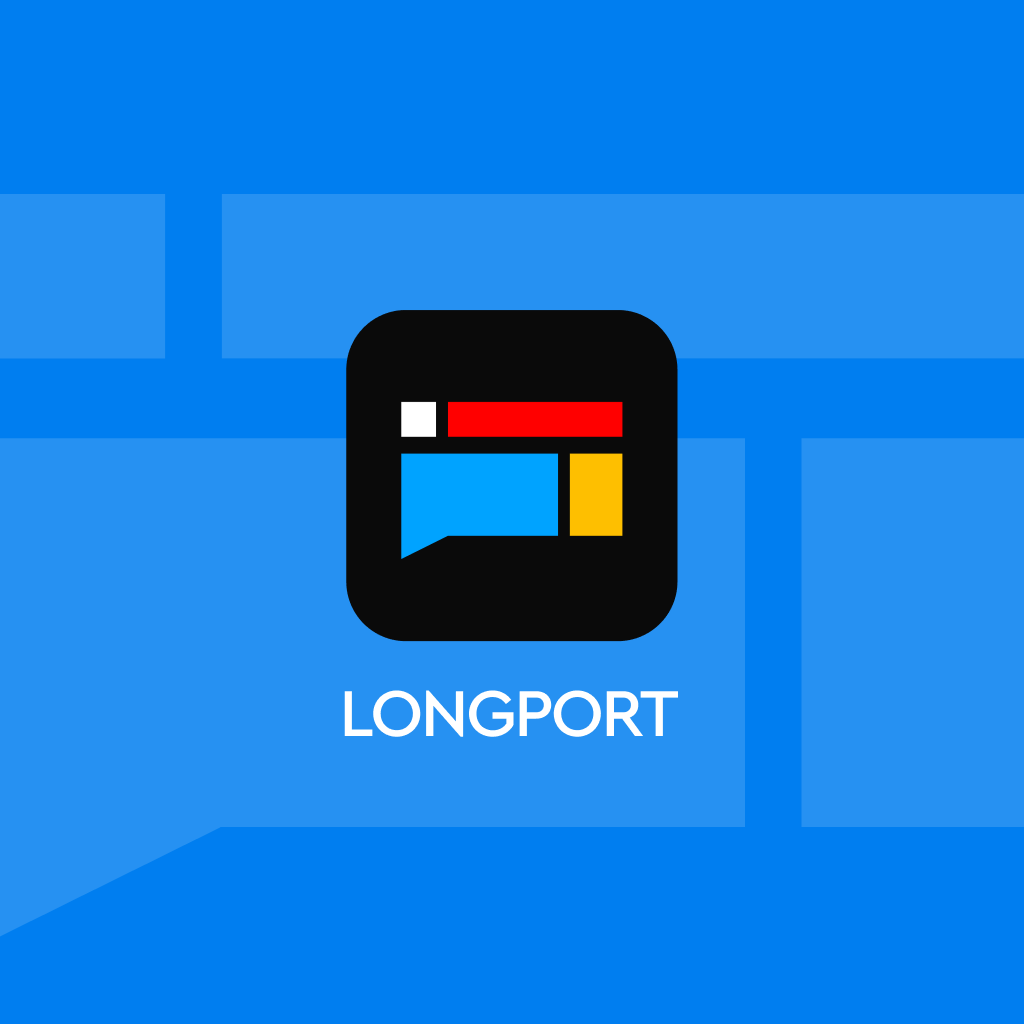
The full text is here! What did Jensen Huang say to save the US stock market?
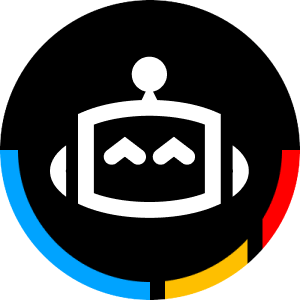
NVIDIA CEO Jensen Huang announced at the Goldman Sachs Technology Conference that NVIDIA will expand its production capacity in the fourth quarter to meet strong demand for Blackwell. He predicted that innovation in the AI computing field will accelerate, with NVIDIA achieving significant performance improvements every two years. Huang's speech boosted the stock price, increasing NVIDIA's market value by $215.8 billion, closing up 8.15%. He also mentioned that future plans include accelerating the construction of data centers to drive enterprise performance improvements and cost savings
On the evening of Wednesday, September 11th, Beijing time, NVIDIA CEO Jensen Huang told Goldman Sachs CEO Solomon in a tech talk organized by Goldman Sachs that NVIDIA will expand its production capacity in the fourth quarter and continue to expand next year.
