transportation Safety Services of Autonomous Vehicles" issued by the Ministry of Transport of the People's Republic of China, the ratio of remote safety operators to vehicles must not be less than 1:3.
Looking ahead, the decline in the cost of safety operators mainly depends on the continued relaxation of regulations regarding the ratio of remote safety operators to vehicles, but the relaxation of regulations still depends on the safety improvements brought about by advancements in autonomous driving technology.
Summary:
From the above analysis of the LuoBoKuaiPao UE model, it can be seen that the biggest difference between the Robotaxi and other platform economy business models is that the platform economy relies more on the downward marginal cost brought about by economies of scale, while the real core factor for the Robotaxi business model to succeed lies in the improvement of technological maturity (the essence of policy also needs to be driven by technological maturity to push for policy relaxation). This is also the reason why the first-mover advantage of Robotaxi's early layout is not significant, and the number of Robotaxi vehicles invested by various companies is not large under the condition of immature technology.
II. What advantages does Tesla have in doing Robotaxi compared to LuoBoKuaiPao?
From the current players laying out Robotaxi, the technical paths can be divided into two routes: modular architecture and end-to-end architecture, with leaders being Waymo/LuoBoKuaiPao vs. Tesla.
The biggest difference between modular architecture and end-to-end architecture is:
① Modular architecture is rule-driven: The performance of autonomous driving is based on rules defined by engineers. The more detailed and comprehensive the rules are, the higher the corresponding autonomous driving performance will be, so the system's lower limit will be higher, as the rules written by humans must comply with traffic regulations.
② End-to-end architecture, on the other hand, is data-driven: The performance of autonomous driving is based on trained neural network models (self-learning systems), and how smart the model is mainly depends on whether the learning data is excellent and rich enough (videos of excellent drivers driving + videos of encountered corner cases).
The advantages of end-to-end architecture are also obvious; the learning materials come from the driving behavior of excellent drivers, making the anthropomorphism of the autonomous driving system higher, and the upper limit of autonomous driving performance also higher. However, due to the "black box" nature of large models, the output results have high uncertainty, and thus the lower limit of autonomous driving may also be lower (and may even lead to violations of traffic regulations)From the performance of the leading player Tesla in end-to-end systems, Tesla began switching to the end-to-end route with the V12 version, but the trend of MPI is also unstable.
Data Source: FSD Tracker
Therefore, L4 players represented by Baidu's Luobo Kuaipao face difficulties in switching to the end-to-end route due to limited driving data, and on the other hand, L4 autonomous driving prioritizes safety and must be accountable to regulators (the requirements for interpretability are much higher than for passenger vehicles, while the downside of end-to-end systems is poor interpretability and low limits for autonomous driving; currently, the MPI of leading end-to-end players is unstable).
From the perspective of algorithm architecture, Luobo Kuaipao is still using a modular solution based on high-definition maps (high-definition maps can greatly reduce the complexity of the autonomous driving system's algorithms), where the perception module employs a neural network algorithm that fuses multiple sensors: "LiDAR + cameras + millimeter-wave radar," while the decision-making and control module still uses traditional rule-based algorithms to ensure sufficient safety redundancy.
However, the inherent drawbacks of the modular architecture are also limiting the true maturity of L4 autonomous driving technology. Dolphin Jun believes that compared to Luobo Kuaipao, which uses a modular architecture, Tesla, which adopts an end-to-end route, is more capable of realizing the commercialization model of Robotaxi:
1. The generalization capability of the end-to-end architecture is stronger:
The key to the successful operation of Robotaxi lies in its strong generalization capability, which is reflected in two aspects: on one hand, it can operate in all areas of the city (strong ability to handle complex scenarios and corner cases), and on the other hand, this model can be quickly expanded nationwide.
The core advantage of the end-to-end architecture lies in its strong generalization capability. Because of its high degree of humanization, it has a strong game-theoretic ability with road participants, making it highly adaptable to various scenarios, and it does not rely on high-definition maps (Luobo Kuaipao's strong reliance on high-definition maps is because they provide prior information, reducing the complexity of rule-based code), allowing for rapid nationwide expansion.
