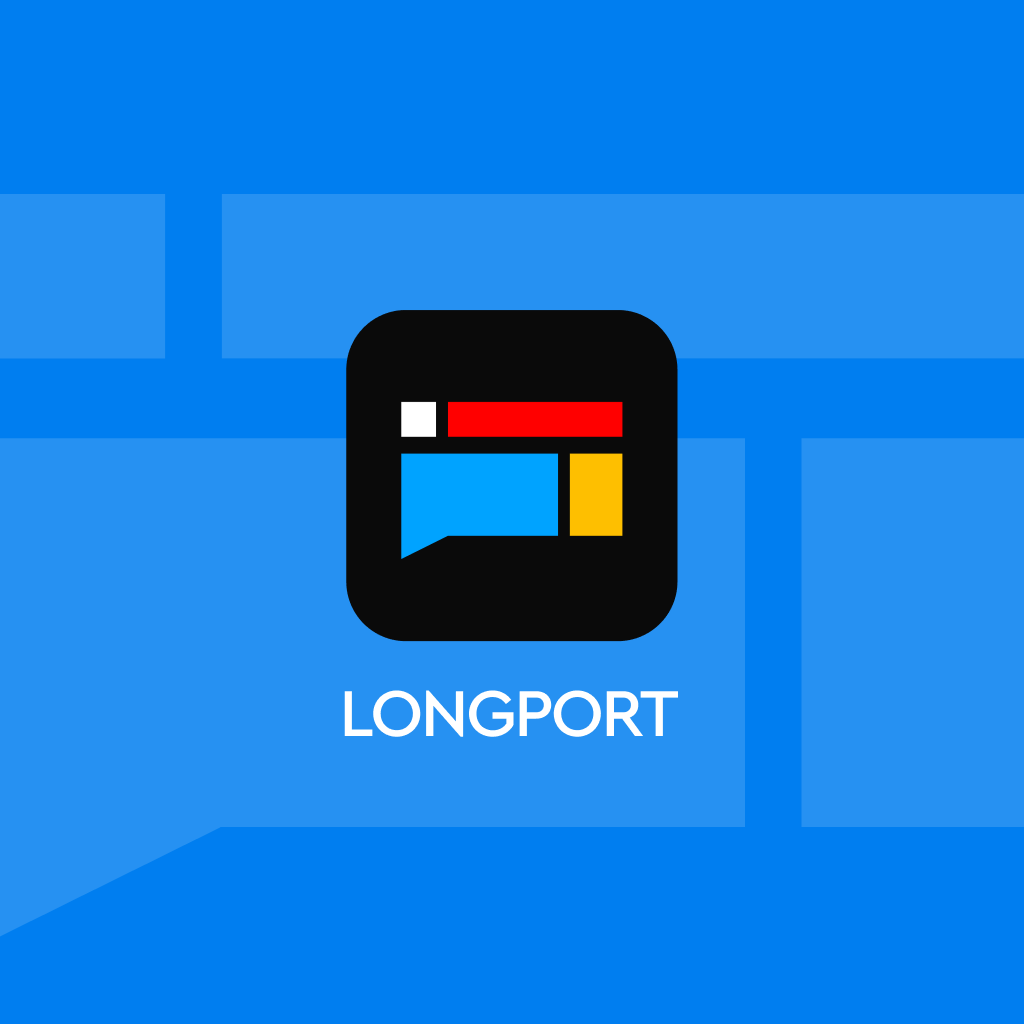


The First Wave of Artificial Intelligence (NVIDIA 3QFY24 Earnings Call)
NVIDIA (NVDA.O) released its fiscal third-quarter earnings report for the 2024 fiscal year (ending in October 2023) after the US stock market closed on November 22nd. The key points from the conference call are as follows:
1. Key information from the NVIDIA conference call:
1) Visibility of revenue from the data center business: It is expected to continue growing until 2025.
2) The wave of generative AI: Shifting from start-ups and communication service providers to consumer internet companies, enterprise software platforms, and enterprise companies.
3) Impact of US restriction policies: Sales to China and other affected destinations that require compliance with licensing requirements currently contribute approximately 20% to 25% of data center revenue.
2. $NVIDIA(NVDA.US) Conference call transcript
2.1 Management statement
The third quarter was another record-breaking quarter. Revenue reached $18.1 billion, a 34% increase MoM and over 200% increase YoY.
