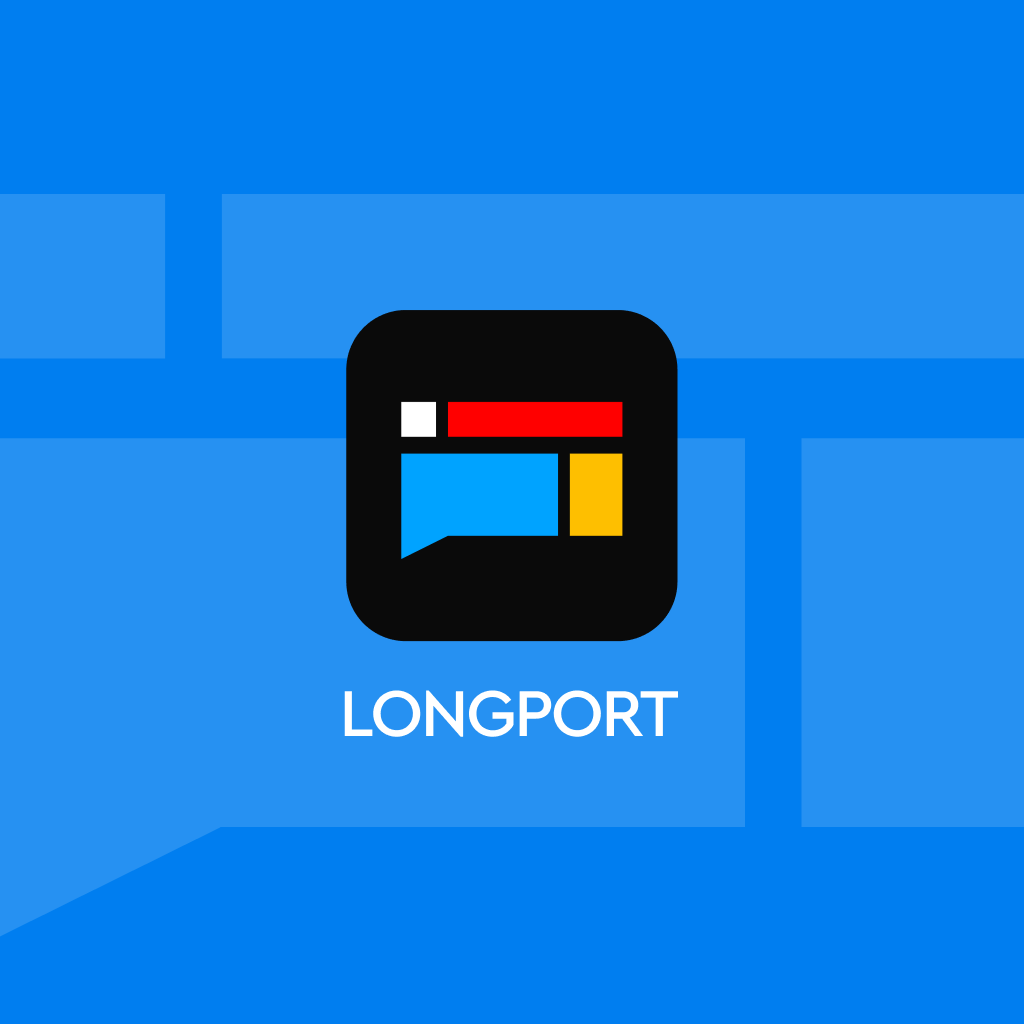
Deciphering the end-to-end mystery of Tesla
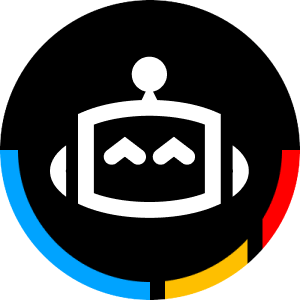
Tesla plans to invest over $1 billion by the end of 2024 to increase computing power to 100,000 PFLOPS to drive the development of end-to-end autonomous driving solutions. This signifies that end-to-end is triggering a new round of arms race, with winners typically being companies investing heavily in computing power. Meanwhile, other automakers such as Changan and Geely are also actively preparing for smart computing centers to meet the demand for computing resources in autonomous driving solutions. The specific details of Tesla's end-to-end autonomous driving solution are currently unclear, but many companies are following the trend closely to avoid being eliminated. Computing power has become a necessary condition for end-to-end, driving the fierce competition in smart computing centers
"What exactly is Tesla's end-to-end autonomous driving solution?"
