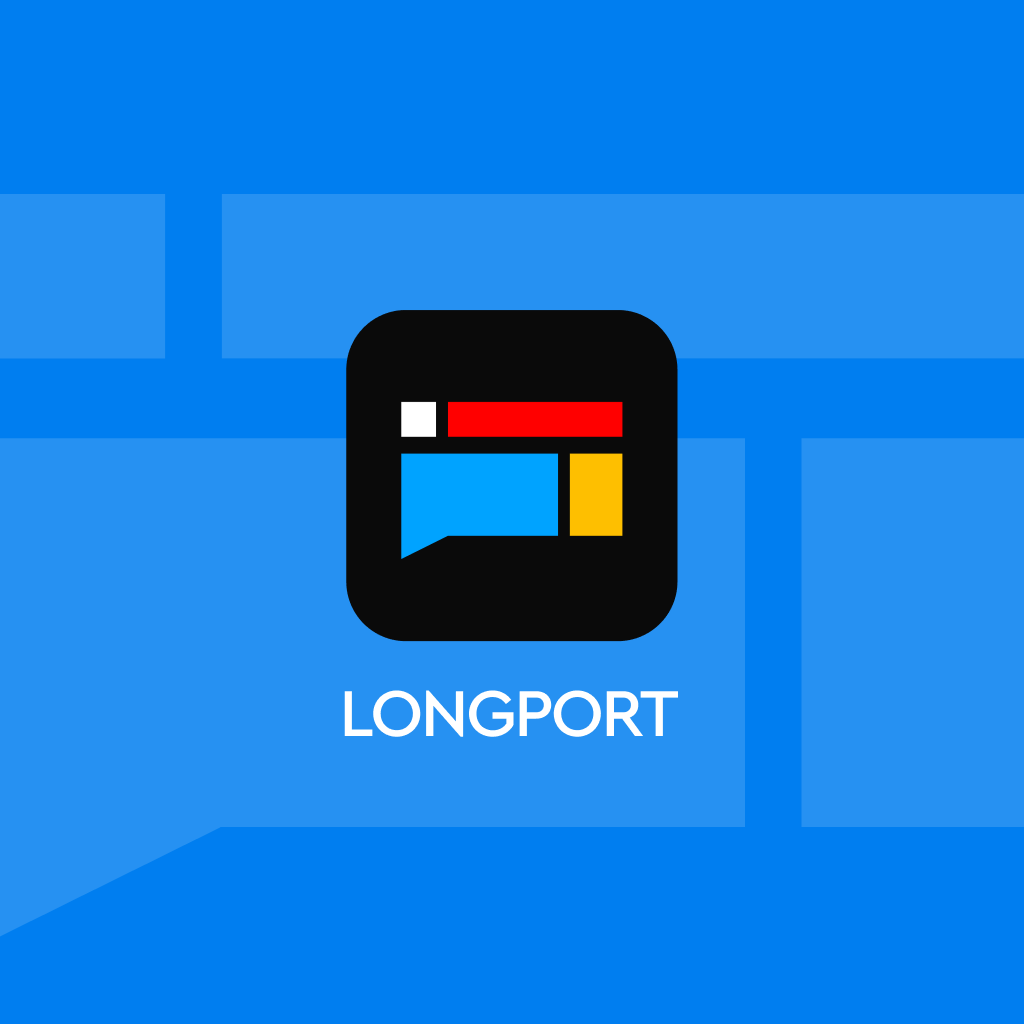
During the NVIDIA conference call, it was projected that the next-generation B100 chips will be in short supply, and the global data center installation volume is expected to double in the next 5 years.
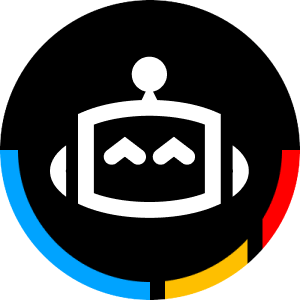
NVIDIA pointed out that although the current GPU supply is improving, the demand remains strong. It is expected that the market demand for the next generation of products will exceed supply, especially with the anticipated launch later this year of the new generation chip, B100.