This significantly exceeded our expectation of $16 billion. Starting from the data center, the continuous growth of the NVIDIA HGX platform based on the Hopper Tensor Core GPU architecture, as well as InfiniBand and networking, drove data center revenue to a record-breaking $14.5 billion, a 41% increase MoM and a 279% increase YoY. NVIDIA HGX and InfiniBand are essentially reference architectures for AI supercomputers and data center infrastructure. Some of the most exciting generative AI applications, including Adobe Firefly, ChatGPT, Microsoft 365 Copilot, and Zoom AI Companion, are built and run on NVIDIA.
Our data center computing revenue has quadrupled compared to last year, and networking revenue has almost doubled. Investments in infrastructure for training and inference of large language models, deep learning, recommendation systems, and generative AI applications are driving strong demand for NVIDIA's accelerated computing. Inference is now the primary workload for NVIDIA AI computing.
Consumer internet companies and enterprises drove astonishing MoM growth in the third quarter, accounting for about half of our data center revenue, surpassing overall growth.Adobe, Databricks, Snowflake, and ServiceNow, among other enterprise software companies, are adding AI co-pilots and assistants to their platforms.
In this quarter, the other half of our data center revenue comes from the strong demand for all hyperscale CSPs and a range of new GPU-dedicated CSPs worldwide. These CSPs are growing rapidly to meet the new market opportunities in the AI field. The demand for NVIDIA H100 Tensor Core GPU instances is significant, and they are now available in almost every cloud with instances. We have significantly increased supply every quarter this year to meet the strong demand, and we expect to continue doing so next year.
We will also have a broader and faster product release cadence to meet the growing and diverse AI opportunities. At the end of this quarter, the US government announced a set of new export control regulations targeting China and other markets, including Vietnam and certain Middle Eastern countries. These regulations require many of our products, including our Hopper and Ampere 100 and 800 series, to obtain export licenses. In the past few quarters, our sales to China and other affected destinations, which currently need to comply with licensing requirements, have contributed approximately 20% to 25% of our data center revenue.
Our training clusters now include over 10,000 H100 GPUs, which is three times the number from six months ago, reflecting highly efficient scaling. Efficient scaling is a key requirement for generating artificial intelligence as the number of law school graduates increases by an order of magnitude each year. Microsoft Azure has achieved similar results on almost the same cluster, demonstrating the efficiency of NVIDIA AI in public cloud deployments. Currently, the annualized revenue run rate for our networking business has exceeded $1 billion.
Our growth is driven by the specific demand for InfiniBand, which has grown fivefold. InfiniBand is crucial for achieving the scale and performance required for training LLM. Microsoft explicitly highlighted this last week, emphasizing that Azure uses over 29,000 miles of InfiniBand lines, enough to circle the Earth. We are extending NVIDIA networking into the Ethernet domain. Our brand-new Spectrum-X end-to-end Ethernet product, built specifically for AI, will be launched in the first quarter of next year and is supported by leading OEMs such as Dell, HPE, and Lenovo.
Compared to traditional Ethernet products, Spectrum-X can achieve a 1.6x improvement in AI communication network performance. I would also like to provide an update on our software and services products, as we are starting to see adoption of these products. We expect to end this year with $1 billion in annual recurring revenue from our software support and service products. We see two major opportunities for mid-term growth, namely our DGX cloud services and our NVIDIA AI enterprise software.Game revenue reached $2.86 billion, with a MoM growth of 15% and a YoY growth of over 80%. The demand for back-to-school shopping season is strong, and NVIDIA RTX ray tracing and AI technology are now priced as low as $299. We have brought the best lineup ever for gamers and creators. Even in the context of a sluggish PC market, the number of games has doubled compared to before the COVID-19 pandemic.
ProViz revenue was $416 million, with a MoM growth of 10% and a YoY growth of 108%. NVIDIA RTX is the preferred workstation platform for professional design, engineering, and simulation use cases, while artificial intelligence is becoming a strong demand driver. Early applications include IMP for AI imaging in healthcare and edge AI in smart spaces and public sectors. We have launched a new desktop workstation series based on NVIDIA RTX, Ada Lovelace, Generation GPU, ConnectX, and SmartNIC, with AI processing, ray tracing, and graphics performance twice that of previous generations.
