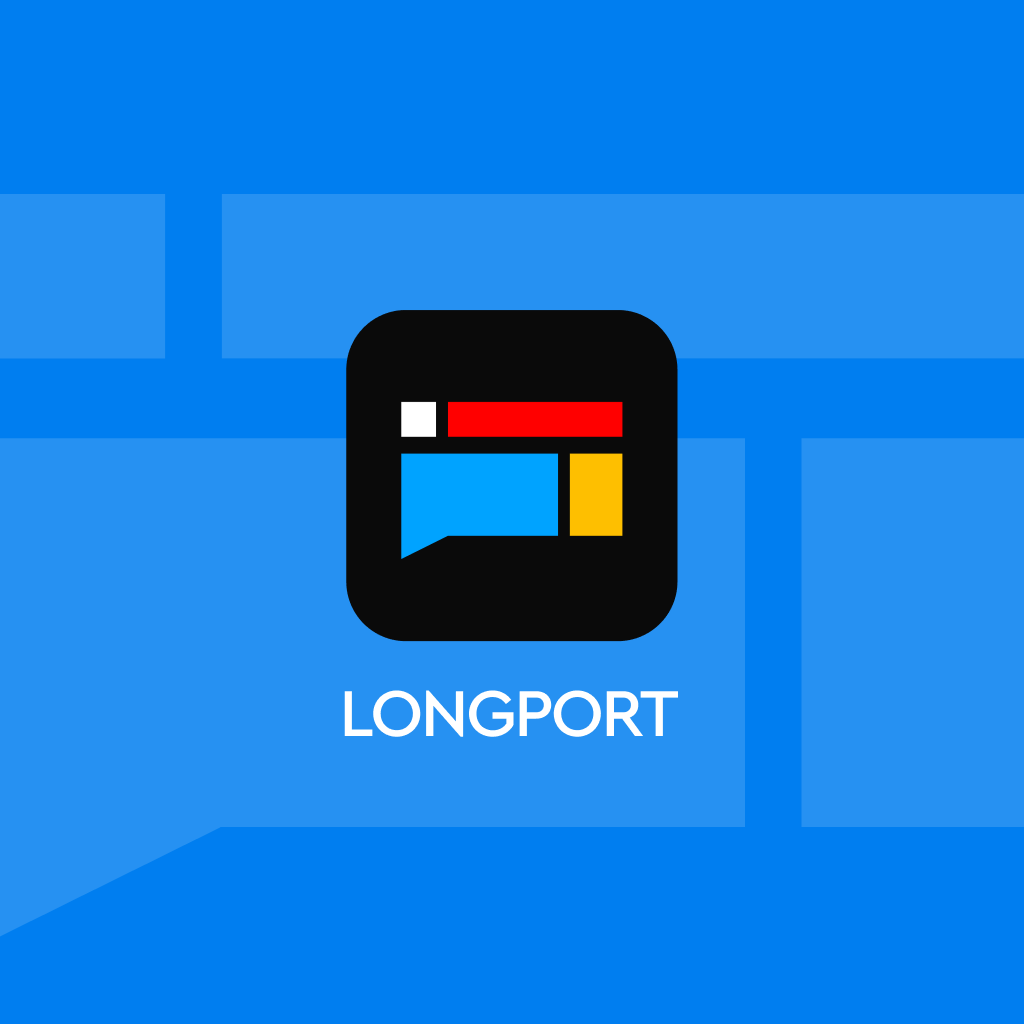
NVIDIA's fiscal year Q4 earnings conference: Data center revenue quadrupled year-on-year to a historic high, marking a turning point as the world enters a new era of computing.
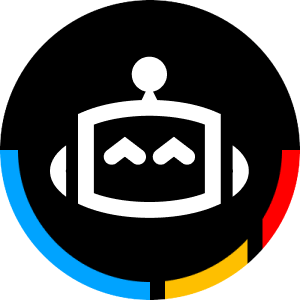
In NVIDIA's Q4 FY24 earnings report, the data center revenue increased fourfold year-on-year, reaching a record high of $18.4 billion, a 27% increase quarter-on-quarter, and a 409% increase year-on-year. NVIDIA pointed out that the world has reached a turning point in the new era of computing, with data center infrastructure transitioning from general-purpose computing to accelerated computing. The company estimates that approximately 40% of last year's data center revenue came from AI inference.