At an end-to-end intelligent body seminar, someone threw this question to a group of end-to-end experts and scholars.
On-site, including Zhao Xing (Assistant Professor at Tsinghua Cross-Information Research Institute), Xu Chunjing (Chief Scientist of Huawei's Car BU Intelligent Driving AI), Wang Naiyan (Outstanding Scientist of Xiaomi Intelligent Driving), and Jia Peng (Vice President of Algorithm Development at Li Auto), no one could give a definite answer.
No one is clear about the specific model architecture of Tesla's FSD V12, but Tesla, with its own strength, has stirred up the tide of end-to-end.
We tried to piece together the rough outline of Tesla's end-to-end from Musk's speeches and Tesla's updates: from perception to decision controlled by a unified neural network, most likely based on generative AI, building a world model on the basis of the existing Occupancy model.
But the certainty captured from it is that the end-to-end solution is reaching a new peak in demand for cloud computing power.
As Musk has repeatedly stated: "The iteration of the FSD V12 end-to-end model is mainly constrained by cloud computing resources."
Therefore, Tesla chooses to invest heavily in computing power, planning to invest over $1 billion in the DOJO supercomputing center by the end of 2024, with the goal of increasing total computing power to 100,000 PFLOPS.
If computing power is a necessary condition for end-to-end, this means that end-to-end is sparking a new round of arms race, where the winner is often the one who exerts great strength.
At the same time, just as no one knows exactly how Tesla's end-to-end is achieved, everyone is just aiming at the direction of the surging tide and rushing towards it.
So, like a spring breeze overnight, end-to-end solutions are everywhere, and everyone is keeping up with the pace, not wanting to be left out.
End-to-End Autonomous Driving, Great "Strength" Leads to Miracles
End-to-end autonomous driving, based on the AI modelized main path, with its huge demand for training computing resources, is bound to fuel the flames of computing power consumption.
The intelligent computing center has entered an era of enclosure, and a competition related to computing power has begun.
Here, car companies such as Tesla, Changan, Geely, etc., are sparing no effort to prepare for the intelligent computing center, either choosing to build their own or cooperating with third parties.
Tesla's DOJO intelligent computing center is expected to reach a total computing power of 100 EFLOPs (100,000 PFLOPS) by October 2024, equivalent to the total computing power of about 300,000 NVIDIA A100s.
Domestic car companies are also striving to catch up in computing power, with Geely, Changan, and new forces like "Weixiaoli" not falling behind It is worth mentioning that Nio has partnered with Tencent to establish the Smart Computing Center. Although the specific strength of its supercomputing center has not been announced yet, Li Bin once described Nio's layout in terms of computing power as "insane" and stated that it will still be the global leader in the next one or two years.
On the other hand, intelligent driving suppliers represented by Huawei, SenseTime, and Momenta are also not falling behind.
Huawei's Car BU Cloud Intelligent Computing Center's Qiankun ADS 3.0 has reached 3500 PFLOPS in terms of computing power, with a training data volume of 30 million kilometers per day. Based on the total global road length of approximately 64 million kilometers, the system can fully cover it in 2.1 days.
SenseTime Technology revealed in its latest financial report that the number of GPUs in its Smart Computing Center has reached 45,000, with a total computing power scale of 12000 PFLOPS, doubling from the beginning of 2023. Additionally, Momenta, in collaboration with Volcano Engine, launched the Smart Computing Center "Xuehu Oasis" with a computing power of up to 670 PFLOPS.
Clearly, the construction of Smart Computing Centers has become a standard for end-to-end autonomous driving, and the demand for computing power is growing at an extremely rapid rate.
"End-to-end intelligent driving companies without Smart Computing Centers are unqualified," a expert from Momenta bluntly stated. The more computing power, the more significant improvements in model iteration efficiency, iteration speed, and repair efficiency under various circumstances.
Shi Jianping, Vice President of Intelligent Driving at SenseTime, also mentioned that high computing power means a wide range of application space, allowing for more attempts, trial and error, which makes it more likely to develop stronger end-to-end models.
So does this mean that achieving end-to-end intelligent driving requires great efforts to work miracles?
Interestingly, the industry has presented two development paths in response to this answer:
- One side tends towards "brute force computing" that emphasizes heavy investment in computing power;
- The other side focuses on "craftsmanship" that delves deep into algorithms.
Indeed, the industry's consensus on the three elements of intelligent driving (algorithms, data, computing power) is that they complement each other, and any shortcomings in one aspect can trigger a domino effect.