We announced two new Omniverse cloud services for automotive digitization on Microsoft Azure: the virtual factory simulation engine and the autonomous driving car simulation engine. We are turning to the automotive field. Revenue was $261 million, with a MoM growth of 3% and a YoY growth of 4%, mainly driven by the continuous growth of the NVIDIA DRIVE Orin SoC-based autonomous driving platform and the increasing adoption of AI cockpit solutions by global OEM customers. We have expanded our automotive partnership with Fuzikang and incorporated our next-generation automotive SoC, NVIDIA Drive Thor.
Fuzikang has become an ODM for electric vehicles. Our partnership provides Fuzikang with standard AV sensors and computing platforms to easily build state-of-the-art, secure software-defined cars. Now, we will expand the GAAP gross margin to 74% and the non-GAAP gross margin to 75%, thanks to the increase in data center sales and the reduction in net inventory reserves, including a 1 percentage point release of previously reserved inventory related to Ampere GPU architecture products.
Operating expenses increased by 12%, and non-GAAP operating expenses increased by 10%, mainly reflecting the increase in compensation and benefits.
FY2Q24 Guidance:
Total revenue is expected to be $20 billion, with a fluctuation of 2%. We expect data centers to drive strong continuous growth, as well as sustained strong demand for computing and networking. Game revenue may decline continuously as it now aligns more with the seasonal nature of laptops.GAAP and non-GAAP gross margins are expected to be 74.5% and 75.5%, respectively, with a fluctuation of 50 basis points. GAAP and non-GAAP operating expenses are expected to be approximately $3.17 billion and $2.2 billion, respectively.
GAAP and non-GAAP other income and expenses are expected to be approximately $200 million, excluding gains or losses from non-affiliated investments. GAAP and non-GAAP tax rates are expected to be 15%, with a fluctuation of 1% (excluding any discrete items).
2.2 Q&A
Q1: In terms of shipment volume in the generative AI market, what position are we currently in? Because when I look at the trajectory in your data center, the central expenses will be close to 30% next year. So, what indicators are you focusing on? Where do we stand in the AI market?
A1: Historically, over the past few quarters, China and some other affected destinations accounted for about 20% to 25% of our data center revenue. We expect that as we enter the fourth quarter, this number will decrease significantly.
Export controls will have a negative impact on our business in China, and even in the long run, we cannot clearly understand the severity of this impact. However, we are working to expand our data center product portfolio to potentially offer new compliant solutions that do not require a license.
These products may be launched in the coming months. However, we expect their contribution to fourth-quarter revenue to be relatively small or insignificant.
Generative AI is the largest software and hardware TAM expansion we have seen in decades. At its core, it is primarily based on retrieval-based methods, where almost everything you do is retrieved from some storage. Now, it has been enhanced, adding generative methods, and it has almost changed everything.
You can see text-to-text, text-to-image, text-to-video, text-to-3D, text-to-protein, text-to-chemicals. These are things that used to be handled and input by humans, but now they are generative methods. The way we access data has changed. It used to be based on explicit queries.
Therefore, we are very excited about the work we are doing with SAP, Dropbox, and many other partners you will hear about. One of the truly impactful areas is the software industry, which is valued at about $1 trillion and has been building tools for manual use for decades. Now, a new software component has emerged, called co-pilot and assistant.
These tools do not require manual use, but have co-pilots to assist you in using them. So, of course, we will continue to do this, not just licensed software, but we will also hire co-pilots and assistants to help us use the software. We will connect all these co-pilots and assistants to the AI team, which will be a modern version of software, a modern version of enterprise software. Therefore, the transformation of software and the way software is completed is driving the underlying hardware.Now we have a better method called accelerated computing, which can save an order of magnitude of energy, an order of magnitude of time, or an order of magnitude of cost. Therefore, if you are willing, accelerated computing is transforming general computing into this new method. The new data center further enhances this point. This is the traditional data center you just mentioned, of which we occupy about one-third.
But there is a new type of data center, which is different from the past data centers. The past data centers run a large number of applications used by different tenants who share the same infrastructure, and the data center stores a large number of files. These new data centers have few applications, and even if there is an application, it is basically used by one tenant. It processes data, trains models, generates tokens, and generates artificial intelligence. We call these new data centers artificial intelligence factories. We see that almost every country is building artificial intelligence factories.