Huang Renxun believes that the entire data center industry is undergoing a new revolution.
During the NVIDIA earnings call held on Wednesday local time, he stated that AI factories, as a new type of data center, will transform data into valuable "tokens," such as what people experience when using services like ChatGPT and Midjourney.
Huang Renxun mentioned that the computing industry is at the beginning of an accelerated transition towards computing and generative AI, which will double the global data center infrastructure installations in the next 5 years, creating market opportunities worth billions of dollars annually.
Considering the broad market prospects, Huang Renxun stated that the conditions for continued growth in the data center business are excellent beyond 2024, with AI Enterprise expected to become a very important business in the future.
As for whether NVIDIA will enter the ASIC (Application-Specific Integrated Circuit) market, Huang Renxun did not provide a direct answer.
NVIDIA CFO Colette Kress stated during the earnings call that while the current GPU supply is improving, demand remains strong, with the market demand for the next generation of products expected to exceed supply, especially for the new B100 chips expected to ship later this year. She mentioned that "building and deploying AI solutions have touched almost every industry," and forecasted that data center infrastructure scale will double in five years.
The financial report released on Wednesday local time showed that NVIDIA's fourth-quarter revenue surged by 265% year-on-year to $22.1 billion, exceeding analysts' expectations of $20.41 billion, with quarterly revenue even surpassing that of the entire year of 2021. The largest revenue source was the data center segment, with fourth-quarter revenue reaching $18.4 billion, a staggering 409% increase year-on-year.
NVIDIA's revenue and profit have set historical records for three consecutive quarters, with a 126% revenue growth for the full fiscal year 2024.
Here is the full transcript of the analyst Q&A session during NVIDIA's fourth-quarter earnings call:
Goldman Sachs Toshiya Hari:
My question is about the data center business, Jensen.
It's obvious that you are doing very well in this industry. I am curious about your expectations for the data center business in the calendar years 2024-2025, any changes in the past three months?
Could you talk about some emerging trends in the data center field, such as software, sovereign AI, etc.? I think you have already clearly expressed the medium to long-term issues.
There was recently an article about NVIDIA possibly entering the ASIC market. Is there any credibility to this? If so, how will NVIDIA compete and develop in this market in the coming years?
Huang Renxun:
Regarding the first question. In one quarter, we are guiding, but fundamentally, the growth environment for 2024-2025 and beyond is very promising. The reason being, we are at the beginning of two industry-wide transformations, both of which are industry-wide.
The first is the shift from general computing to accelerated computing. As you know, general computing is losing momentum. You can see this from the expansion of cloud service providers (CSPs) and many data centers, including our own general computing center, which has extended depreciation from four years to six years. But when it can no longer significantly increase its data processing capacity as before, there is no reason to use more CPUs for updates, so everything must be accelerated.
This is what NVIDIA has been pioneering. Accelerated computing can significantly improve LNG efficiency, reducing data processing costs by 20 times, which is a huge number. Of course, there is also speed.
The speed is incredible, to the point that today we are experiencing the second industry-wide transformation, known as Generative AI.
Yes, we can, I believe we will discuss a lot in the call, but please remember, Generative AI is a new application. It is empowering a new way of software generation, creating a new type of software. This is a new way of computing. You can perform Generative AI tasks on traditional general computing devices. This process must be accelerated.
Thirdly, it is empowering a whole new industry, which is worth taking a step back to examine, related to your last question about sovereign AI.
In a sense, the entire data center industry is undergoing a new transformation. Traditionally, data centers were only used to compute data, store data, and provide services to company employees. Now, we have a new type of data center that focuses on AI generation. I have described it as an AI factory, essentially using raw materials, which is data, to transform it with these AI supercomputers produced by NVIDIA into highly valuable "tokens."
These "tokens" are what people experience in the astonishing ChatGPT, Midjourney, or now search services, all of which are now enhanced by this. All the super-personalized services related to this, these incredible startups, digital biology generating proteins, chemicals, and so on, all these "tokens" are generated in a highly specialized data center. We call this type of data center an AI supercomputer and AI generation factory.