However, based on this foundation, there are some differences in which aspect needs to be strengthened the most at present.
Advocates of brute force computing believe that the algorithms used by various companies are essentially the same, and the key lies in how to efficiently train the data in the supercomputing center.
An industry professional pointed out that with feasible end-to-end algorithm architectures already publicly available in academia, and with continuous updates on cutting-edge advancements, the industry can fully refer to research results from academia for mass production and practical experiments. This requires accumulating sufficient strength in computing power and data scale at the current stage.
But there is another voice mixed in. They believe that deepening algorithms is currently a more urgent breakthrough method to achieve end-to-end intelligent driving.
Yuan Rongqixing expressed to AutoHeart that the competition in computing power centers is just one aspect, but what is more important at the current stage is to build a network model that satisfies the Scalling law.
Scalling law refers to the law of scale, where as the model scale increases (including the number of parameters, data scale, and computing resources), the model's performance will also improve accordingly In other words, in order for the law of scale to take effect, the key point to consider first is the optimization problem of the model, which is the driving force for miraculous achievements in the future.
Ultimately, there is no absolute distinction between the two paths, as each company has different end-to-end strategic planning and capital strength.
However, judging from the actions of leading car companies such as Tesla and Huawei reinvesting in supercomputing centers, the higher the computing power, the higher the ceiling of end-to-end intelligent driving effects will be raised, meaning the upper limit will be increased.
So, how much computing power in a supercomputing center can support end-to-end intelligent driving?
According to the "End-to-End Autonomous Driving Industry Research Report" released by Chentao Capital, most companies indicate that 100 high-performance GPUs can support one end-to-end model training session, but this is unlikely to support the solution to the mass production stage.
MoMo Intelligent Mobility believes that, based on the need for continuous algorithm iteration, the initial stage of end-to-end requires 1000 GPUs.
But as for how to measure the upper limit, there is no consensus.
The industry unanimously believes in acting within one's means. After all, the giant Tesla stands in front of many competitors.
It is reported that Tesla plans to increase the number of NVIDIA GPU H100 to over 85,000 this year, reaching the same level as Google and Amazon, a level that domestic companies can only dream of.
After all, one H100 currently sells for between $25,000 and $40,000, which means Tesla will have to invest at least $2 billion this year.
Without a strong financial foundation, not everyone can afford to "play" like this. Because Tesla's mission is to globalize embodied intelligence, with goals including Robotaxi, intelligent robots, etc., the difficulty of solving problems involves a new level.
Therefore, Tesla's bold moves are based on the alignment of financial resources, goals, and data scale, and other companies do not need to emulate it and pursue blindly high computing power.
For domestic intelligent driving companies, the current goal is to solve the mass production of urban NOA and achieve advanced autonomous driving.
MoMo Intelligent Mobility states that to achieve nationwide availability, 2000-5000 GPUs are already sufficient.
However, as the goals continue to advance from L2 to L3, L4, and even L5, the demand for computing power will continue to rise.
In any case, the end-to-end wave has indeed triggered a new reshuffle, whether it is data scale, algorithm structure, or computing power requirements, leading companies that master the technological core to the forefront.
End-to-End Puzzle: Who is the Real End-to-End?
The end-to-end trend is creating a new wave of internet memes Everyone wants to catch the express train end-to-end. Even if the technology is not keeping up, the high ground of publicity must be occupied.
Interestingly, in the scenario of "you are also end-to-end, I am also end-to-end", it is difficult to burst the true and false bubbles.
Fundamentally, the reason lies in the fact that the end-to-end implementation path has not been unified, and each company has the right to speak.
Now the definition of end-to-end can be divided into broad and narrow senses.
Broad sense emphasizes that end-to-end is the lossless transmission of information, without information loss caused by artificially defined interfaces, and can achieve data-driven holistic optimization.
The narrow sense of end-to-end only emphasizes a single neural network model from sensor input to planning and control output.
In other words, as long as the broad sense standard is met, it can be called end-to-end. Therefore, various end-to-end intelligent driving enterprises have different implementation forms from input to output. The mainstream solutions now have the following three types:
-
Perception and cognition modularization. Splitting the large model into two stages of perception and cognition (prediction decision planning), training them in series. Represented by Huawei Qiankun ADS 3.0, its perception part uses the GOD large perception network, and the cognition part uses the PDP network to achieve an end-to-end network.
-
Modular end-to-end. Linking all models of intelligent driving together and training them in a unified way. Represented by OpenDriveLab's UniAD (2023), it completes global optimization through gradient propagation across modules (perception prediction planning).
