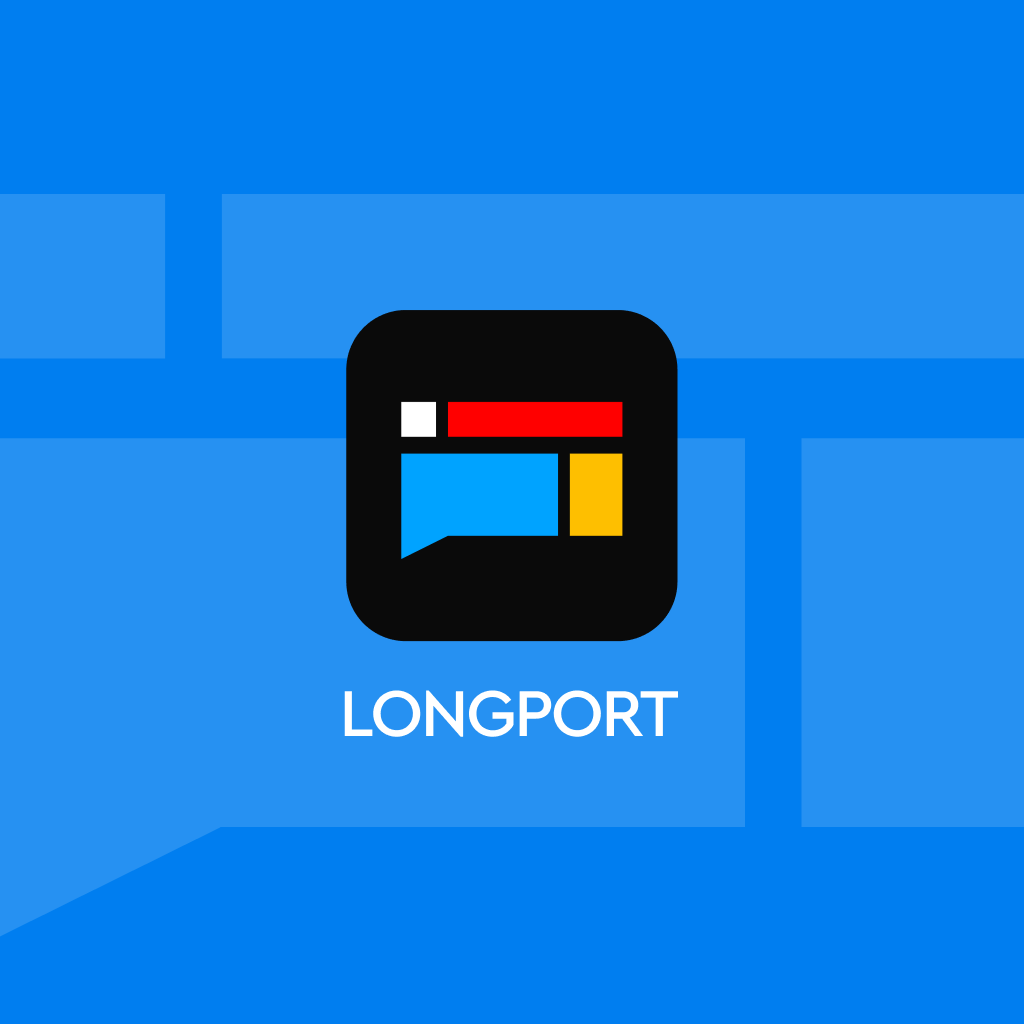
Can AI make money now?
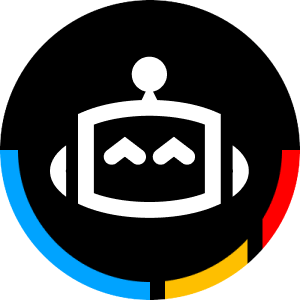
The development of AI technology from 0 to 1 has actually never been completed.
Recently, various large-scale models have been intensively released, and opinions such as "catching up with GPT-4" and "becoming China's OpenAI" can be seen in various articles. But let's go back to the fundamental question: Can AI make money?
This is a soul-searching question for people in this industry.