But what we see is diversity, we have made a lot of progress in this area. Firstly, the amount of inference we do has skyrocketed, almost every time you interact with ChatGPT, we are doing inference, every time you use Midjourney, we are also doing inference. Every time you see these amazing generative videos, or videos edited by Runway, Firefly, NVIDIA is doing inference, our inference business has expanded significantly, to about 40% or so. The training volume continues to increase as these models become larger, and the inference volume is also growing. We are diversifying into new industries. Large CSPs are still expanding, evident from their capital expenditures and discussions. Enterprise software platforms are deploying AI services, with Adobe, SAP, and other companies as prime examples.
Moreover, consumer internet services are now enhancing all their services through generative AI to create more hyper-personalized content.
We are talking about industrialized generative AI. Overall, in vertical industries such as automotive, financial services, and healthcare, the scale has now reached billions of dollars.
Of course, there is also sovereign AI.
The reason for proposing sovereign AI is that each region has different languages, knowledge, history, and culture, with their own data. They want to utilize their data to train AI models, develop digital intelligent services, and create their own AI systems. Data varies worldwide.
Because this data is most valuable to each country, nations will protect their data, handle it themselves, develop and provide AI services independently, rather than relying on external AI systems.
Therefore, we see Japan, Canada, France, and many other regions building sovereign AI infrastructure. Hence, my expectation is that the experience of the western United States will certainly be replicated worldwide. In the future, these AI foundries will be present in every industry, company, and region.
I believe that generative AI truly became a new application space last year, a new way of computing. A new industry is forming, driving our growth.
Morgan Stanley analyst Joe Moore:
40% of revenue comes from the inference business, which is higher than I expected. What was this proportion about a year ago, and how much has the inference business related to large models grown? How does NVIDIA measure this ratio? Is it reliable? Because I assume that in some cases, the same GPU can be used for both training and inference.
Huang Renxun:
I would say that this proportion may be underestimated. Let me tell you why. A year ago, when people used the internet, browsed news, watched videos, listened to music, and viewed recommended products, they faced trillions of data. Assuming your phone is only three inches, integrating all this information into such a small space requires a powerful recommendation system.
These recommendation systems were all CPU-based in the past but have recently shifted to deep learning and generative AI, directly benefiting from GPU acceleration.
It requires GPU for accelerating embeddings, nearest neighbor search, re-ranking, and generating personalized recommendations. So now, every step of the recommendation system's operation requires a GPU. As you know, recommendation systems are the largest software engine on this planet. Almost all major companies in the world need to operate these large-scale recommendation systems.
Whenever you use ChatGPT, you are engaging in inference, and when you hear about the quantity of things they generate for consumers along the way. When you see Getty, the work we do with Getty and Firefly from Adobe, these are all generative models. The list goes on. All of these that I mentioned a year ago did not exist, they are all 100% new.
Every time you use ChatGPT, you are reasoning, and Midjourney is the same. Our collaborations with Getty, Adobe, Firefly, and these applications of generative models are constantly increasing. All of these that I just mentioned, did not exist a year ago, they are all 100% new.
Bernstein Research analyst Stacy Rasgon:
You expect the next generation of products to face supply shortages. I would like to delve into this situation, what are the reasons for the tight supply of next-generation products? Since GPU supply is easing, why would there still be a supply shortage for the next generation of products?
How long do you expect this constraint to last? Does it mean that throughout the entire calendar year of 2025, there will be a shortage of supply for next-generation products?
Huang Renxun:
Overall, our supply is improving. Our supply chain is doing exceptionally well, from wafers, packaging, storage, all power regulators, to transceivers, networks, cables, and all the components we use. As you know, people think NVIDIA GPUs are just a chip, but the NVIDIA Hopper GPU consists of 35,000 parts, weighing 70 pounds. These are very complex things we manufacture, and there are good reasons why people call it an AI supercomputer. If you look behind the data center, the systems, the wiring is incredible. It is the most dense and complex network cabling system in the world.
