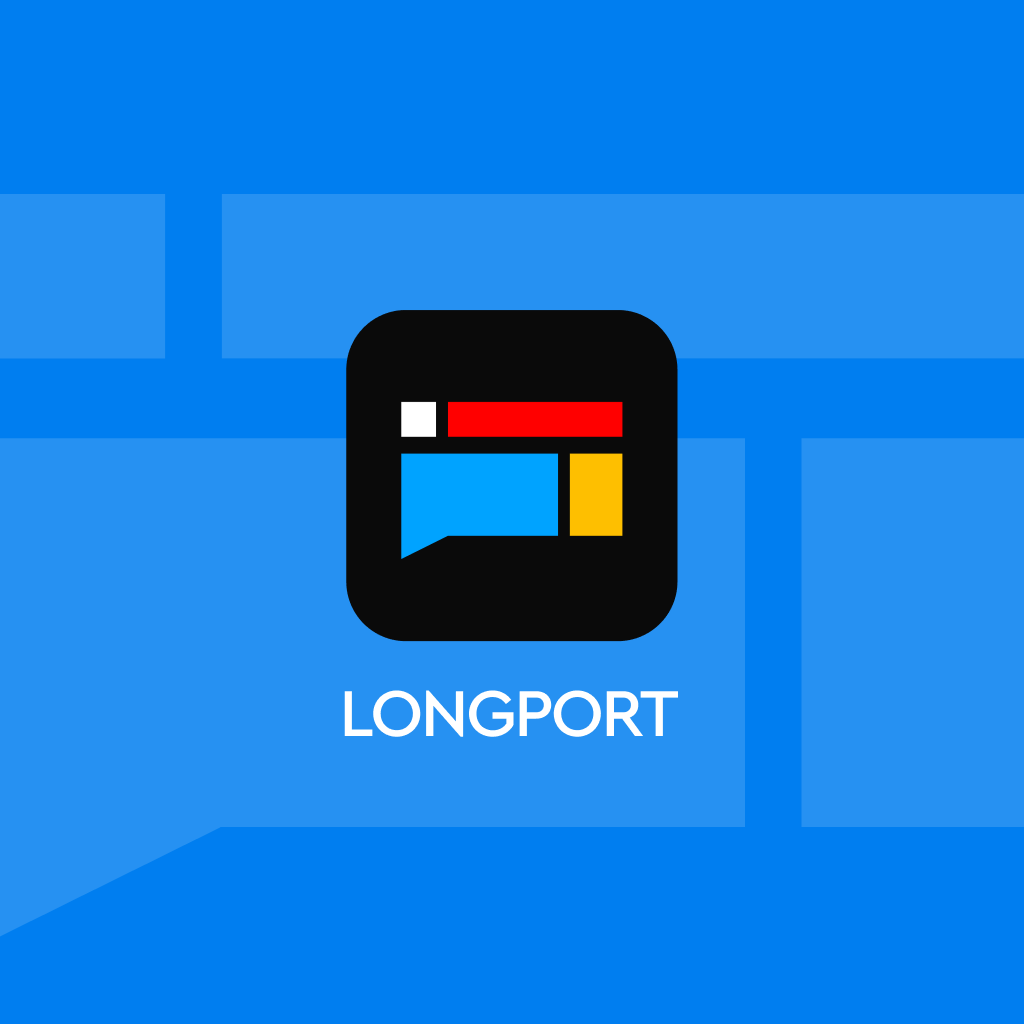
Alibaba Cloud's large-scale model "Juejin"
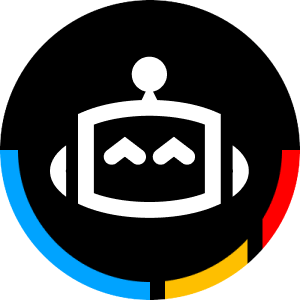
Increase efficiency
Author | Chai Xuchen
Editor | Zhou Zhiyu
The era of large-scale model players has entered the "harvesting moment", kicking off a race to land industry scenarios.
On May 24th, Alibaba Cloud's one-stop financial scenario development platform "Tongyi Dianjin" was upgraded, providing rich financial scenario templates and professional plugins.
As a member of the Tongyi family, "Tongyi Dianjin" was initially positioned as a "smart investment research assistant" for consumers. The upgraded 2.0 version not only enriches the landing of large models in the financial industry, but also further expands to the business side.