Therefore, if you look at where we are in terms of expansion and transition to this new computing method, this is the first wave you see in large-scale language model startups, artificial intelligence generation startups, and consumer internet companies. We are making great efforts. At the same time, as this situation continues to increase, you will see that we are starting to cooperate with enterprise software companies who want to build chatbots and co-pilots and provide assistance to enhance their tools on their platforms. You will see the emergence of CSPs specializing in GPUs around the world, who are truly dedicated to one thing, which is processing artificial intelligence. You will see that sovereign artificial intelligence infrastructure, individuals, and countries now realize that they must leverage their technology. Owning their own data, retaining their own data, retaining their own culture, processing this data, and developing their own artificial intelligence, you can see this in India.
Therefore, I believe that as the generative artificial intelligence wave spreads in every industry, every company, and every region, you will see new developments. So, we are at the beginning of this turning point, this computing transformation.
Q2: I would like to ask about the evolution of Ethernet in the business network aspect.
A2: Our network business has exceeded $1 billion and will continue to expand. We recently added a new network platform to our network business. However, the vast majority of dedicated large-scale AI factories are standardized on InfiniBand. The reason is not only because of its data rate and latency, but also because of the way it moves traffic in the network, which is very important.
With InfiniBand and software-defined networking, we can achieve control, adaptive routing, performance isolation, and noise isolation. Not to mention the data rate, low latency, and very low cost of InfiniBand, which are natural parts of InfiniBand. Therefore, InfiniBand is not just a network, it is also a computing structure. We have added many software-defined functions, including computing, to the structure.For example, we recently talked about one of the models we are creating, called Shipnemo, and we are building many other models. We will create tens or hundreds of custom AI models within the company.
What we have done is invent this new platform that extends Ethernet without replacing it. It is 100% compatible with Ethernet and optimized for east-west traffic (the location of the computing structure). It allows us to perform some functions of InfiniBand (not all, but some) through BlueField's end-to-end solution and our Spectrum switch added to the Ethernet network, and we have achieved excellent results. Our market entry strategy is to enter the market with large enterprise partners who already provide our computing solutions. Therefore, HP, Dell, and Lenovo have integrated NVIDIA AI stack and NVIDIA AI enterprise software stack, and now they bundle and sell their Spectrum switches.
With their large sales teams and extensive distributor networks, they will be able to provide fully integrated (if you wish) AI solutions that are at least end-to-end optimized for enterprise clients worldwide.
Q3: I would like to know if you can talk more about Grace Hopper, how you view the use of certain microprocessors as TAN expanders, and what applications you think using Grace Hopper has compared to more traditional H100 applications?
A3: Grace Hopper is already in production and is currently being mass-produced. We expect that next year, with all the design victories we have achieved in high-performance computing and AI infrastructure, we will rapidly evolve from our first data center CPU to a billion-dollar product line. This will be a very large product line for us. It can create computing nodes with both fast memory and large memory. In the field of vector databases or semantic search, known as RAG (Retrieval-Augmented Generation), this allows you to reference proprietary data or factual data before generating responses in generative AI models.
This way, the generative model can still interact with you naturally while referencing factual data, proprietary data, or domain-specific data, your data, and can relate to the context and reduce hallucinations. For example, this is a great use case for Grace Hopper. It also serves customers who really want a CPU different from x86. It could be the European Supercomputing Center or European companies wanting to establish their own ARM ecosystem and build the entire stack, or CSPs deciding to switch to ARM because their custom CPU is based on ARM.Q4: I want to ask about the visibility of your revenue. Do you think the Data Center can grow until 2025?
A4: I absolutely believe that the Data Center can achieve growth before 2025. There are several reasons for this. We are significantly expanding our supply. We already have one of the world's widest, largest, and most powerful supply chains. People think that GPUs are just a chip, but the HGX H100 (Hopper HGX) has 35,000 components. It weighs 70 pounds. Eight of those chips are Hopper's. It is a supercomputer, so the only way to test a supercomputer is to use another supercomputer. Therefore, every aspect of our HGX supply chain is complex, and the team we have here has truly expanded that supply chain in an incredible way. Not to mention, all of our HGXs are connected to the NVIDIA network, and the complexity of the network, transceivers, NICs, cables, switches is incredible.
As I mentioned before, we have new customers. Different regions are establishing GPU expert clouds, sovereign AI clouds from around the world, because they realize that they cannot afford the cost of giving their country's knowledge and national culture to others and then reselling AI to them. They must, they should, they have the skills, of course, combine with us, and we can help them do this, establish their national AI, so the first thing they need to do is create their AI cloud, national AI cloud. You also see that we are now growing into enterprises.
