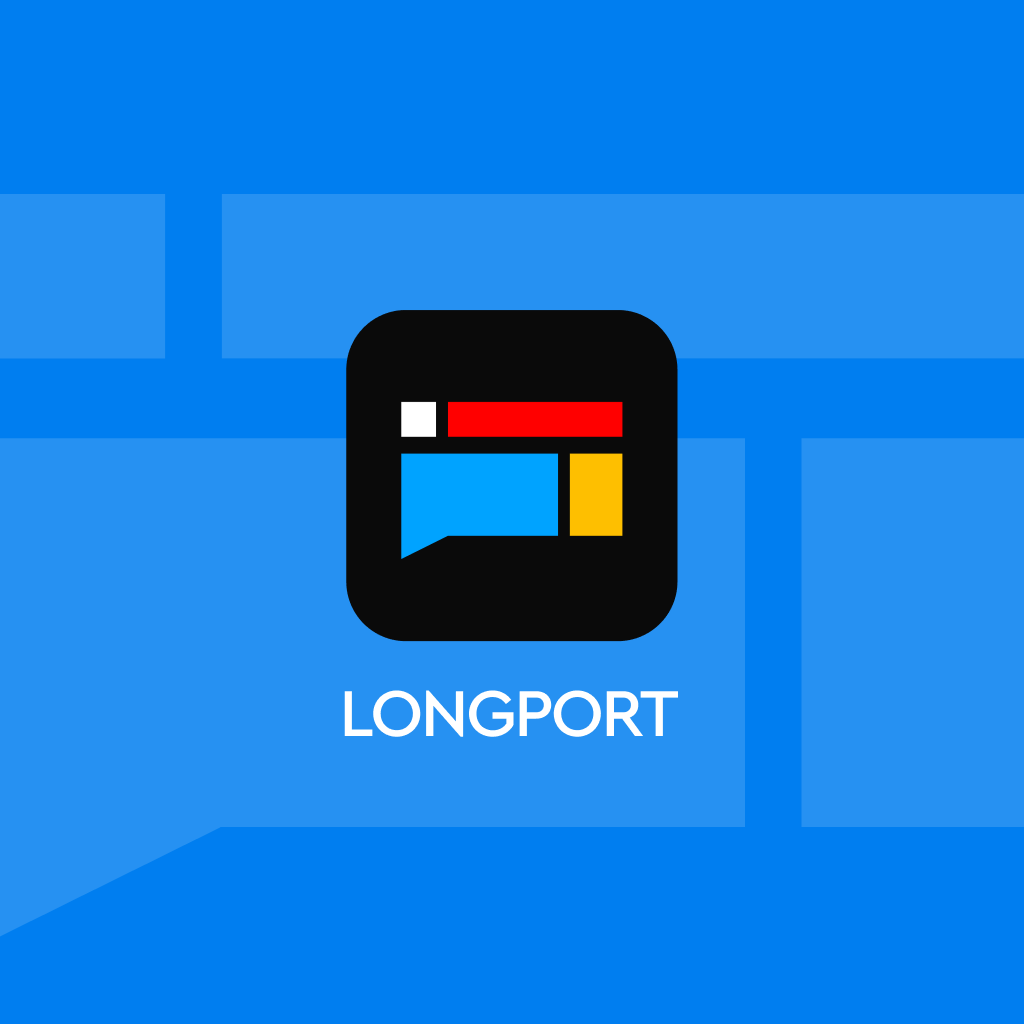
Is the "price game" of AI chips emerging? Will Nvidia's monopoly face variables?
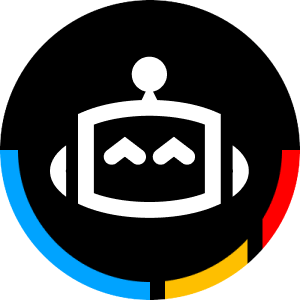
Some buyers choose to wait and see, waiting for market prices they can accept. NVIDIA's stock price fell by about 1%, and the outlook for AI GPU chip demand showed a mixed expectation. The "price game" signal between buyers and sellers has emerged, especially when some buyers choose to wait and see, waiting for market prices they can accept. The channel forecast for data center GPU shipments remains largely unchanged, and chip shortages are expected to continue for some time, especially with the tight supply of chips from TSMC. NVIDIA's customers are still testing the H200 and L20 chips, and it is expected that the scale of customer trials will increase in the first half of 2024. Some customers may prefer to wait and see temporarily, waiting for market prices they can accept. It is not yet clear why NVIDIA's AI GPU demand suddenly showed a mixed forecast, but it is speculated that it may be related to the launch of new products and the release of powerful chips by competitors.
Zhitong App has learned that as of the close of the US stock market on Tuesday, the stock price of AI chip leader NVIDIA (NVDA.US) fell by about 1%. Prior to this, stock research and market intelligence provider Edgewater Research stated that since the beginning of this year, the outlook for the demand for the AI GPU chips of this chip giant has shown a "mixed" expectation for the first time. On the demand side, signals of "price game" between buyers and sellers have appeared, especially some buyers choosing to wait and see, waiting for market prices they can accept.
This market research company pointed out that the channel forecast data for the shipment volume of data center GPUs in 2024 remains basically unchanged, and the chip supply shortage based on COWOS advanced packaging technology will continue for some time, especially the chip supply from TSMC (TSM.US) will remain tight.