For the continuous iteration of financial model platforms, Alibaba Cloud values the attractive imaginative space behind the financial industry.
McKinsey pointed out that generative AI can further expand the "value pool" of the financial industry. Just looking at the banking industry, it will bring about $200 billion to $340 billion in additional value to the entire industry.
In fact, under the wave of digitalization, banks and securities firms are facing strong demands for digital transformation. Insiders in the industry said, "Banks are now looking for scenarios, credit demands, good assets everywhere, and there is a strong desire for breakthroughs internally."
Zhang Chi, Vice President of Alibaba Cloud Intelligence Group, revealed to Wall Street News that customers in the financial industry are very active, with many people registering for tomorrow's meeting that the venue cannot accommodate. Insiders at Alibaba Cloud pointed out that the revenue contributed by the financial industry accounts for a considerable portion of the cloud business.
Zhang Chi stated that behind this, there needs to be a platform that affects the entire IT upstream and downstream chain, allowing everyone to work in a general direction. "The financial industry cannot just choose one company to handle everything from computing power, to models, to tools, to business, and Alibaba Cloud wants to play a key role in between."
The ready-to-use "Tongyi Dianjin" is the killer weapon unveiled by Alibaba Cloud. "Financial institutions no longer need to focus on the principles of databases and clouds, they only need to focus on loans, advanced processes, and truly spend time optimizing the value of business application scenarios." Zhang Chi frankly said, "Having their own provisions, self-built platforms, and self-developed tools will be very challenging for them."
If large models can successfully land in the financial industry, Alibaba Cloud's AI + public cloud model will usher in a commercial virtuous cycle.
Because in Zhang Chi's view, China's software and IT development is prone to fragmentation, and the domestic SaaS market has not been successful in the past. However, as the value of large model application business scenarios emerges, APIs have generated new SaaS models and a new platform economy.
However, compared to other fields, the financial industry has extremely stringent requirements for data security, privacy compliance, timeliness, and accuracy, which means that the application and landing of large financial models face multiple obstacles. How will Alibaba Cloud face this challenge?
The following is a transcript of the conversation with Zhang Chi, Vice President of Alibaba Cloud Intelligence Group and General Manager of the New Financial Industry (edited): Question: What are the core changes in version 2.0 of "Tongyi Dianjin"?
Zhang Chi: In the past, "Dianjin" mainly enhanced the model corpus data, but today it has made many financial-level enhancements at the AI native (financial-grade AI native) application layer. Previously, common financial knowledge in the industry was embedded in the model, so when you asked "Dianjin" finance-related questions, it would talk nonsense. Today, it can provide more professional answers.
Financial-grade AI native is designed and optimized specifically to meet the strictest requirements of the financial industry. It is not only highly advanced in terms of technology but also meets high standards in security, reliability, scalability, and compliance, allowing developers to focus on the fundamental business logic and patterns.
We hope to build a large model with API as the core, enabling more openness and collaboration. We aim to avoid each entity building its own system in this field, so if you have a good business application scenario, you can integrate it.
Question: Everyone is talking about the accelerated landing of large-scale model applications in various industries by 2027. How is the financial industry's acceptance of large-scale model applications compared to other industries?
Zhang Chi: Large-scale models are currently most widely adopted in financial institutions because the financial industry is intensive in development. Acceptance in this field is very high due to the numerous specific scenarios, including customer service, investment research, insurance underwriting and claims, and internal compliance.
Question: Is the situation of large-scale model applications in the financial industry still in its infancy or about to explode?
Zhang Chi: I think it is about to explode. The key challenges for everyone are the shortage of computing power and the difficulty in model selection, both of which will improve rapidly this year.
Why release "Dianjin" at this time? Because as commercial models become more mature and computing power transitions to focus on reasoning and applying large models, these will no longer be the biggest issues. I believe the explosion is waiting for a sufficient number of large model applications to emerge, and corresponding financial industry scenarios will appear in every field.
Question: What value will Alibaba Cloud's large-scale model, released this time, bring to financial institutions in terms of revenue and sales?
Zhang Chi: Large models are not omnipotent and cannot solve all the needs of the financial industry through a general artificial intelligence approach. We hope to see more large model applications emerge and accumulate value over time through a combination of more scenarios, which may lead to corresponding chemical reactions.
With so many customers in the financial industry, large models need to integrate with many banks' existing digital platforms, cloud-native platforms, and data middle platforms in specific areas to better leverage their value.