In contrast, Luobo Kuaipao's rule-based + high-definition map solution crashes in slightly complex scenarios and cannot exhaust all corner cases. Therefore, the modular solution is more suitable for routes with well-developed traffic infrastructure and simple road conditions, making it less capable of operating in all areas of a city.
Moreover, high-definition maps involve data privacy and security issues, and the approval process for commercial use is slow. The collection of high-definition maps also requires sending mapping vehicles to run the routes in each city first, allowing for city-by-city expansion (similar to how car manufacturers gradually opened cities for NOA), resulting in poor scalability2. Stronger end-to-end timeliness and better humanization:
End-to-end solutions have higher game-theoretic capabilities, stronger humanization, and more aggressive driving strategies, resulting in better timeliness and driving experience compared to modular solutions.
According to research, if a human driver takes half an hour to commute from point A to point B, Tesla V13 can control it to around 40-45 minutes, while Waymo takes over an hour (having been deploying Robotaxi commercial services in Phoenix for more than 4 years).
For long-distance driving, a one-hour journey for a human driver takes Waymo 2-3 hours, indicating very weak timeliness.
3. Rapid technological advancement of end-to-end solutions, while modular solutions can only improve linearly:
Rule-based methods rely on patch-like approaches to write scenarios, so the improvement in intelligent driving capabilities can only progress linearly, making it difficult to achieve qualitative leaps in technology. In contrast, data-driven end-to-end models have the potential for advancements similar to ChatGPT, achieving scaling law.
Summary:
Although the current implementation speed of end-to-end solutions is slower than that of modular solutions, Dolphin believes that the modular solution adopted by $Baidu.US, due to inherent flaws in its technical route, cannot resolve the inherent defects in providing passenger services, and the degree of technological advancement has become limited.
On the other hand, Tesla, which adopts an end-to-end solution, is closer to the passenger services provided by ride-hailing in terms of generalization, humanization, and timeliness, and has a rapid pace of technological advancement, possessing the true potential to achieve L4 passenger services.
3. How much improvement can the UE model achieve after the maturity of the Robotaxi business?
In the article "Tesla 'Darkly' Plans, Is the Robotaxi Story Just a 'Facade'?," Dolphin has mentioned the changes Robotaxi brings to the traditional ride-hailing UE model, concluding that:
The cost borne by drivers remains unchanged (vehicle depreciation, fuel/electricity costs, etc.), while previously, nearly half of the ride-hailing fees paid by users went to cover the driver's labor costs. Robotaxi fundamentally changes this by redistributing the saved 49% (assuming all ride-hailing vehicles are new energy electric vehicles, adjustments have been made to the UE model compared to the previous article).
But Dolphin ignored two factors:
① Robotaxi can theoretically operate 24 hours a day, and the increase in operating hours can lead to an increase in the number of orders per vehicle per day, thereby significantly increasing GTV. However, in reality, this theory has limitations:
- The operating time of Robotaxi is limited by the lifespan of batteries and components, making 24-hour operation impossible:
a. If vehicle components operate at high saturation (especially industrial control computers and onboard computers) for extended periods at full load, it will reduce their lifespan and reliability.
b. The battery itself has a limited number of charge cycles, and without a significant improvement in battery lifespan, it also restricts the actual operating time of Robotaxi models.
Therefore, currently, the actual operating time of Robotaxi is about 12 hours, slightly exceeding the average operating time of ride-hailing vehicles at 10 hours.
- The daily order volume of Robotaxi is also affected by supply and demand:
The shared mobility market itself is relatively limited in scale, and the product offered by Robotaxi has not fundamentally changed compared to ride-hailing (point-to-point passenger service). Therefore, it can only penetrate the shared mobility market by lowering prices.