Our InfiniBand business has grown fivefold year-on-year. The supply chain has provided us with great support. So overall, the supply is improving. We expect demand to still exceed supply, and we will do our best. The process cycle is shortening, and we will continue to strive.
However, as you know, every time we develop a new product, it goes from zero to a very large number, which cannot be achieved overnight. Everything is accelerating, but it won't happen overnight. Therefore, when we develop a new generation of products, we cannot fully meet the demand in the short term, and now we are increasing the supply of H200.
We are also expanding the construction of the Spectrum-X platform. This is a brand-new product as we enter the Ethernet world, and it has made very good progress. InfiniBand is the standard for AI-specific systems, and we have enhanced it on the basis of new features in Ethernet, such as adaptive routing, congestion control, noise isolation, or traffic isolation, so that we can optimize Ethernet for AI. Therefore, InfiniBand will be our AI-specific infrastructure. Spectrum-X will be our AI-optimized network, and it is accelerating.
So, demand exceeds supply, which is the common issue new products often face. We are working as fast as we can to meet the demand. Overall, our supply is increasing very smoothly.
TDCowen analyst Matt Ramsey:
Good afternoon, Jason. My question consists of two parts, related to the supply shortage Stacy just mentioned. First, how does your company consider product allocation to meet customer demand, and do you monitor product inventory to avoid stockpiling?
Then, Jason, I really want to hear your thoughts on how you and NVIDIA allocate products among customers. Many customers are competing for chips, from industry giants to small startups, from healthcare to government. It's a very unique technical activity that you are driving, and I am very interested in hearing how you think about fair distribution to safeguard the company's interests while also considering the interests of the entire industry.
Huang Renxun:
First, to answer your question about how we work with customers to build GPU instances and our allocation process.
Our collaborative customers have been long-term partners for many years, and we have been assisting them in deploying in the cloud and setting up internally. Many service providers operate multiple products to meet various end-user and internal needs.
Of course, they plan the configurations needed for new clusters in advance. We not only discuss the current Hopper architecture but also help them understand the next wave of products, listening to their interests and needs. So, their procurement and construction content is an ongoing process of change. But the relationships we have built and their understanding of the complexity of construction have indeed helped us with allocation, and both contribute to our communication with them.
First, our CSP has a very clear understanding of the product roadmap and iterations. Our transparent communication with them gives them confidence in the timing and location of product placement. They understand the timeline of the product and the approximate supply volume. In terms of allocation, we strive for fair distribution, avoiding unnecessary allocations. As you mentioned earlier, if the data center is not ready, allocation is meaningless. Nothing is more challenging than fair distribution of idle resources and avoiding unnecessary allocations.
Regarding the end market you asked about, we have an excellent ecosystem, including OEMs, ODMs, CSPs, and the very important end market.
What truly sets NVIDIA apart is our ability to bridge partners and customers. We connect cloud service providers and manufacturers with customers in healthcare, finance, AI developers, large language model developers, autonomous driving companies, and robotics companies. Various robotics startups are emerging, from warehouse robots, surgical robots, humanoid robots, to various interesting robotics companies, and agricultural robotics companies. At the intersection of surgery and humanoid robots. All these startups and major companies are built on top of our platform. We directly support them. Typically, we can achieve a win-win situation by allocating to cloud service providers and simultaneously bringing customers to them.
The unique aspect is that we bring our customers, we bring our partners, CSPs, and OEMs, and we bring customers to them. Biotech companies, healthcare companies, financial services companies, AI developers, large language model developers, autonomous driving car companies, robot companies. A large number of robot companies are emerging. Their warehouse robots, surgical robots, humanoid robots, agricultural robots... various very interesting robot companies, all these startups are helping major companies in fields like healthcare, finance, and automotive work on the NVIDIA platform. We directly support them. Typically, we can achieve a win-win situation by allocating to CSPs and simultaneously bringing customers to CSPs.
So, this ecosystem, as you rightly pointed out, is vibrant, but at its core, we aim for fair distribution, avoiding resource waste, seeking opportunities to connect partners and end-users, and we are always looking for these opportunities.
