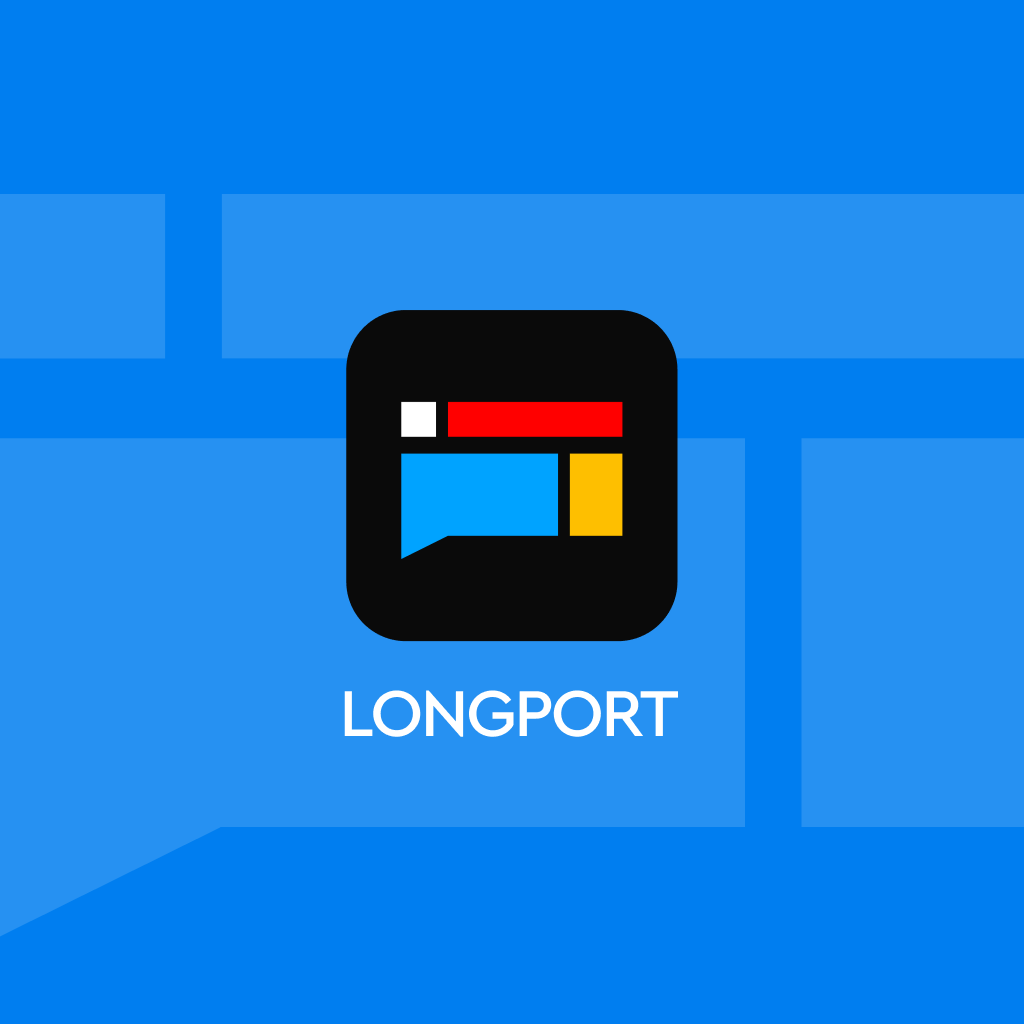
The major debate affecting NVIDIA's fundamental logic: What does OpenAI's change in strategy mean? Who is lying?
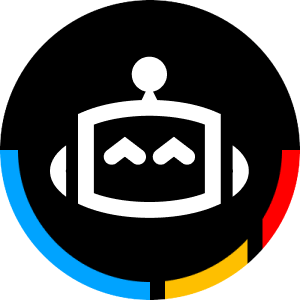
So far, the AI hardware market has been primarily dominated by the unlimited demand for NVIDIA training chips. However, if better results can be achieved by increasing training time and testing (inference) time, market demand may shift from large-scale pre-training clusters to inference clouds, where NVIDIA may face more competition in the inference chip market
Is the "Scaling Law" of large model pre-training failing? Model inference becomes the "antidote," and will NVIDIA's dominance change?
Under the guidance of the "Scaling Law," AI large model pre-training is currently facing bottlenecks. According to a report by Reuters on December 12, major AI labs in Silicon Valley are experiencing widespread difficulties in their new model training plans, with delays and disappointing results. Compared to GPT-4o, Orion reportedly shows almost no improvements, and Google's Gemini 2.0 faces similar issues.