If we ask this question without the groundwork of the past ten years, it may seem a bit impatient. However, with the foundation of ten years of losses, whether or not to make money becomes a combination of technology and business: it is both a touchstone for technology and a proof of commercialization. Before answering this question, we need to summarize the potential business models behind AI.
Potential Business Models behind AI
If AI develops to a very mature stage, what are the potential monetization methods? There are not many proven business models in the past, and there are only a few that match AI:
First, subscription. This is almost the most typical monetization channel for AI at present. It is actually a kind of cloud service, and major cloud providers naturally integrate their self-developed AI functions into their own cloud product matrix.
Second, new value-added services. The movie "Her" is actually a new type of value-added service, and future electronic pets that can communicate are also in this category. Compared with the first category, the difference is that this category is the final dish that is made, while the first category is the raw material. There will be many overlapping areas between the two, but the difference is similar to the difference between PaaS and SaaS. In the past, we often said that SaaS is closer to new value-added services, so we will not list them separately, such as various enterprise-level assistants.
Third, hardware product sales. This category is similar to Lenovo selling computers. Multi-modal large models need this as support. Without the success of large-scale new intelligent product categories such as robots, smart speakers, and AR glasses, the success of multi-modal large models is unlikely. In terms of industrial division of labor, this model will overlap with the previous two and become a driving force for them.
Fourth, new advertising. Some people have said that large models will make it difficult to display search ads. I don't think so at all. The screens are large enough, and even direct recommendations can be made: "If you want to buy, please look at...". The key is to increase the frequency and accuracy.
Fifth, solution sales. Products like Watson are unlikely to become completely standard products. They always need to be connected to various specific situations around them, which inevitably requires solution integration. From a technical perspective, it seems similar to the second and third categories, but from a business model perspective, the difference is very large and needs to be listed separately. New value-added services and hardware product sales are still standard products, and the unit price is limited to the upper limit of iPhone or Vision Pro. But solutions are not. The unit price here must be very high, such as tens of millions, to be valuable, otherwise it cannot support the long-term investment in the early and later stages. To some extent, the AI transformation of existing products will actually become a solution, such as e-commerce, short videos, etc., and industry-wide large models are even more so. Here, AI is not a disruptive force, but a solution that enhances existing products. Sixth, Games and Metaverse. This may seem like a product, but it is significantly different from the first five in that it supports a virtual central bank model. The virtual central bank model refers to the ability to issue its own tokens (not necessarily digital currency). Only this type of product supports a separate ecosystem and monetary system.
If we divide these business models vertically, there are two obvious common characteristics:
First, as mentioned in the previous article, AI is actually a deep well drilling model, manifested as an update to existing models (including humans). The result is that creating new models is not as impactful as the internet, but the impact on existing models is greater than that of the internet.
Second, the economic value of technology is more likely to be personified, doing what humans do in the economic system and surpassing them. (Various assistants, etc.)
These two points are crucial because they directly affect who will make money from AI and the potential end state.
Who will ultimately make money from AI?
The above characteristics of AI determine that it is actually a link in the supply chain. As a result, in addition to 1, companies that want to make their own models work must become the kind of company they are not yet. For example, even for relatively lightweight games and metaverse, it means that large model companies need to force themselves to become companies that understand games and metaverse.
This further raises two questions:
First, if it is in the form of cloud services like 1, will AI be a separate cloud service or part of existing cloud services?
Second, if it needs to be integrated with a certain existing field, will it be purely AI companies leading the way or will the native companies in that field evolve and take the lead?
For the first question, I think the answer is quite clear. Cloud services cannot exist as a separate category, they will definitely be consolidated. This is determined by the inherent economies of scale in the heavy asset industry.
For the second question, the answer is also quite clear. In different fields, the weight of the field and the weight of the technology are different. For example, the weight of the gaming field is low, while the weight of the tax and medical fields is high. The higher the weight of the field, the less likely it is for a purely technical AI company to dominate. And in reality, the weight of the field is often high. It is difficult to say which specific company, but the balance between technology and domain knowledge will be crucial.