There are two paths in the enterprise market. The first path, of course, is ready-made AI, of course, there is ChatGPT, incredible ready-made AI and others. There is also proprietary AI because software companies like ServiceNow and SAP, as well as many other companies, cannot afford the cost of outsourcing their company's intelligence to others.
We have a new service called AI Foundry, where we leverage NVIDIA's capabilities to provide services to them. The next one is enterprises building their own custom AI, their own custom chatbots, their own custom rules. And this capability is spreading worldwide. The way we serve this market is by using the entire system stack, including our computing, networking, and switches, running the software stack we call NVIDIA AI Enterprise, and acquiring this software stack through our market partners such as HP, Dell, Lenovo, and so on.
Therefore, we see the wave of generative AI shifting from startups and communication service providers to consumer internet companies, to enterprise software platforms, to enterprise companies. Ultimately, you see one of the areas where we are investing a lot of effort is related to industrial generative AI, which is the combination of NVIDIA AI and NVIDIA Omniverse, and this is a very, very exciting work.Q5: You mentioned that you will be launching regulatory-compliant products in the coming months, but their contribution to fourth-quarter revenue is expected to be relatively limited. Is this a timing issue? Will it become a source of reacceleration and growth in data center revenue from October onwards? Or should we expect a relatively limited contribution to future revenue due to pricing? How will the AI Foundry service, announced last week, operate in terms of profitability? Will it mainly generate revenue from services and software? How should we consider long-term opportunities? Will this be exclusive to Microsoft or do you have plans to expand to other partners as well?
A5: Regarding the potential new products we can offer to Chinese customers, the design and development of these new products are crucial processes. As we have discussed, we will ensure that we also have thorough discussions with the US government regarding our intentions for these products. Given the current quarter's situation, several weeks have already passed, and we need some time to carefully study and discuss with customers their needs and desires for these new products we possess. Looking ahead, whether in the medium or long term, it is difficult to say what we can produce in collaboration with the US government and what the interests of our Chinese customers are. Therefore, we are still focused on finding the right balance for Chinese customers, but it is currently difficult to determine.
AI Foundry presents significant opportunities and holds great significance. Firstly, every company has its own core intelligence, which constitutes our company. Our data, our domain expertise. For many companies, we create tools, and most software companies in the world are tool platforms that people use today. In the future, these AI-enhanced people will use these AI platforms we hire. These AI platforms must go global, and you will see that we have already announced some, such as SAP, ServiceNow, Dropbox, Getty, and many other platforms that will be launched soon. This is because they have their own proprietary AI, and they want their own proprietary AI. They cannot afford the cost of outsourcing intelligence and distributing data, nor can they hand over the reins to other companies to build AI for them.
We have a few things that are very important for foundries, just like TSMC is a foundry. You must have AI technology. As you know, we have incredible depth of AI capability, AI technology capability. Secondly, you must have best practices, known practices, skills in inventing AI models to handle data, to create AI with guardrails, fine-tuning, and so on. The third thing is you need a foundry, which is the DGX Cloud. Our AI models, called AI foundations, run on the DGX Cloud, if you will, the CAD systems we use to create AI, called NEMO.We have a large installation base in the cloud, on-premises, and anywhere else. It is secure, securely patched, constantly patched, optimized, and supported. We call it NVIDIA AI Enterprise. The price of NVIDIA AI Enterprise is $4,500 per GPU per year. That's our business model. Our business model is essentially a license. Then, our customers can build their profit models on top of this basic license. In many ways, wholesale has become retail.
They can have a subscription license basis for each instance or a subscription based on usage. They can create their own business models in many different ways, but our approach is basically like a software license, like an operating system. So, our business model is to help you create custom models and then run these custom models on NVIDIA AI Enterprise.
Q6: I want to know if the guidance for the fourth quarter would be higher without the restrictions in China? Or is your supply restricted, forcing you to redirect goods that could have been shipped to other parts of China? Along these lines, if you could let us know the current delivery time in the data center and if the current situation would reduce these delivery times because you have some parts available for immediate shipment?
A6: Yes, in some cases, we are constantly working to improve our supply each quarter. We have been very solid in terms of growth each quarter, which determines our revenue. But since we don't have China in our outlook for the fourth quarter, we are still working to improve our supply and plan to continue growing next year and make efforts for that.