Assuming that Robotaxi technology and operations mature, Robotaxi can save on driver costs and pass on 30% of GTV to passengers as rebates to achieve full penetration of the shared mobility market (ordinary ride-hailing rebates 10% of GTV), while also stimulating an overall increase in demand for shared mobility. The market size of shared mobility in China could reach trillions after Robotaxi matures (optimistic assumption).
If we assume an average daily revenue of 600 yuan (GTV) per Robotaxi, China would only need 6.6 million full-time Robotaxi vehicles (working 360 days a year, averaging 12 hours a day) to meet the entire market demand, and the expansion of Robotaxi capacity is also very easy.
If passenger autonomous vehicles can also join the Robotaxi fleet for operation, the market could easily become saturated, or even experience oversupply, fundamentally remaining a demand-driven rather than supply-driven business.
② Robotaxi also needs to bear additional costs that were originally borne by drivers:
Robotaxi incurs additional costs for cleaning, inspection, and ground operations when encountering faults, so it still requires additional ground staff, resulting in extra costs (which were originally borne by ride-hailing drivers)Due to these two limiting factors, Dolphin adjusted the UE model for Robotaxi after it matures (after technology matures and operations are optimized):
In the assumptions regarding GTV (GTV = average daily orders per vehicle * nominal price per kilometer * average kilometers per order):
a. The actual operating time of Robotaxi is still limited. Based on the longer actual operating time of Robotaxi (12 hours vs 10 hours) and providing higher passenger incentives due to saved driver costs, Dolphin assumes that the daily order quantity per vehicle increases from 20 orders for ride-hailing to 25 orders for Robotaxi.
b. Dolphin assumes that the average kilometers per order for Robotaxi remains unchanged compared to ride-hailing (mainly determined by usage scenarios, which currently still focus on short-distance travel for ride-hailing).
From the results, it can be seen that even though the daily GTV of Robotaxi is higher than that of ordinary ride-hailing (increased daily order quantity per vehicle), the self-operated cost rate is still slightly higher due to:
① Higher vehicle costs (assuming the cost of autonomous driving domain controllers + cameras in a mature state) is 20,000 yuan higher than ordinary ride-hailing (180,000 yuan vs 160,000 yuan);
② Assuming the cost of ground staff (assuming ground staff: vehicles = 10:1) is the main influencing factor;
③ Higher maintenance costs (increased maintenance costs for autonomous driving hardware and software).
The actual operating cost rate of Robotaxi is still slightly higher than the driver cost rate (Robotaxi self-operated cost rate 36% vs ride-hailing driver cost rate 31%), and the portion of driver income savings that Robotaxi can redistribute from GTV decreases from 49% to 44%.
If the platform and autonomous vehicle providers jointly participate in operations, the net cash flow portion for drivers (44% of GTV) will be distributed to:
① Platform; ② Autonomous vehicle providers; ③ Discounts for passengers.
If Robotaxi is to achieve full penetration of the shared mobility market, Dolphin believes it needs to offer 25% more GTV in discounts to passengers compared to ride-hailing (ride-hailing offers 10% more GTV in discounts to passengers compared to traditional taxis, but has not achieved penetration into the traditional taxi market), which means that the platform and autonomous vehicle providers would only barely receive 20% of GTV.
In the case of vertically integrated self-operation (providing Robotaxi vehicles + self-operation + self-built platform), the portion of revenue based on GTV under the ride-hailing model (driver 49% + platform 10% + passenger rebates 10%) changes to a distribution of 64% of GTV for the Robotaxi vertically integrated self-operation model, allocated to: ① Robotaxi self-operation provider; ② Passenger rebates.
Similarly, if 35% of GTV is offered as discounts to passengers, the Robotaxi self-operation provider will receive about 30% of GTV
4. How much valuation can Robotaxi contribute to Tesla?
From the user perspective, the product offered by Robotaxi has not fundamentally changed compared to ride-hailing services. Owning a self-driving vehicle is superior to taking a Robotaxi ride in terms of cost and other attributes of vehicle ownership (such as status/toy attributes), making it difficult for the shared mobility market to penetrate the passenger vehicle market.