UBS analyst Timothy R. Curry:
Thank you very much. I would like to ask how you convert backlog orders into revenue. Obviously, your product delivery time has been greatly reduced, but Colette did not mention inventory purchase commitments, but if I add the inventory purchase commitments you have obtained and the prepayment support for supply, you know, your total supply, in fact, has decreased a bit. How should we interpret this decrease? Is it because delivery times have shortened, and NVIDIA no longer needs to make such large financial commitments to suppliers? Or is NVIDIA approaching a balance between filling orders and backlog orders?
Colette Kress:
Yes, let me emphasize our view on three different aspects of supply. You are right, considering the inventory we have on hand, given the allocations we are making, we are working hard because goods are shipped to customers immediately upon receipt. I believe customers will appreciate our ability to meet their delivery schedules.
Secondly, our purchase commitments have many different components, including not only the components needed for our production but also the components needed to meet capacity, with varying lengths of demand time, some of which may last for two quarters, but some may last for several years.
Prepayments are the same, all to ensure that we obtain the spare capacity we need from several suppliers in the future.
So, these roughly similar figures should not be overinterpreted because we are increasing supply. All of these have different time spans, as sometimes we have to purchase things well in advance or build capacity for us. Colette, congratulations on achieving such outstanding results. I'd like to discuss your views on gross margin, which have returned to around 75%, is this due to the HBM memory content in the new products? Do you think there are other reasons? Thank you very much.
Colette Kress:
Thank you for your question. As we emphasized in the opening remarks, our performance in the fourth quarter and the outlook for the first quarter are both quite unique. The gross margins in these two quarters are also unique because they benefit from component costs in the supply chain, covering our computing and networking products, as well as multiple stages of the manufacturing process.
Looking ahead, we predict that the gross margin for this fiscal year will return to around 75%, back to the levels before the peak in the fourth and first quarters. So, the key driver of the gross margin for the full year ahead will be our product portfolio, which will be the most important factor. These are the main factors driving the gross margin.
Analyst CJ Muse:
Good afternoon, Jason. GPU computing power has increased by 1 million times in the past decade. Faced with this rapid advancement, how do NVIDIA's customers view the long-term availability of their NVIDIA investments today? Can systems used for training today still be used for inference in the future? How do you see this developing?
Huang Renxun:
Thank you for your question. Yes, the really cool thing is that we can greatly enhance performance because our platform has two key features: acceleration capability and programmability.
NVIDIA is the only one with a consistent architecture from the beginning, from the initial convolutional neural networks, AlexNet proposed by Alex Krizhevsky, Ilya Sutskever, and Geoffrey Hinton, to RNN, LSTM, RLS, and now Transformers, such as Vision Transformers, Multi-modality Transformers, and so on. Every variant model of AI, we can support them, optimize our stack, and deploy them on the installed user devices.
This is truly remarkable. On one hand, we can invent new architectures and technologies, like our Tensor Cores, tensor core conversion engines, improving data formats and processing structures, just as we did in Tensor Cores.
At the same time, we also support the existing installed base. Therefore, all our new software algorithm innovations, as well as all the new model innovations in the industry, can run on one foundation. On the other hand, when we see something revolutionary, like Transformers, we can create something entirely new, like the Hopper transformation engine, and apply it in the future. Therefore, we have the ability to introduce software into the installation base and continuously improve it, so over time, our customer installation base will be enriched with our new software. On the other hand, new technologies have created revolutionary capabilities.
If breakthroughs occur in the next generation on LLM, don't be surprised. Some of these breakthroughs are in software because they run on the CUDA platform, providing to the installation base. So, we are moving forward with everyone. On the one hand, we are making huge breakthroughs; on the other hand, we are supporting the existing base.
Piper Sandler analyst Harsh Kumar:
I would like to talk about NVIDIA's software business. I am pleased to hear that its revenue exceeds $1 billion. But I hope that Jensen or Collatif can help us break it down a bit so that we can better understand the growth sources of this business.
Huang Renxun:
Let me step back and explain the fundamental reasons why NVIDIA is so successful in software.