The Business Challenges of Making Money from AI
The previous article focused more on the commercialization process from a technical perspective, while this time we are looking at it purely from a business model perspective, and the conclusion is not complicated:
Pure AI companies face obvious business challenges. If they remain in the supply chain, the monetization channels are too narrow. To successfully complete the final stage, they not only need to handle the models but also the products (products represent the fusion of domain knowledge and technology).
It seems that the future may unfold as follows: If the top-tier large model companies cannot establish other monetization channels and are limited to the supply chain, they are more likely to be acquired by large cloud companies. The middle-tier domain models are more likely to gradually evolve and succeed with the combination of domain knowledge, such as publishing companies having a greater chance of success in content moderation products compared to outsiders. All analysis and judgment of business models require one foundation: the technology itself must be sufficient to create value. So how mature is the technology? Is it enough?
Is it really enough? In reality, when trying to implement a product in a real scenario, it is often found that the technology supply is still insufficient, and it is also impossible to completely meet the demand in the short term.
The development of AI technology from 0 to 1 has not actually been completed. This is a significant difference between AI and previous technologies such as the Internet.
To some extent, around the year 2000, many of the basic technologies of the Internet were already in place, and what remained was the improvement in speed and scale. (The basic Internet protocols we still use today, such as TCP/IP and HTTP, are technologies from a long time ago.)
But AI is different. Its foundation has been continuously improving, and all corresponding applications need to be perfected while being applied.
A comparison between the two reveals that the development of the Internet was a step-by-step process, while AI has been growing by constantly deceiving itself. We announce today that we have solved one problem, and tomorrow we announce that we have solved another problem, but the progress of large models is far from what we initially expected. (And the success of large models also has a touch of magical realism, even from companies whose founders do not have an AI background.)
So, if we look at it from the perspective of sufficiency, then it is indeed not enough. But even if it is not enough, AI is like water. The parts it submerges will undergo a complete transformation, and the corresponding functions will undergo a thorough change. For example, in terms of image creation, basic illustrations will no longer require human artists.
Apart from sufficiency, how can we judge the technological maturity of a product? Or whether it is sufficient or not?
In fact, we can use the method of comprehensive scenario coverage, and from a business perspective, it can only be comprehensive scenario coverage. As mentioned earlier, the commercial path of AI always exhibits a kind of personification, but humans live in a certain relationship and cannot handle the corresponding comprehensive relationships, so it is not personified enough.
Comprehensive Scenario Coverage
AI is prone to becoming self-referential, using technical indicators to measure itself, but this can become a self-referential loop, and the extreme is deceiving oneself.
AI fundamentally tests its general capabilities. If a specialized method can perform exceptionally well on any test set in theory, surpassing any existing artificial intelligence, it is of no use other than creating PowerPoint presentations. Because when AI is implemented, the personification of AI means that it intersects deeply with complex environments in any given scenario, which still requires general capabilities. This specialized evaluation method actually constitutes the fundamental reason for the mixed reactions towards AI: on one hand, AI seems to be capable of doing everything and is incredibly magical; on the other hand, it is not user-friendly and does not generate profits.
What is the "full-scenario coverage" approach?
In simple terms, it means that if recruitment is a scenario, does the technological supply support the direct creation of a digital employee who can perform all the functions of a traditional recruiter? For example, if given a requirement, the digital employee can complete the entire recruitment process without human intervention.
If this cannot be achieved, except for the first model, none of the high-value models behind it will work.
This is indeed a real challenge.
Summary
Upon careful consideration, it is evident that the new fields in the post-internet era each have their own difficulties. If we go back to around 2015, there were roughly three new directions: artificial intelligence, blockchain, and SaaS. Many individuals who felt that the internet had reached its limit and were unwilling to be lonely ventured into these three fields. However, artificial intelligence and SaaS have not been profitable for 10 years, while blockchain made money but disappeared due to other reasons. Now, it seems that large models can inject new vitality into these three areas simultaneously and are approaching the final stage. Every time I see a graph like the one below, I believe in this even more:
Many people are concerned about which field will come first. This cannot be specified, but there can be a basic judgment model:
Assess how far the path is from technological creation of new value to commercial value. The Midjourney is actually short, while Watson is long. Realizing this requires matching funds, manpower, and this length.
Source: Zhuomoshì, Original Title: "Can AI Make Money?"