For example, in the past, doing digital credit might involve making corresponding marketing decisions, such as which products to target at which demographics. It was difficult to truly know if a person had a willingness to take out a loan or make a deposit at the right time, leading to a strong sense of disconnection. However, with large models in the middle, they can enhance scattered content through reasoning and decision-making capabilities Question: What challenges might companies encounter in the field of large-scale models?
Zhang Chi: Many financial institutions used to like to build their own wheels, but they didn't really spend time optimizing the value of business application scenarios.
I don't want the industry to have to buy cards, hoard people, and reserve data for the sake of large models, spending 80% of their time on basic preparations, building platforms, foundations, and engineering work.
For financial institutions, Alibaba Cloud's "Dianjin" platform already has a good form and template, so there is no need for piecemeal debugging. You can first try to see if it fits your scenario, and then optimize and deploy this part of the content locally in a privatized manner.
On the other hand, for financial institutions, the hardest part is how to choose, not whether the business application of large models can run. They choose this component, that model, this platform, only to find that others have done better. There are many uncertainties and rapid technological iterations.
When we gradually shield this architecture and technological complexity layer by layer at cloud service providers, the choices behind it are all based on technical value and market value.
Question: Due to some compliance requirements, the financial industry may be more inclined towards private clouds. Now Alibaba Cloud emphasizes the construction of public clouds behind open source. How can these two be better combined?
Zhang Chi: The issue of private clouds must be faced, but in the context of large models, there will be a hybrid architecture.
Large models have training, development, inference, and operation states. Training is basically difficult, as it requires high energy and network requirements.
We found that many financial institutions need to dig up computing power from scratch, adjust models, build tool frameworks, provide model services, and develop applications, which requires a huge investment of manpower and time. In the case of a shortage of computing power, it is recommended to develop, test, and verify based on public cloud platforms. At least, there is no need to rebuild a set for developing large model applications.
In practice, many models can be quantified locally, and the inference can also be run on some small computing power. However, many financial industries require models of over hundreds of billions, and there are many challenges in running domestic GPU chips. We are constantly optimizing this.
Question: There are quite a few large model vendors now, and various fintech companies are all working on large models. What is the advantage of Alibaba Cloud?
Zhang Chi: I found that many financial institutions first optimize scenarios with OpenAI and then look for privatized large models, including some insurance companies' underwriting processes. They will turn some desensitized things into data and feed them into OpenAI.
Instead of doing this, why not use our domestic large models and cloud platforms to do this, as we can do more. Because OpenAI does not provide something that good. It also has some simple framework tools, but they do not fully meet the requirements of financial-grade.
Our large model capabilities can be honed in our e-commerce, logistics, and Ant Financial. So our own large model capabilities are also accumulated from our internal business practice capabilities At the same time, we have talent advantages and data advantages. We started to catch up with OpenAI as early as when they released GPT-3. Because there are many aspects to model training, it not only relies on machines but also on humans. "As many human efforts as there are, there is as much intelligence." It requires these scientists to continuously experiment in the field of model training to achieve success.
Question: Recently, large models have been engaging in price wars. Will the industry's leading effects become more concentrated? As a major player, how does Alibaba Cloud differentiate itself at the application level?
Zhang Chi: We will emphasize more on industry applications, which are more about attracting economies of scale from top institutions. For us, our differentiation lies in whether there is industry specificity.
In terms of future business models, not only ourselves but also the large model providers we cooperate with, when they truly provide services, there will be a premium for industry capabilities, such as financial-grade knowledge enhancement. We have already reduced costs to this extent, so when they design and develop, the costs will be even lower, enabling them to truly impact more high-value parts of the industry market, which can be better reflected.
Question: Now, companies like SenseTime, Huawei Cloud, and Alibaba Cloud are collaborating with financial institutions. What are the possibilities for the future of this collaborative competition?
Zhang Chi: Currently, financial large models are quite open. For example, Mini-max and Kimi are available in our tool framework, and customers can choose.
The challenge lies in computing power. NVIDIA has its own moat and advantages, and we are also developing our own domestic chips, but the costs of investment, compatibility, and adaptation are enormous.
The choice is more from the perspective of the scale of public clouds. Large models have to pick cards themselves, and when choosing, they need to select the situation where the speed, efficiency, and cost are optimal. It is difficult to do overall content optimization for a specific client of a financial institution