Huang stated that the demand for Blackwell from customers is enormous, everyone wants to be the first company to have it, everyone wants the most production capacity, and everyone wants to lead. He also specifically mentioned NVIDIA's advantages in algorithm optimization and architectural consistency, which can significantly increase customers' total ownership costs and competitiveness.
The remarks about the huge demand for AI chips stimulated NVIDIA's stock price. In the early trading session, NVIDIA's stock price fell to $107 at one point, but closed at $116.9 following Huang's speech, a surge of 8.15%, adding $215.8 billion in market value, equivalent to RMB 15.369 trillion.
Huang emphasized that with the end of Moore's Law, general computing has reached a bottleneck, and the key in the future lies in accelerating data centers, improving their density and energy efficiency.
He predicted that the global value of general data centers worth trillions of dollars will gradually be replaced by accelerated computing centers. By accelerating core tasks such as SQL processing and recommendation systems, enterprises will achieve significant performance improvements and cost savings.
Future computing will not only be limited to data processing but will also expand into the field of skill enhancement. Huang believes that the application of generative AI technology will change the way we work, and digital assistants and AI tools will become indispensable partners in various fields, further driving productivity improvements in industries.
He also forecasted that the pace of innovation in the AI computing field will continue to accelerate. By developing a variety of chip and technology combinations, NVIDIA will achieve significant performance improvements every two years, maintaining its leadership position in the market.
Below is the complete content of this dialogue, enjoy~
Solomon:
Since you founded NVIDIA in 1993, you have been a pioneer in accelerating computing. The GPU invented by the company in 1999 drove the growth of the PC gaming market, redefined computing, and ignited the modern AI era. Jensen holds a bachelor's degree from Oregon State University and a master's degree from Stanford University.
I want to start from 31 years ago when you founded the company. From a GPU company centered around gaming to now a company providing a wide range of hardware and software services to data centers.
I would like you to talk about the ups and downs of this journey. What were your thoughts when you started, and how did the company evolve along the way? This has been a very extraordinary journey. Perhaps you can also talk about your key priorities looking ahead and the future directionJensen Huang:
I think one thing we did right at the time was that we had a vision, which was to have a new way of computing that could complement the shortcomings of general-purpose computing and solve problems that general-purpose calculators could never solve.
This processor initially started with the extremely difficult task of computer graphics for CPUs, but we knew it would eventually expand to other fields.
The first field we chose was image processing, which is a complement to computer graphics. Then we expanded to physical simulation because in the video game applications we selected, you not only need beautiful images but also dynamic effects to create a virtual world.
We gradually advanced and brought it into the field of scientific computing. Some of our earliest applications were molecular dynamics simulations and seismic processing, which is essentially inverse physics. Seismic processing is very similar to CT reconstruction, both being another form of inverse physics. We progressed step by step in this way.
We thought about adjacent industries and complementary algorithms, gradually solving problems. But the common vision from that time was that accelerating computing could solve interesting problems.
If we could maintain architectural consistency, meaning that software developed today could run on the larger computational foundation you left behind, and past software could be further accelerated through new technologies. This way of thinking about architectural compatibility, starting in 1993, has been our consistent approach to this day.
This is also why NVIDIA's CUDA has such a large installed base. Because we have always protected it, protecting the investments of software developers has always been our company's top priority.
Looking ahead, along the way we have learned a lot, such as how to be a founder, how to be a CEO, how to run a company, how to build a company, and more. These are all new skills. We also learned how to invent the modern computer gaming industry.
Many people don't know that NVIDIA has the largest gaming architecture installed base in the world. GeForce has around 300 million gamers, and its growth momentum is still very strong and vibrant.