Zhitong App learned that on February 22nd, NVIDIA held its Q4 earnings conference for the 24th fiscal year. NVIDIA reported that its revenue in the fourth quarter reached a record high of $22.1 billion, a 22% increase MoM and a 265% increase YoY, far exceeding the expected $20 billion. For the fiscal year 2024, the revenue reached $60.9 billion, a 126% increase from the previous year. In the data center sector, the revenue for fiscal year 2024 was $47.5 billion, more than triple that of the previous year.
NVIDIA pointed out that the world has reached a turning point in the new era of computing. The installation base of data center infrastructure worth trillions of dollars is rapidly transitioning from general computing to accelerated computing. With the slowdown of Moore's Law and the surge in computing demand, the company is accelerating all possible workloads to drive future performance, cost, and energy efficiency improvements. Meanwhile, enterprises are starting to build the next generation of modern data centers, known as AI factories, specifically designed to refine raw data and generate value in the era of artificial intelligence.
In the fourth quarter, driven by the Nvidia Hopper GPU computing platform and InfiniBand end-to-end network, data center revenue reached $18.4 billion, hitting a historic high with a 27% MoM increase and a 409% YoY increase. Computing revenue increased by over 5 times, and network revenue doubled.
The growth of the data center in the fourth quarter was driven by the training and inference of generative AI and large language models across different industries, use cases, and regions. The versatility and leading performance of the company's data center platform can bring high return on investment for many use cases, including AI training and inference, data processing, and a wide range of CUDA-accelerated workloads. The company estimates that about 40% of last year's data center revenue came from AI inference.
The construction and deployment of AI solutions have penetrated almost every industry. Many companies across various industries are scaling up the training and operation of their AI models and services. Enterprises are using Nvidia AI infrastructure through cloud providers, including hyperscale, GPU-dedicated clouds, private clouds, or on-premises clouds. Nvidia's computing stack can seamlessly scale across cloud and on-premises environments, allowing customers to deploy multi-cloud or hybrid cloud strategies. In the fourth quarter, major cloud providers accounted for more than half of the data center revenue, supporting internal workloads and external public cloud customers.
Q&A
Q: Regarding the data center business, what changes have occurred in the past quarter in terms of expectations for 2024-2025?
A: We guide on a quarterly basis, but fundamentally, the conditions for sustained growth in 2024 and 2025 and beyond are very good.
We are at the beginning of two industry-wide transformations. The first is the shift from general computing to accelerated computing. General computing is losing steam, as evidenced by communication service providers and many data centers (including our own) extending their depreciation time from four years to six years. When you can no longer significantly increase throughput as before, there is no reason to update more CPUs. So, you have to accelerate everything.This is an area that Nvidia has been exploring for some time. By accelerating computing, you can significantly improve energy efficiency and reduce data processing costs by 20 to 1, which is a huge number. Of course, there is also an improvement in speed.
This speed has led to the second industry-wide transformation, namely generative artificial intelligence. Generative artificial intelligence is a new application that supports a new way of software development, creating new types of software. It's a new way of computing. You can't do generative artificial intelligence on traditional general-purpose computing; you have to accelerate. It is giving rise to a whole new industry that is worth taking a step back to examine. It relates to your final question about sovereign artificial intelligence.
Data centers are no longer just about computing data, storing data, and serving company employees. We now have a new type of data center focused on artificial intelligence generation, an artificial intelligence generation factory. It takes raw materials (data), transforms them with Nvidia-built artificial intelligence supercomputers, and converts them into extremely valuable tokens. These tokens are what people experience in ChatGPT, the metaverse, or enhanced search. Now, all your recommendation systems have been enhanced, with hyper-personalization, and all these incredible startups in the field of digital biology are producing proteins and chemicals, the examples of which are countless. These tokens are generated in a very specialized data center, which we call the artificial intelligence supercomputer and artificial intelligence generation factory.
We see this diversity manifested in new markets.
The amount of reasoning we do is astonishing. Almost every time you interact with ChatGPT, we are reasoning. Every time you use the metaverse, we are inferring. Every time you see amazing videos being generated or edited, Nvidia is reasoning.