To overcome these bottlenecks, OpenAI is exploring "test-time computation" technology, allowing models to think through problems (inference) using a multi-step approach rather than being limited to pre-training, thereby enhancing performance. Reports indicate that the application of this technology will ultimately drive OpenAI to release the o1 model.
This could change the competitive landscape of AI hardware.
So far, the AI hardware market has been dominated by the unlimited demand for NVIDIA's training chips. However, if better results can be achieved by increasing training and testing (inference) time, then the next generation of models may not require such large parameters, and smaller models will directly reduce costs. Market demand may shift from large-scale pre-training clusters to inference clouds, and NVIDIA may face more competition in the inference chip market.
Challenges to the "Scaling Law"
Major AI labs in Silicon Valley are currently experiencing widespread difficulties in new model training.
According to technology media The Information, OpenAI's next flagship model "Orion" has completed 20% of its training. Although its performance is close to the existing GPT-4, the degree of improvement is far less than the leaps between the previous two flagship models.
Orion performs better on language tasks but may not outperform previous models on tasks like coding. Moreover, compared to other recently released models, the cost of running Orion in OpenAI's data center may be higher.
The slowdown in Orion's progress directly challenges the "Scaling Law" that has long been upheld in the field of artificial intelligence, which states that model performance will continue to improve significantly with increasing data volume and computational resources.
Ilya Sutskever, the former co-founder of OpenAI who was among the first to apply the "Scaling Law" in practice and ultimately succeeded in creating ChatGPT, candidly stated in an interview with Reuters that the results of AI model scaling training have stabilized. The phase of explosive performance improvement in AI models through the use of more data and computing power in pre-training may have come to an end.
The 2010s were the era of the "scaling law," and now we have returned to an era of miracles and discoveries. Everyone is looking for the next miracle.
What is important now is to "scale the right things."
Ilya revealed that his team is researching a brand new alternative method to scale pre-training.
Will "training runs" be the breakthrough method?
OpenAI has strongly denied that AI model training is facing bottlenecks. In response, OpenAI researchers are exploring a technique called "training runs." A dozen AI scientists, researchers, and investors told Reuters that they believe these technologies have driven OpenAI to release the o1 model.
The so-called training runs technology refers to enhancing the model during the inference phase (when the model is being used), allowing the model to generate and evaluate multiple possibilities in real-time, rather than understanding and selecting a single answer to ultimately choose the best path.
This approach allows the model to allocate more processing power to challenging tasks such as mathematics and coding problems, as well as complex operations that require human-like reasoning and decision-making.
With the application of new technology, o1 undergoes another round of training based on foundational models like GPT-4. The model is no longer limited by pre-training and can think through problems using a multi-step approach (similar to human reasoning) to enhance performance. o1 also incorporates data and feedback curated from PhDs and industry experts.
An OpenAI researcher involved in the development of o1 candidly stated at last month's TEDAI conference:
Allowing AI to think for 20 seconds with a hand of poker yields performance improvements equivalent to scaling the model 100,000 times and extending the training time 100,000 times.
Is NVIDIA's monopoly position about to be broken?
Other tech giants are also actively following suit.
Reuters cited multiple insiders reporting that researchers from other top AI labs, such as Anthropic, xAI, and Google DeepMind, are also developing their own versions of the "training runs" technology.
This could change the competitive landscape of AI hardware.
So far, the AI hardware market has been dominated by the insatiable demand for NVIDIA's training chips. However, if better results can be achieved by increasing training and testing (inference) time, then the next generation of models may not require such large parameters, and smaller models will directly reduce costs.
From Sequoia Capital to Andreessen Horowitz, well-known venture capital firms have invested billions of dollars to fund the expensive AI model development of multiple AI labs like OpenAI and xAI, and they are closely monitoring this shift and weighing the impact of their costly bets.
Sequoia Capital partner Sonya Huang told Reuters:
This shift will take us from a world of large-scale pre-training clusters to inference clouds, which are distributed cloud servers used for inference.This may break NVIDIA's monopoly in the training chip field, and the company may face more competition in the inference chip market. Inference chip companies like Groq may be able to "tear open" industry gaps.
NVIDIA also candidly acknowledged the changes in demand for inference chips. In a speech in India last month, Jensen Huang talked about the increasing demand for chips for inference due to new technologies, emphasizing the importance of the technology behind the o1 model:
We have now discovered the second "scaling law," which is the "scaling law" for inference... All these factors have led to very high demand for Blackwell