So every time we enter a new market, we need to learn new algorithms, understand new market dynamics, and create new ecosystems. We do this because unlike general-purpose computing, if you build an accelerator, things won't operate automatically. As an accelerator, you need to ask yourself: What should be accelerated? Because there is no universal accelerator.
Solomon:
Let's delve deeper and talk about the differences between general-purpose computing and accelerated computing.
Jensen Huang:
If you look at software, from the large software you write, you will find many parts involving file input and output, setting up data structures, and some parts containing magical core algorithms.
These algorithms vary depending on the application field, whether it's computer graphics, image processing, or any other field. It could be fluid dynamics, particle systems, or something like inverse physics that I mentioned, or even something in the field of image processing. These different algorithms are all differentIf you create a processor that excels in these algorithms and can complement the shortcomings of the CPU (what the CPU is good at), theoretically, you can greatly accelerate an application.
The reason is that, typically, 5% to 10% of the code will account for 99.99% of the running time. So if you offload that 5% of the code to our accelerator, theoretically, you can accelerate the application by 100 times. This situation is not uncommon, and we do achieve it quite often.
For example, we accelerated image processing by 500 times. Now we also handle data processing, which is one of my favorite applications because almost everything related to machine learning is data-driven, and data processing is at the core of it.
Whether it's SQL data processing, Spark-like data processing, or vector database processing, all of these involve structured or unstructured data processing, which is the processing of data frames.
We have accelerated these processing tasks significantly, but to do this, you must create the corresponding libraries. For example, in computer graphics, we are fortunate to have graphics libraries like OpenGL and Microsoft's DirectX. But beyond these, there are hardly any ready-made libraries available.
So we created our own libraries, such as one of our most famous libraries, similar to SQL. SQL is a storage computation library, and we created a library called CuDNN, which is the world's first neural network computation library.
We also have CuOpt for combinatorial optimization, CuQuantum for quantum simulation and emulation, and libraries like CuDF for data frame processing (similar to SQL).
All these libraries need to be invented by us. We need to refactor the algorithms in the applications to make them run on our accelerator. If you use these libraries, you can achieve a 100-fold acceleration, or even more.
This concept is very reasonable, but the question is how to invent these algorithms and make the entire video game industry use them? How to make the entire seismic processing and energy industry use them? How to make the entire AI industry use them? Do you understand what I mean?
We must first conduct computer science research, then develop the ecosystem, and convince everyone to use it. At the same time, we must ensure that these libraries can run on all different computers. That's how we are, crossing domains one by one.
We have created rich libraries for autonomous driving cars, excellent libraries for robotics technology development, as well as libraries for virtual screening, whether it is physics-based virtual screening or neural network-based virtual screening. We even have dedicated climate technology libraries.
So we develop field by field, make friends, and create markets. What NVIDIA is truly good at is creating new markets.
We have been doing this for so long, and it seems that accelerated computing is everywhere, but in reality, we are conquering one domain after anotherSolomon:
I know that many investors present are very concerned about the data center market. It would be interesting to hear your views on the long-term and mid-term opportunities for the company. Obviously, the industry you are in is driving the next industrial revolution. What challenges do you think the industry is facing? Let's talk about how you view the development of the data center market.
Jensen Huang:
Two things are happening at the same time, and these two are often confused, we need to discuss them separately. First, let's assume that AI does not exist.
In a world without AI, general computing has reached a bottleneck. Everyone knows that the era of Moore's Law, transistor miniaturization, and performance improvements at equal power or cost has ended.
In the future, we will no longer see CPUs doubling in performance every year. We are fortunate to see performance doubling in 10 years.
In the past, Moore's Law meant a 100-fold performance improvement every 5 years, and a 1000-fold improvement every 10 years. We just had to wait for CPUs to get faster. However, that era has ended, and we are now entering an era of computational expansion.
Solomon:
Now, with the end of Moore's Law, we are experiencing computational expansion.