The reasoning part of our business has grown by about 40%. As these models become larger, the training volume continues. We are also diversifying into new industries. From capital expenditures and discussions, it can be seen that large communication service providers are still under construction. But there is a new category called GPU-dedicated CSP. They focus on Nvidia's artificial intelligence infrastructure. You will see enterprise software platforms deploying AI, such as ServiceNow, Adobe, SAP, and others. Consumer internet services are now enhancing all their services through generative artificial intelligence to provide more hyper-personalized content.
We are talking about industrial generative artificial intelligence. Our industry now represents a billion-dollar business, including automotive, health, and financial services.
Sovereign artificial intelligence is related to the fact that each region has its own language, knowledge, history, and culture, and its own data. They want to use their data to create their own digital intelligence and offer it for their own use of raw materials. We see Japan, Canada, France, and many other regions building sovereign artificial intelligence infrastructure. My expectation is that what the United States and the West are going through will definitely be replicated worldwide. These artificial intelligence generation factories will be present in every industry, every company, and every region.Last year, we witnessed the rise of generative artificial intelligence as a new application space, a novel computing method, and the formation of a new industry, all of which are propelling our growth.
Question: How do we calculate that "40% of data center revenue comes from AI inference"? What were the historical figures like?
Answer: This percentage may be underestimated. The internet has trillions of items, yet your phone screen has limited space. The ability to compress all this information into such a small area is achieved through recommendation systems. These recommendation systems traditionally relied on CPU methods. However, the recent shift towards deep learning and generative artificial intelligence has led these systems onto the path of GPU acceleration. Therefore, GPUs are now involved in every step of recommendation systems - embedding, nearest neighbor search, reordering, and generating enhanced information all require GPU acceleration. Recommendation systems are the largest software engine on Earth and are indispensable for almost all major global companies.
Whenever you use ChatGPT, generate images with MidJourney, or collaborate with Getty and Adobe's Firefly, you are utilizing inference. These examples, along with others, are 100% new and did not exist a year ago.
Question: How should we understand "the next generation of products will face supply constraints"?
Answer: Firstly, overall, our supply is improving. Our supply chain has done an incredible job for us, ranging from wafers, packaging, and memory to all power regulators, transceivers, networks, and cables. People often think Nvidia GPUs are just a chip, but the Nvidia Hopper GPU has 35,000 parts and weighs 70 pounds. The wiring system behind data centers is the densest and most complex network system in the world to date.
Our InfiniBand business has grown fivefold year-on-year. The supply chain has provided us with tremendous support, and overall, the supply is improving. We anticipate that demand will continue to outstrip supply for the whole year, but we are making every effort to shorten lead times.
However, for new products, the growth from zero to mass production will not happen overnight. Everything is gradually heating up. We are currently strengthening the H100. With our ramp-up, it is impossible to meet demand in the short term. We are also enhancing the Spectrum X, which has shown outstanding performance in the Ethernet field. InfiniBand is the standard for AI-specific systems, and Ethernet, traditionally not a good horizontal scaling system, has been enhanced through Spectrum X. We have added new features such as adaptive routing, congestion control, and traffic isolation to optimize Ethernet for AI. InfiniBand will be our AI-specific infrastructure, and Spectrum X will be our AI-optimized network. For all new products, demand will exceed supply, which is a typical scenario for new product releases. We are working diligently to meet demand as quickly as possible. Overall, our supply is significantly increasing.Q: How many products are currently being shipped to the Chinese market? Will there be other alternative solutions added?
A: The core purpose of the U.S. government is to restrict NVIDIA's latest capabilities in accelerating computing and artificial intelligence technologies in the Chinese market, while also hoping that we can succeed in China under these restrictions. When the new restrictive measures were announced, we immediately stopped to fully understand them and reconfigured our products to meet the requirements, ensuring that they cannot be cracked by software. This process took some time, resulting in a readjustment of our product supply in China. We are now providing samples to our Chinese customers and will do our utmost to compete and succeed in the market within the specified restrictions.
In the last quarter, our business saw a significant decline due to the suspension of shipments to the market. We expect a similar situation to occur this quarter as well. However, we hope to effectively compete in our business thereafter and see how it unfolds.
Q: How is the software business broken down?