Q7: Perhaps you can take some time to discuss the evolution of large models in inference and how your company positions itself in this area rather than smaller model inference. Secondly, until a month or two ago, I had never really received any questions about the data processing part of AI workloads. Maybe you can talk about how CUDA accelerates these workloads?
A7: We can create TensorRT-LLM because CUDA is programmable. If CUDA and our GPUs were not so programmable, it would be difficult for us to improve the software stack at the current speed. TensorRT-LLM on the same GPU can double the performance without anyone touching anything. And of course, the most important thing is that our innovation is so fast that H200 doubled it. So our inference cost has been reduced by four times in about a year. So it's really hard to keep up. Now, everyone loves our inference engine because of our installation base. For 20 years, we have been dedicated to our customer base.
The installation base we have is not only the largest in every cloud, but also available from every enterprise system manufacturer. Companies in almost every industry are using it. Whenever you see an NVIDIA GPU, it runs our stack.It is compatible in terms of architecture.
The stability and determinism of NVIDIA's platform are the reasons why everyone builds on our foundation and optimizes on our foundation. All the engineering work and inventions you make on NVIDIA's foundation will benefit everyone who uses our GPUs. With such a large installed base, millions of GPUs in the cloud, 100 million GPUs in people's PCs, and almost every workstation in the world, they are all compatible in terms of architecture. Therefore, if you are a reasoning platform and are deploying reasoning applications, you are basically an application provider, and as a software application provider, you are looking for a large installed base.
Data processing, before training a model, you need to organize the data. You have to infer the data, maybe you have to use synthetic data to augment the data, so you process the data, clean the data, align the data, and normalize the data. All this data is not measured in bytes and megabytes, but in terabytes and petabytes. The amount of data processing done before data engineering and training is very large.
It may represent 30%, 40%, or 50% of the total workload required to create a data-driven machine learning service. So data processing is just one important part. We accelerate Spark, we accelerate Python. One of the coolest things we just did is cuDF pandas.
Pandas is the most successful data science framework in the world, and now PANDAS is accelerated by NVIDIA CUDA, ready to use without writing a single line of code. So the acceleration is really amazing, and people are very excited about it. The design of PANDAS has only one purpose, which is data processing for data science. NVIDIA CUDA provides you with all of this.
Q8: How do we view the prospects of your R&D and operating expenses growth to support a more proactive and expansive future roadmap, but more importantly, what is the team doing to manage and drive the execution of all this complexity?
A8: First of all, the reason we accelerate execution speed is because it fundamentally reduces costs. The combination of TensorRT LLM and H200 has reduced the inference cost of our customers' large models by four times.
Therefore, we want to accelerate our roadmap. The second reason is to expand the coverage of generative AI, the number of data center configurations in the world. NVIDIA's presence is in every cloud, but no two clouds are the same. NVIDIA works with every cloud service provider, but their network control plane security conditions are different.
We are now pushing all these products to the market. So, complexity includes all the technologies, market segments, and speed. It includes the fact that we are compatible with each of them in terms of architecture. It includes all the domain-specific libraries we have created. That's why every computer company can effortlessly include NVIDIA in its roadmap and push it to the market.The reason is because there is market demand. There is market demand for healthcare. Of course, there is market demand for artificial intelligence, financial services, supercomputing, and quantum computing. We have a wide range of markets and submarkets with specific domain libraries. Finally, we provide end-to-end solutions for data centers. InfiniBand networks, Ethernet, x86, ARM, almost all combinations of solution, technology, and software stack are provided.
This means having the largest number of ecosystem software developers, the largest system manufacturer ecosystem, the largest and most extensive distribution partner network, and ultimately the largest coverage. This certainly requires a lot of energy. But what really brings them together is a great decision we made decades ago, that everything is compatible at the architecture level. When we develop a domain-specific language that runs on one GPU, it runs on every GPU. When we optimize TensorRT for the cloud, we also optimize it for enterprises.
When we do something that brings new features, new libraries, new functionality, or new developers, they immediately benefit from all our influence. Therefore, this principle of architectural compatibility has been going on for decades, and it is one of the reasons why NVIDIA is still very, very efficient.
Risk disclosure and statement for this article: Dolphin Research Disclaimer and General Disclosure