Jensen Huang:
So what we need to do is accelerate everything as much as possible. Whether it's SQL processing or any form of data processing, especially if you have created an internet company with a recommendation system, it absolutely needs to be accelerated. These systems are now fully accelerated.
A few years ago, they were all running on CPUs, but now, the world's largest recommendation system, this type of data processing engine, has all been accelerated. So, if you have a recommendation system or search system, or any large-scale data processing system, you must accelerate them.
The first thing that will happen next is that the world's multi-trillion-dollar universal data centers will be upgraded to accelerated computing data centers. This will definitely happen. It is inevitable.
One reason is that we have reached a stage where changes must be made. The first dynamic you will see is an increase in computer density. You know, these huge data centers are very inefficient because they are filled with air, and air is a poor conductor of electricity.
What we want to do is compress these large data centers that may consume 50, 100, or 200 megawatts into a very small data center. If you look at some of our server racks, NVIDIA's racks may look expensive, costing millions of dollars per rack, but they can replace thousands of nodes.
Surprisingly, the cost of just connecting cables to old general computing systems may be higher than the cost of replacing these old devices with a high-density rack.
Another benefit of high density is that once you reach this high density, you can do liquid cooling, because cooling a huge data center is difficult, while cooling a small data center is much easier.
So our top priority now is to accelerate and modernize data centers, increase their density, make them more energy-efficient. You can save money, save energy, and greatly improve efficiencyThis is what we are going to focus on for the next 10 years. Now, of course, there is a second dynamic. Due to NVIDIA's accelerated computing bringing huge cost savings, in the past 10 years, computing power has not just increased by 100 times, but by 1 million times.
So, the question becomes: if your speed is increased by a million times, what different things would you do? Suddenly, people say, "Hey, why don't we let the computer write software itself, instead of us trying to determine the functionality or algorithms? We just need to give all the data, all the predictive data to the computer, and let it find the algorithm itself."
We have been operating in such a large-scale different data field that now computers can not only process data but also understand the meaning of data. Because it can understand multiple modes at the same time, it can perform data translation.
We can translate from English to images, images to English, English to proteins, and then proteins to chemical molecules. Therefore, because it can understand all data simultaneously, it can now perform these translation operations we call generative AI.
It can generate small-scale text from large-scale text, or vice versa, now we are entering an era of computational revolution.
It is amazing that the first batch of data centers worth trillions of dollars have already been accelerated. We have invented this new buffering technology in AI, this AI revolution is not just a tool, but also a skill.
That is why a whole new industry is being created now. Because, if you look back at the entire IT industry, until now, we have been creating tools and instruments that people can use. But this time, what we are going to create is the enhancement of human skills.
That is why people believe that AI will not only be limited to data centers worth trillions of dollars but will also expand into the field of skills.
So, what are these skills? First is digital skills, such as a digital assembly line robot, or a digital customer service robot, or even a digital visual employee dedicated to planning and business strategy.
It could also be a digital SAP agent. Our company uses a lot of ServiceNow services, and we even have digital employee services. So now we have these digital "humans," this is how AI works.
Solomon:
Let's take a step back and look at it from a different perspective. Based on all that you just mentioned, there is an ongoing debate in the financial markets about whether we can achieve sufficient ROI when building AI infrastructure.
How do you assess the return on investment for clients in the current cycle? If we look back at cloud computing, how did the return on investment perform in similar stages of adoption? How does our current position compare to that time?
Jensen Huang:
Before cloud computing, virtualization was the main trend, remember? Virtualization basically virtualized all the hardware in the data center into a virtual data centerThen we can move workloads across the entire data center rather than directly associating them with specific computers. As a result, the utilization of the data center has been improved.
Through virtualization, the cost of the data center has been reduced by half or more. Building on this, we push these virtual machines to the cloud, where multiple companies can share the same resources, further increasing utilization.
Over the past 10 to 15 years, the development of virtualization and cloud computing has masked a fundamental change happening at the bottom— the end of Moore's Law. Virtualization and cloud computing have brought significant cost savings, but they have masked the end of transistor scaling and CPU performance growth.
Now, as the effects of these cost savings gradually diminish, what we see is the expansion of data centers and computing. Therefore, the first thing that happens is accelerated computing.