Therefore, due to the limited market size of shared mobility and the potential for oversupply once self-driving technology matures (especially as passengers' own self-driving vehicles can also join the Robotaxi fleet), it is believed that the evolution of the Robotaxi business will ultimately be a competition among mobility platform providers (as long as the supply of Robotaxi vehicles is not restricted and can freely join various mobility platforms), resulting in limited profit margins.
Thus, for the valuation of Tesla's Robotaxi business, a simple assumption is made for reference:
- Assume that in the U.S. market, whether it is Tesla-operated vehicles or passengers' own self-driving vehicles (whether Tesla or vehicles from other manufacturers authorized by Tesla's FSD), they can only use Tesla's ride-hailing platform.
In terms of Robotaxi deployment, since currently only Tesla has the capability to operate an end-to-end route in the U.S., it is assumed that Tesla occupies a leading position in Robotaxi, achieving an 80% market share in the U.S. Robotaxi market (currently Uber's market share in the U.S. is around 75%).
At the same time, it is assumed that after Robotaxi matures, by providing 30% of GTV as rebates to passengers, it will achieve full penetration of the U.S. ride-hailing market, while also expanding the overall shared mobility market size (increased user frequency leading to order growth), with the mature U.S. Robotaxi market size reaching $83.5 billion (currently around $60 billion).
Even so, assuming a full-time Robotaxi vehicle generates an annual GTV of $90,000 (based on a GTV of $10 per ride, 25 rides a day, operating 360 days), considering population density, operational area, and coverage issues, the U.S. Robotaxi market would require about 1.6 million full-time Robotaxi vehicles. If Tesla occupies 80% of the market share, it means Tesla operates around 1.3 million Robotaxi vehicles.
Finally, based on Tesla's profit model for Robotaxi in the U.S., it is reasonably estimated that Tesla's net profit/GTV ratio is around 26%, resulting in an annual net profit of $17.3 billion for Tesla's Robotaxi in the U.S. With a valuation of 20 times PE for the mature Robotaxi business, the discounted valuation of Tesla's U.S. Robotaxi business is approximately $170 billion
② In the Chinese market, due to the following factors, Dolphin believes that the Robotaxi business in China should not be valued.
a. There are many players capable of operating end-to-end routes, making it difficult for Tesla to dominate the market alone;
b. Due to regulations, safety, and various other factors, it is unlikely that Tesla will be allowed to enter into exclusive agreements that grant it a monopolistic position in the Chinese shared mobility market;
c. The Chinese Robotaxi market will likely end up being a competition among mobility platform providers, with limited profit margins, making Tesla's layout less significant;
5. Where are the real opportunities after L4 autonomous driving matures? Is Tesla's current valuation overestimated?
In the article "Tesla FSD: Can the Stars and the Sea Stand the Test of Reality?," Dolphin mentioned that the purpose of Tesla's Robotaxi project is to cultivate user mindset, ultimately giving rise to Tesla's next true annual production of 4 million vehicles, capable of integrated hardware and software delivery (where owners are willing to pay for software), using FSD software to promote hardware vehicle sales, and then charging separately for FSD, achieving a closed-loop ecosystem similar to Apple's hardware-software integration.
Therefore, Dolphin believes that when L4 autonomous driving technology matures, the real opportunity lies not in Robotaxi, but in the monetization of car manufacturers' vehicle sales + intelligent driving software business:
① The maturity of autonomous driving technology can spur a new wave of global vehicle replacement cycles (the overall vehicle sales market size continues to expand);
Referencing the mobile phone industry, after 2007, Apple began releasing the iPhone, marking the start of the smartphone era, and global mobile phone shipments rapidly increased.
② The concentration of the global automotive market may continue to increase, while also leading to an increase in vehicle sales market share;The maturity of L4 autonomous driving technology may lead to a continued increase in the global automotive CR5 concentration, while Tesla currently has an advantage over other new energy vehicle companies in the globalization of new energy vehicles.