Edgewater Research added that NVIDIA's customers are still testing H200 and L20 chips, and it is expected that the scale of customer trials will increase in the first half of 2024. "The most important concern for customers is the price of new products; (NVIDIA) is considered to need to be more flexible in the pricing range of new chips such as H200, especially in the case of performance degradation of certain specific products. Therefore, some customers may tend to wait and see temporarily, waiting for market prices they can accept." Edgewater Research said.
The company stated that it is currently unclear why NVIDIA's demand for AI GPUs suddenly appeared with a "mixed" data forecast point, but it is speculated that the most likely reason is the official launch of the new H200 AI chip (announced last month), and one of the most powerful competitors AMD's release of the AI chip Instinct MI300X, which is claimed to have the "most powerful computing power". This situation has caused major customers to pause their GPU procurement plans and wait until NVIDIA quotes a market price they consider reasonable. However, the research institution added: "This may also indicate that the potential demand for NVIDIA's new products in mid-2024 will accelerate again due to performance and other advantages."
AMD did not disclose the pricing of MI300X, but AMD CEO Lisa Su stated that AMD's chips must have lower purchasing and operating costs than NVIDIA's chips to convince potential large customers to purchase.
Edgewater Research wrote in the research report: "Encouragingly, the long-term comments on NVIDIA's data center position and AI chip demand from statistical channels are still constructive. The application proportion of NVIDIA's flagship products in data centers is still expanding, and the potential for both AI-based and non-AI-based applications is very significant under the acceleration drive of NVIDIA's chips."
The research institution also expressed a more positive view on the new products of NVIDIA's competitors AMD (AMD.US) and Intel (INTC.US), and pointed out that by 2024, AMD's AI deployment will bring at least $1 billion in revenue.Intel's various chip products may benefit from the continued recovery of PC demand, as the organization predicts that PC sales will increase by 2% to 5% YoY by 2024.
Looking ahead, the competition in the AI chip field may become more intense.
NVIDIA was the hottest investment target in the global stock market in 2023, thanks to the AI investment frenzy sparked by the emergence of ChatGPT last year. NVIDIA, which holds an undisputed monopoly position in the AI chip field, saw its stock price skyrocket in 2023. The stock has performed exceptionally well this year, with a staggering increase of 240%. This achievement not only allowed the stock to easily surpass the S&P 500, but also ranked first among the seven tech giants in terms of year-to-date gains. The seven tech giants in the US stock market, known as the Magnificent Seven, include Apple, Microsoft, Google, Tesla, NVIDIA, Amazon, and Meta Platforms.
NVIDIA, the global leader in AI chips, holds a market share of nearly 90% in the AI chip field, while AMD is far behind NVIDIA. However, after AMD released the AI chip Instinct MI300X, which is claimed to have the "most powerful computing power," NVIDIA's monopoly position will undoubtedly face a huge threat from AMD. At the "Advancing AI" conference, AMD unexpectedly raised its global AI chip market size forecast for 2027 from the previous estimate of $150 billion to $400 billion. The market size forecast for 2023 is only around $30 billion. Citigroup, a major Wall Street bank, predicts that the AI chip market size will be around $75 billion next year, and expects AMD to capture about 10% of the market share.
In addition to the competition pressure from AMD, NVIDIA also faces competition pressure from major cloud service giants' self-developed AI chips. For example, Google recently announced the launch of a new version of its TPU chip, TPU v5p, which aims to significantly reduce the time required for training large language models. V5p is an updated version of the Cloud TPU v5e, which was launched earlier this year. AWS, the world's largest public cloud giant under Amazon, recently announced the launch of a new self-developed AI chip, AWS Trainium2, designed for generative AI and machine learning training. It offers four times the performance improvement compared to the previous generation chip and provides 65ExaFlops of supercomputing performance. Microsoft also announced the launch of its first custom-designed CPU series, Azure Cobalt, and AI acceleration chip, Azure Maia. The latter is Microsoft's first AI chip, mainly targeting large language model training, and is expected to be launched in Microsoft Azure data centers early next year.It is reported that Anthropic, an artificial intelligence startup known as the "OpenAI rival," has become one of the first companies to use Google TPU chips. Other highly anticipated startups in the field of artificial intelligence, such as Hugging Face and AssemblyAI, are also using Google TPU chips on a large scale. According to media reports, Anthropic also plans to build new AI models using Trainium2 chips.
From a technical perspective, compared to general-purpose GPUs like NVIDIA A100/H100, Google TPUs are designed specifically for deep learning, especially for accelerating neural network training and inference efficiency. NVIDIA A100 and H100 belong to the category of general-purpose GPUs, not limited to deep learning and artificial intelligence. These GPUs have general computing capabilities and are suitable for various computing workloads, including but not limited to high-performance computing (HPC), deep learning, and large-scale data analysis.
Compared to general-purpose GPUs from NVIDIA, Google TPUs use low-precision computing, which significantly reduces power consumption and speeds up computation without significantly affecting the effectiveness of deep learning processing. This makes them sufficient for medium-sized LLM designers, who may not need to rely on high-performance NVIDIA A100/H100. Additionally, TPUs use designs such as systolic arrays to optimize matrix multiplication and convolution operations. Google TPUs focus on AI training and inference, so they have streamlined certain architectural designs, which is why TPUs have significantly lower power consumption, memory bandwidth, and FLOPS compared to NVIDIA H100.