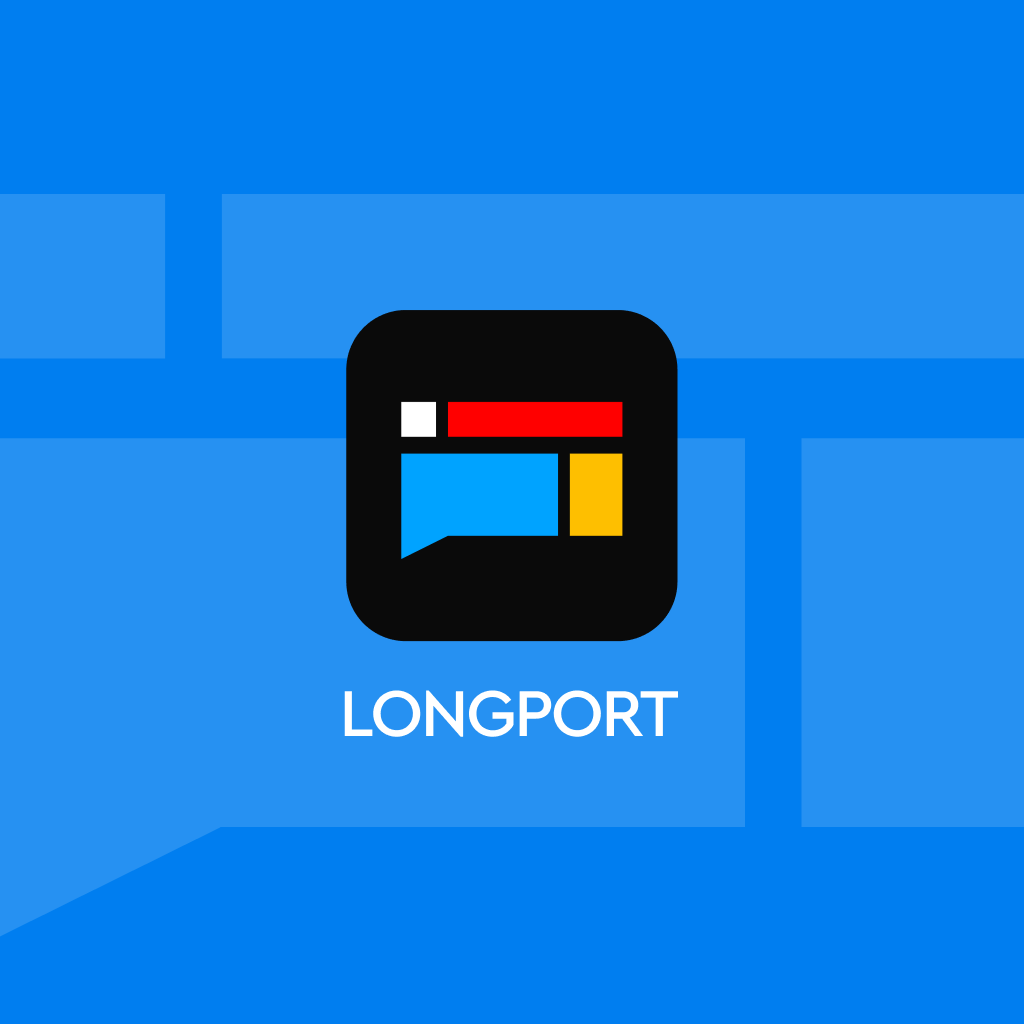
The AI industry buys NVIDIA GPUs, spending 17 times more money than it earns
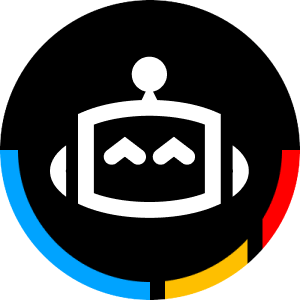
The AI industry buys NVIDIA GPUs, spending 17 times more money than it earns. Microsoft, Google, and Meta are unable to acquire due to antitrust regulations, so they can only invest in AI technology development. Depreciation of GPU expenses can avoid paying more taxes. Star startup company Cognition Labs has nearly six times higher valuation, and AI code tool Devin is considered a major leap in artificial intelligence, indicating the beginning of a large-scale automation road in software development. The rise of generative AI field, with Cognition being a rising star
Building AI large models is really burning money.
We know that a large part of today's generative AI is a game of capital, with tech giants leveraging their powerful computing power and data to take the lead, and using advanced GPU parallel computing power to promote its implementation. What is the cost of doing this?
A recent article in The Wall Street Journal about star startups provided an answer: the investment is 17 times the output.
Last weekend, the machine learning community heatedly discussed this number.
Star startups, doubling valuation in a few weeks: but no revenue
AI startup Cognition Labs, supported by renowned investor Peter Thiel, is seeking a valuation of $2 billion, and a new round of financing has increased the company's valuation by nearly six times in just a few weeks.
In the hot field of generative AI today, Cognition is a rising star. If you're not familiar with it yet, here are two key words: International Olympiad gold medal team, the world's first AI programmer.