First of all, as you know, accelerated computing is truly thriving in the cloud. CSPs have very large engineering teams, and the way we collaborate with them allows them to operate and manage their own business. If there are any issues, we will send out a large team to serve them. Their engineering teams directly interface with our engineering teams to improve, fix, maintain, and repair complex accelerated computing software stacks.
Accelerated computing is fundamentally different from general-purpose computing. It doesn't start with a program like C++ and compile it to run on all CPUs. From data processing, structured data, all the way to unstructured data like images, text, PDFs, classic machine learning, computer vision, speech, large language models, and all recommendation systems, each field requires a different software stack. That's why NVIDIA has hundreds of software libraries. Without software, new markets cannot be developed, and new applications cannot be enabled. Software is the foundation for accelerated computing.
As you know, accelerated computing is very different from general-purpose computing. You don't start with a program like C++. You compile it, and everything runs on all your CPUs. The software stack required for each field ranges from data processing, SQL databases, structured data, all images, text, and PDFs (unstructured) to classic machine learning, computer vision, speech, two large language models, all recommendation systems, all of which require different software stacks.
This is why NVIDIA has hundreds of libraries. Without software, you cannot open up new markets. Without software, you cannot open and enable new applications. Software is crucial for accelerated computing.
This is the fundamental difference between accelerated computing and general-purpose computing, which most people took a long time to understand. Now people understand that software is crucial in the way we cooperate with CSPs. It's very simple; our large team is working with their large team. However, now generative AI allows every enterprise and enterprise software company to embrace accelerated computing. Embracing accelerated computing is now essential, as relying solely on general-purpose computing to increase processing power is no longer feasible. All these enterprise software companies and corporations lack large engineering teams to maintain and optimize their software stacks for running globally across all clouds, private clouds, and on-premises.
Therefore, we will manage, optimize, patch, and fine-tune all their software stacks, optimizing the installation foundation. We will containerize them into our stack, which we call NVIDIA AIEnterprise. We will market NVIDIA AIEnterprise as a runtime, just like an operating system. It is an artificial intelligence operating system. We charge $4,500 per GPU annually. My guess is that every enterprise in the world, every software company deploying software in all clouds and private clouds. Initially, we will run on NVIDIA AI Enterprise, especially, you know, obviously for our GPUs. So, over time, this could become a very significant business. We are off to a good start. Collette mentioned that it has grown at a rate of $1 billion, and we are just getting started.
Huang Renxun's closing remarks:
The computing industry is shifting in two directions simultaneously (accelerated computing and generative AI).
The trillion-dollar value of data center installations is transitioning from general-purpose computing to accelerated computing. Every data center will be accelerated, enabling the world to meet computational demands while managing costs and energy. NVIDIA has achieved incredible acceleration, ushering in a new computing paradigm, generative AI, where software can learn, understand, and generate any information from human language to biological structures and the 3D world.
We are at the beginning of an emerging industry, with specialized AI data centers processing vast amounts of raw data to distill it into digital intelligence, much like the exchange power plants of the last industrial revolution. The NVIDIA AI supercomputer is essentially the AI generation factory of this industrial revolution.
Every company and every industry is fundamentally built on its proprietary business intelligence. In the future, their proprietary generative AI will kick off a new investment cycle to build the infrastructure for the next trillion-dollar AI generation factory.
We believe that these two major trends will double the global data center infrastructure installations in the next 5 years, creating billions of dollars in market opportunities annually. This new artificial intelligence infrastructure will open up a whole new world of applications that were previously impossible.
We have embarked on the AI journey with hyperscale cloud providers and consumer internet companies. Now, from automotive, healthcare, financial services to industrial telecommunications, media, and entertainment, every industry is involved. NVIDIA's full-stack computing platform has industry-specific application frameworks and a vast developer and partner ecosystem, providing us with speed, scale, and influence to help every company, every industry become an AI company. At the upcoming GTC in San Jose next month, we have a lot to share with you, so be sure to join us. We look forward to presenting to you the latest developments of the next quarter.