The establishment of Apple's integrated hardware and software closed loop has rapidly increased Apple's global smartphone market share: In 2010, Nokia was still the world's largest mobile phone manufacturer, holding about 33% of the global smartphone market share. However, in 2011, Nokia's market share dropped to 23% (after the release of the iPhone 4). After 2013, Nokia's mobile phone business was sold to Microsoft, effectively exiting the competition in the mobile phone market.
The new energy vehicle market may also follow a similar evolutionary trend. In 2023, the global penetration rate of new energy vehicles was still only 15.8%. Due to the higher compatibility of new energy vehicles with intelligent hardware and software, the elimination of fuel vehicle companies will accelerate. Tesla, with its mature FSD software, may replace Toyota as the global "dominant player" in smart vehicles, just as Apple replaced Nokia.
③ The high gross margin (over 90%) of charging for intelligent driving software can also enhance overall profit margins:
Apple's service business high gross margin raised Apple's overall gross margin from 33% in 2007 to 46% in 2024.
Dolphin assumes that Tesla's FSD autonomous driving technology can mature to L4-L5 level, achieving Apple's "App Store" moment, and valuing the car sales hardware and FSD business separately:
① Car sales business valuation: Successfully replacing Toyota's dominant position, achieving a valuation of 700 billion for car sales
Assuming global car sales of 80 million units (global sales in 2023 were 77.69 million), after the maturity of intelligent driving in 2032, it becomes Tesla's core competitive barrier. Tesla successfully replaces Toyota as the new generation of car sales leader, achieving a global market share of 13%. When the selling price of cars drops to 35,000 yuan, annual sales reach 10.4 million units (using affordable cars for market share), and finally, Tesla's annual car sales revenue reaches 364 billion yuan.
At the same time, for the hardware gross margin of car sales, we assume a steady-state gross margin of 25% for Tesla's automotive business (slightly exceeding Toyota), and a cost rate of 6% under scale effects. Tesla's operating profit after tax adjustment is around 55 billion.
Finally, referencing Apple and Toyota, we assign Tesla's car sales business a valuation of 25 times PE, discounted to 2025, Tesla's car sales valuation is around 700 billion② FSD Business Valuation: Assuming FSD evolves into a fully subscription model (the most favorable model for Tesla)
a. Tesla's existing vehicle FSD subscriptions:
Assuming FSD matures in volume by 2032, Tesla will have approximately 44.6 million existing vehicles by 2032. Assuming a monthly price of $65 (currently $99/month) achieves a 70% paid penetration rate for Tesla's existing vehicles, Tesla's FSD annual revenue will reach $24.6 billion.
b. Opening third-party FSD licensing:
Assuming there are 21.6 million third-party paid users (based on the assumption of a 50% penetration rate for global new energy vehicles by 2032, with approximately 300 million existing new energy vehicles globally, and third-party users using Tesla's FSD accounting for about 8% of global new energy vehicles), with the monthly subscription price unchanged and Tesla taking a 50% cut, Tesla's annual revenue from third-party FSD licensing will be $8.5 billion.
Finally, applying a 25x P/S software valuation to Tesla's FSD business, the discounted valuation of Tesla's FSD business by 2025 will be $420 billion.
Final comprehensive valuation:
It can be seen that even if all optimistic expectations for mature autonomous driving technology have been fully accounted for, Tesla's market value is only around $1.4 trillion (implying a 2025 P/S multiple of 11 times). This is already based on all the grand visions that Dolphin has painted for Tesla, assuming they will be realized around 2030.
If there is one area that is not optimistic enough, it is based on the current actual layout of various smart car companies, which does not allow for the FSD penetration rate of global non-Tesla smart vehicles to be mindlessly raised to double digits or 50%, nor does it account for the valuation of Optimus.
Under such extremely optimistic assumptions, it is merely Tesla's current market value. Clearly, the market has priced in too many uncertain future elements for Tesla at present.