Today, you can use NVIDIA accelerators for data processing in the cloud, such as Spark, which is one of the most commonly used data processing engines in the world today. If you use Spark and NVIDIA accelerators in the cloud, you can typically see a 20x acceleration effect.
So, you can save a lot of computing time, although the computing costs may slightly increase, the overall return on investment is very substantial. This is the direct ROI brought by acceleration.
Next is the first wave of AI generation. In this stage, infrastructure providers like us and all cloud service providers deploy infrastructure to the cloud, allowing developers to use these machines to train models or fine-tune models.
This has brought very good returns because the demand is very strong. For every dollar spent, there is a fivefold return. This situation is happening globally.
Some applications we are familiar with, such as OpenAI's ChatGPT, or tools like Github Copilot, bring amazing productivity improvements.
Today, almost all of our software engineers are using these generation tools, whether it's our own developed tools or collaborative generation using C++ and CUDA.
In the future, every software engineer will have a digital engineer as an assistant, working 24/7 to assist them. This is the trend of the future.
Our company has 32,000 employees, but we hope to increase this number by 100 times through digital engineers. Many industries are actively embracing this trend.
In our company, AI has become an important tool in computer graphics. We now calculate one pixel and infer the remaining 32 pixels to complete image generation, which can significantly save energy and computing time.
Without AI, we cannot support the autonomous driving industry, nor can we complete research in robotics and digital biology. Almost all tech bio companies are using AI for data processing, even for protein generation and virtual screening. The entire new drug discovery process has been reinvented because of AI, which is very excitingSolomon:
Now, let's talk about your competitive advantage. Obviously, there are some public and private companies trying to challenge your leadership position. How do you view your competitive barriers?
Jensen Huang:
First of all, there are a few things that set us apart. First, remember that AI is not just about hardware, AI is more about infrastructure. Today's computers are not just about manufacturing chips and then selling them.
Building an AI computer is not just about assembling chips, but building a complete data center. For example, our Blackwell system, which consists of seven different types of chips, with Blackwell being just one of them.
Solomon:
Yes, tell us more about Blackwell.
Jensen Huang:
When you want to build an AI computer, people may mention terms like "supercluster" or "supercomputer" because it's not just about chips or a single computer, but the construction of an entire data center.
If you look at these superclusters, imagine the software needed to run them. There is no Microsoft Windows to run these systems, each system's software is completely customized.
Companies that design chips also design this supercomputer and all its software. Therefore, having a fully optimized, more efficient, and energy-saving system makes sense.
Secondly, AI involves algorithms, and we are very good at understanding the requirements of algorithms and how to distribute computing tasks among millions of processors to ensure stable operation for a long time, achieving high energy efficiency and fast task completion. This is an area we excel in.
Finally, AI is about computation, and the key to computation is installing the foundation. Having the same architecture, whether in the cloud or on-premises, whether running on supercomputers, robots, or personal computers, having a unified architecture that can run the same software is very important.
This consistency is the principle we have adhered to for the past 30 years, and it is also why if you were to start a company today, the most obvious choice would be to use NVIDIA's architecture.
Our architecture is everywhere, no matter what computing device you choose, as long as it has "NVIDIA Inside," you know it can run the software you need.
Solomon:
Your innovation speed is very fast, I would like you to talk about Blackwell. Its training speed has increased fourfold, and its inference speed is 30 times faster than the previous generation Hopper. You seem to be innovating at an amazing speed, do you think you can maintain this fast pace? When you consider your partners, how do they keep up with your rapid pace of innovation?
Jensen Huang:
Our innovation pace is based on a fundamental approach: we develop seven different chips each time. The update cycle for each chip is about two years, and we can give them a mid-term boost each year.
But if you introduce a completely new architecture every two years, it's like moving at the speed of light. We have seven different chips, all contributing to performanceTherefore, we can introduce a better AI cluster or supercluster than the previous generation every year because we have many different components that can be optimized.
This scale of performance improvement directly translates into the total cost of ownership (TCO) for customers. For example, Blackwell's performance is three times that of the previous generation products. If a customer has a power budget of 1 gigawatt, their revenue will also triple.