A: Let me explain why NVIDIA has been very successful in software. Accelerated computing is very different from general computing, mainly growing in the cloud. In the cloud, service providers have large engineering teams that work closely with our team to manage, repair, and maintain the complex software stack required for accelerated computing.
Accelerated computing involves specialized software stacks in different areas such as data processing, machine learning, computer vision, speech recognition, large language models, and recommendation systems. NVIDIA has developed hundreds of libraries because software is crucial for opening up new markets and enabling new applications. The necessity of accelerated computing software is a fundamental difference from general computing, which took some time for many to understand.
With the emergence of generative artificial intelligence, every enterprise and software company is now embracing accelerated computing. Unlike large cloud service providers, these companies do not have large engineering teams to maintain and optimize their software stack across various environments. NVIDIA addresses this gap by managing, optimizing, patching, and tuning its software stack and containerizing it into what we call Nvidia AI Enterprise. This solution acts as a runtime, similar to an artificial intelligence operating system, and our charge is $4,500 per GPU per year.
We expect every enterprise and software company deploying applications across clouds, private clouds, and on-premises to use Nvidia AI Enterprise, especially with our GPUs. This plan has had a good start, achieving a billion-dollar run rate, and we are just getting started.
Q: How do you manage product allocation based on customer deployment readiness? How do you monitor whether there is a backlog of products that have not been activated? In the competitive landscape across industries, small startups, healthcare entities, and government, how do you ensure fair product distribution?
A: Firstly, our CSPs have a clear view of our product roadmap and transformation. This transparency gives them confidence to know where products stand and when they will be placed. They understand the timing, quantity, and our allocation process. We strive for fair distribution, doing our best to avoid unnecessary allocations.As mentioned earlier, it is inefficient to allocate resources when the data center is not ready, leading to resource idleness. Our goal is to distribute resources fairly and avoid unnecessary allocations.
We have an excellent ecosystem, including OEMs, ODMs, CSPs, and important end markets. What sets Nvidia apart is that we not only provide technology but also bring customers to our partners, including CSPs and OEMs. This includes biotech companies, healthcare companies, financial services companies, AI developers, large language model developers, autonomous vehicle companies, robot companies, and more. We are witnessing a surge in robot companies, from warehouse and surgical robots to humanoid robots and agricultural robots.
These startups and large companies span across multiple fields such as healthcare, finance, automotive, working on the Nvidia platform. We directly support them, sometimes by allocating resources to CSPs and introducing customers to CSPs to facilitate connections.
Our ecosystem is indeed vibrant, with the core goal of fairly distributing resources, avoiding waste, and seeking opportunities to connect partners and end users. We have always been looking for these opportunities.
Q: How does the company convert backlog orders into revenue? While product delivery times have been significantly reduced, the total supply of the company has actually decreased slightly when adding inventory to contracts and your prepayment supply.
A: Let me focus on how we view three different aspects of the supplier. First, we strive to ship items to our customers as they enter inventory. The second part is that our procurement commitments have many different components. We manufacture the required components, but we also often procure the capacity or components we may need. The procurement time required for capacity or components varies. Some may last for the next two quarters, while others may last for several years. Third, the same applies to our prepayments. Our prepayments are designed in advance to ensure that our several manufacturing suppliers have the reserve capacity we will need in the future. They are just purchased at different lead times because sometimes we have to buy things with longer delivery times or things that need to be built for us.
Q: How does Nvidia view the long-term availability of its products? Will today's training clusters become tomorrow's inference clusters?
A: The reason we are able to significantly improve performance is that our platform has two characteristics. One is that it is accelerated, and the other is that it is programmable. Nvidia is the only architecture that has been evolving from the beginning. We have been able to support it, optimize our stack, and deploy it to our installed base.
On one hand, we can invent new architectures and technologies, such as Tensor Cores, the Transformer engine of Tensor Cores, and improve new numerical formats and processing structures with different generations of Tensor Cores, while supporting the installed base. Therefore, we adopt all new software algorithms, invest in new inventions of industry models, and run them on our installed base.On the other hand, whenever we encounter something revolutionary, like Transformer, we can create something entirely new, such as the Hopper Transformer engine, and apply it to the future. Therefore, we have the ability to introduce software into existing installations and continuously improve it. As time goes on, our new software continues to enrich our customers' installed base. When it comes to new technologies, we have the revolutionary capability to innovate.