6. Which players can still enjoy the opportunity as intelligent driving gradually matures?
Based on the above analysis, Dolphin believes that the real opportunity after the maturity of L4 autonomous driving still lies with automakers, replicating a similar "App Store" moment as Apple did, achieving a closed-loop integration of software and hardware.
Similar to the product requirements for ride-hailing services regarding passenger services, Dolphin believes that passenger car owners' requirements for intelligent driving experiences also follow three dimensions: scenario adaptability, traffic efficiency, and human-like interaction.
The continuous optimization process of these three dimensions corresponds to consumers' subjective feelings towards intelligent driving systems, from usable to user-friendly to beloved. Currently, in terms of technology route selection, the end-to-end technology route remains the most promising path to truly achieve L4-L5 autonomous driving technology.
Therefore, Dolphin believes that automakers that can closely follow the end-to-end technology route are most likely to enjoy the opportunity as intelligent driving matures
The end-to-end route has achieved a transformation of autonomous driving algorithms from rule-driven to data-driven, making high-quality data + backend training computing power the core competitive factors, with the reserve of technical talent in intelligent driving as an auxiliary.
The key to obtaining high-quality data lies in the sales volume of high-level intelligent driving hardware models (only vehicles equipped with high-level intelligent driving hardware can complete high-quality driving data). The training computing power + intelligent driving technical talent essentially represents a capital competition (corresponding to increased capital investment/R&D investment). Therefore, it is expected that when intelligent driving technology is not yet mature and domestic car companies have not been able to charge separately for intelligent driving software, the following closed loop will form:
Progress of Leading End-to-End Players
However, looking at domestic car companies, the highest valued XPeng is only at 1.2 times P/S, while other new energy vehicle companies generally have P/S multiples of less than 1, basically not incorporating expectations of monetizing intelligent driving into their valuations, which still rely on hardware sales for valuation. Dolphin Jun believes that under the trend of this wave of intelligent evolution, domestic leading players closely following the end-to-end route, $ XPeng.US and $ Li Auto.US, have greater opportunities.
The potential catalysts for valuation improvement in the future are:
① The maturity of intelligent driving technology improves, leading to a significant enhancement in the intelligent driving experience, reflected in a. Rapid increase in urban MPI; b. Improvement in urban traffic efficiency (the time to complete the same travel route is closer to human driver performance); c. Expansion of complex urban scenarios/functions and increased success rates (currently, end-to-end competition focuses on parking space to parking space functionality).
② On the passenger side, when intelligent driving software is charged separately, the main observation is the increase in the penetration rate of intelligent driving software. However, when intelligent driving is not charged separately, it is reflected in a. A significant increase in the number of active users of urban intelligent driving; b. A significant increase in the proportion of users choosing high-level intelligent driving models when opting for intelligent driving; c. In the case of standard intelligent driving, an increase in sales of models chosen by users due to intelligent driving factors, forming popular models③ The potential catalyst for Tesla's FSD entering China may generate a "silver carp effect," accelerating the improvement of domestic intelligent driving technology and possibly cultivating users' awareness of paying separately for intelligent driving, prompting domestic car manufacturers to initiate a separate charging model for intelligent driving software;
④ The issuance of national-level regulations and licenses for L3 and above means that after activating the intelligent driving mode, the main responsibility for accidents caused by the intelligent driving mode shifts to the car manufacturers, rather than the car owners.
History of Intelligent Driving Research Articles:
On January 2, 2025, "Tesla FSD: Can the Stars and the Sea Withstand Reality's Test?"
On December 3, 2024, "Tesla's 'Dark Maneuver': Is the Robotaxi Story Just a 'Cover'?"
The copyright of this article belongs to the original author/organization.
The views expressed herein are solely those of the author and do not reflect the stance of the platform. The content is intended for investment reference purposes only and shall not be considered as investment advice. Please contact us if you have any questions or suggestions regarding the content services provided by the platform.