This performance improvement translates into throughput, and throughput translates into revenue. For customers with a fixed power budget, this means a threefold increase in revenue. No other cost-saving measures can compensate for this revenue growth.
Therefore, by integrating all these different components and optimizing the entire stack and cluster, we can provide higher value to customers.
Similarly, for any amount customers want to spend, performance improvement means cost reduction. We have the best performance per watt (i.e., revenue) and the best total cost of ownership (TCO), which means better gross margins.
We continue to drive these innovations in the market, allowing customers to benefit continuously. And because our architecture is compatible, software developed yesterday can still run tomorrow, and software developed today can run across the entire installed base, allowing us to progress very quickly.
If we were to change architectures each time, this speed would be impossible to achieve. Building a system itself takes a year. The reason we can move so fast is because we integrate all components together.
Someone once tweeted that within 19 days of our shipment, they had already put a supercluster online and running. It is impossible to piece together such an efficient system in a year.
Therefore, I believe that we are converting the speed of innovation into customer revenue growth and margin improvement, which is truly remarkable.
Solomon:
Most of your supply chain partners are in Asia, especially in Taiwan. How do you view this situation in the current geopolitical context?
Jensen Huang:
The supply chain in Asia is indeed very extensive and intertwined. People often think that when we talk about GPUs, it's just a small chip. In fact, NVIDIA's system has 35,000 parts, weighs 80 pounds, and consumes 10,000 amps of current.
Once installed, the entire system weighs 3,000 pounds. This GPU system is very complex, building it is like making an electric car. We have designed as much diversity and redundancy as possible to ensure the stability of the supply chain.
When necessary, we have enough intellectual property to switch flexibly between different supply chains. Perhaps some production technologies are not the best choice, and performance and cost cannot be maintained at the same level, but we can still provide viable solutions. If any unexpected situation occurs, we can quickly adjust the supply chain.
We cooperate with TSMC because it is the best in the world, not just excellent, but absolutely leading. It has a long history of cooperation, high flexibility, and scalabilityLast year, NVIDIA's revenue experienced explosive growth, thanks in large part to the support of its supply chain. TSMC's and the supply chain's rapid response capabilities are incredible.
In less than a year, we have significantly increased production capacity, and we will continue to expand next year. This agility and responsiveness are the reasons we chose TSMC. However, if necessary, we can certainly choose other suppliers.
Solomon:
Yes, the company is indeed in a very favorable position, and we have discussed many good things. So, what are you most worried about?
Jensen Huang:
Well, our company is currently collaborating with all the data centers in the world. I can't think of a data center, cloud service provider, or computer manufacturer that we are not working with right now.
So, this means we have a huge responsibility. Many people rely on us, and there are high expectations for us. The demand is very strong, and delivering our components, technology, infrastructure, and software is emotionally very important to many people. Because it directly affects their income and competitiveness.
Therefore, today we may have more emotionally invested customers who really need our products, and emotions are running high.
We can feel that everyone is waiting for us to meet their needs, and once we deliver these products, this emotion will disappear. But the current emotions are very intense, and the pressure is high.
We have a great responsibility and are striving to do our best. We are fully committed to driving the production of Blackwell, which is now in full production.
We will ship in the fourth quarter and expand production capacity in the fourth quarter, continuing to expand next year. The demand for Blackwell is just too high, everyone wants to be the first company to have it, everyone wants the most production capacity, everyone wants to be ahead.
Therefore, this sense of urgency is really strong. I think it's very interesting to invent the next computing era, it's exciting to see these amazing applications being created, it's exciting to see robots walking around, and it's amazing to see these visual agents working as a team on your computer to solve problems.
At the same time, we design chips with AI, and then run our AI on these chips, which is all very impressive. But the truly tense part is that we bear the expectations of the world.
Translated from: Newin, original title: "In-depth | NVIDIA Soars 8%! Jensen Huang Tells Goldman Sachs CEO - The Demand for AI Chips is Just Too High! Every Customer Wants Them, Wants to Get Stronger"