Founded by Scott Wu, Cognition's team is eye-catching, currently consisting of only 10 people, but including many gold medalists from international informatics Olympiads.
The company launched an AI code tool called Devin in March this year, claiming to be the "first AI programmer close to humans", capable of independently completing complex coding tasks, such as creating custom websites. From development to deployment, and then to debugging, just provide the requirements in natural language, and AI can do it.
The news quickly made headlines in many media outlets and became a hot topic:
Some investors have expressed that Devin represents a significant leap in artificial intelligence and may herald the beginning of large-scale automation in software development.
Although Cognition is amazing, it is not alone. Recently, generative AI has shown an unimaginable ability to attract funds. In December last year, France-based Mistral raised $415 million in financing, with a valuation of $2 billion, about seven times higher than the previous summer's round of financing In early March, Perplexity, an AI startup aiming to challenge Google's dominance in web search, also announced news of a new round of financing, with a new valuation expected to reach nearly $1 billion.
Meanwhile, as a startup company aiming to provide AI automatic code tools, Cognition only started developing products last year and has not yet achieved meaningful revenue figures. Earlier this year, in a $21 million financing round led by Founders Fund, the company was valued at $350 million. It is reported that Peter Thiel, a well-known American venture capitalist and founder of Founders Fund, helped lead the investment in Cognition.
AI code writing seems to be a promising direction for large-scale applications, and other companies offering similar products have also seen growth. In the last quarter, Microsoft's code tool GitHub Copilot saw a 30% increase in users to reach 1.3 million. Magic AI, a competitor of Cognition, received a $117 million investment in February. There are also some domestic startups in China that are accelerating industry implementation after the outbreak of generative AI technology.
Despite encouraging signs of growth and the continuous expansion of new company valuations, this rapid development has also raised concerns about the emergence of a bubble. So far, few startups have been able to demonstrate how they can make money, and there seems to be no way to recoup the high costs of developing generative AI.
In a speech in March, an investor from Sequoia Capital estimated that the AI industry spent $50 billion solely on training large models on NVIDIA chips last year, while generating revenue of $3 billion.
So, excluding electricity costs, expenses are 17 times revenue.
How about it, can you still afford to play this year?
Where is the way out
The outbreak of generative AI technology today can be said to have validated the assertion of reinforcement learning pioneer Richard S. Sutton in "Bitter Lesson" that using computing power is the key. Huang Renxun also stated two weeks ago at GTC: "General computing has lost its momentum, and now we need larger models, larger GPUs, and we need to stack GPUs together... This is not to reduce costs, but to expand scale." However, after the emergence of large models with parameters in the range of billions and trillions, whether the method of improving intelligence by increasing scale can continue is an unavoidable question. Moreover, large models nowadays are already very expensive.
An article in The Wall Street Journal quickly sparked a lot of discussion. Some netizens believe: "Capital expenditure is usually one-time, while the income from investment accumulates over time. Generative AI is just getting started, and its subsequent economic benefits could be huge."
However, this optimistic view was quickly refuted by another netizen who pointed out: "Capital expenditure is indeed one-time, but GPUs will depreciate relatively quickly."
Why is it said that GPUs will depreciate rapidly? Although older versions of GPUs will still support CUDA (a computing platform introduced by NVIDIA), compared to the H100, the energy consumption of the V100 is a huge waste.
After all, also in March, NVIDIA has released the all-new generation of AI-accelerated GPU Blackwell series.
If using the V100 can make money, then of course there is no problem. However, as many media reports have pointed out, for most companies at this stage, running large models has not translated into actual income.
On the other hand, considering that new large models are being introduced every week, even though GPUs from a few years ago may be acceptable in terms of computational power, large models are also experiencing "rapid depreciation." Can the infrastructure of today support AI seven years from now?
Furthermore, if a company spends a large amount of money to purchase V100s, trying to keep up with the trend of generative models, it may face the problem of insufficient research team hiring costs, and ultimately may still not be able to produce products with practical applications and economic benefits.
It is worth noting that many large language models (LLMs) require additional processing layers to eliminate illusions or solve other problems. These additional layers significantly increase the computational cost of generative models. It is not a 10% small increase, but a magnitude increase in computational complexity. And many industries may require such improvements.
From an industry perspective, running large generative models requires large data centers. NVIDIA is very familiar with this market and continues to iterate and update GPUs. Other companies may not be able to invest hundreds of billions of dollars just to compete with them. And the demand for these GPUs is not only from major internet companies, but also from many startups, such as Groq, Extropic, MatX, Rain, and so on.
Finally, some have given a "reason" for such exaggerated investments: cash-rich companies like Microsoft, Google, and Meta, who, due to antitrust regulations, cannot continue acquisitions and can only choose to invest their funds in AI technology development. The depreciation of GPU expenses can be used as a loss to avoid paying more taxes.
But this is not something that startup companies need to consider.
In any case, competition will determine the winner. Regardless of how much money is spent, becoming the first may bring potential returns...
However, what kind of returns, we cannot predict yet. Could it be that NVIDIA is the real winner of generative AI?