-
Single neural network. That is, the narrow sense of end-to-end concept. Using a large model covering input to output for direct training. Represented by Wayve, its generative world model GAIA-1, visual-language-action model LINGO-2 may be important foundations for future One Model end-to-end.
It is worth mentioning that in order to keep up with the trend of end-to-end, companies that stand on top of traditional rule-based algorithms cannot start over for a while, so they follow a progressive technical path.
The report also clearly indicates the four stages of evolution of autonomous driving architecture: perception "end-to-end", decision planning modularization, modular end-to-end, single model (One Model) end-to-end.
Image Source: Chentao Capital "End-to-End Autonomous Driving Industry Research Report"
In other words, get on the perception model first, then model the planning, and finally link them together for end-to-end training. This is a relatively smooth transitional form.
Ren Shaoqing, Vice President of Intelligent Driving Research and Development at Nio, also believes that the large models of autonomous driving need to be broken down into several levels. The first step is modularization, the industry has basically completed the perception modularization, but the leading companies have not fully done well in the planning and control modularization. The second step is end-to-end, removing the artificially defined interfaces between different modules, and the third step is the large model Of course, the path to end-to-end can be smooth or start over.
Xpeng emphasized on AI DAY that they have unloaded their burdens and implemented end-to-end large models.
Haomo also mentioned, "If you have enough courage and determination to reconstruct a system, efficiency may be higher."
Therefore, the choice of path and method depends on practical considerations.
However, due to the different implementation paths, progress, and publicity efforts of each company, the diverse opinions on end-to-end have indeed created a puzzle.
An awkward point is that when trying to distinguish the authenticity of end-to-end from some obvious features, it is found that none of them work.
For example, the BEV+Transformer architecture, which many companies consider as a standard for perceptual modeling, does not represent a binding relationship. It can only be said that this is a better implementation method for perceptual models at present.
Tesla's pure visual route and Huawei's fusion route with lidar can both be called end-to-end, which are just different choices made by different companies.
Although some companies emphasize that without high-precision maps, end-to-end models cannot be achieved.
But more voices tend to believe that there is no absolute connection between the two.
Shi Jianping emphasized that abandoning high-precision maps is not a prerequisite for end-to-end. Although SenseTime has achieved "no map" now, in order to make the interaction more user-friendly, they are also preparing to add navigation maps.
Especially considering factors such as the complexity of model training, the safety of mass production implementation, and the cost of end-to-end solutions, both the pure visual and lidar routes are technical choices made by various companies.
The root cause of the inability to prove end-to-end lies in the fact that end-to-end autonomous driving emphasizes the gradient transmissibility and global optimization in structure, which is just a training method.
It is often confused with another term, "large model."
Industry professionals unanimously point out that these are two different dimensions of concepts. Large models focus on the number of model parameters and emergent capabilities. Currently, large models provide solutions for end-to-end implementation, but end-to-end is not necessarily based on large models.
So, returning to the initial question, how to distinguish between true and false end-to-end?
The answer is, either dig into the code or observe the experience.
The former looks at how the code is written and whether it achieves lossless information transmission from input to output. Obviously, this is not very practical.
The latter is to judge the level of autonomous driving during the landing verification phase, whether it can handle various corner cases like an "experienced driver." This is the only reliable way to distinguish.
Some industry professionals have expressed that "after the end-to-end solution is implemented, there will be a significant leap in autonomous driving level. If the effect is similar, it means the end-to-end solution is fake."
End-to-end is not necessarily the final solution, but it is the optimal solution today
From the UniAD paper published by the Shanghai Artificial Intelligence Laboratory winning the best paper award at CVPR 2023, to the launch of Tesla FSD V12, to the autonomous driving company Wayve receiving a $1 billion financing, under the "conspiracy" of academia, industry, and capital, end-to-end autonomous driving has initiated a new round of industrial revolution NVIDIA Automotive Vice President Wu Xinzhou believes that end-to-end is the final movement of the smart driving trilogy.
Xiaopeng CEO He Xiaopeng also stated that end-to-end will bring disruptive changes to smart driving.
However, in the roundtable debate on end-to-end vs. traditional modular design at the end-to-end intelligent body seminar, the final conclusion was that end-to-end design did not completely crush traditional modular design. There are still cold thoughts about verification, implementation, and mass production.
So it can only be said that end-to-end is not necessarily the ultimate solution to the endgame of smart driving, but it is currently the best solution. It can handle extreme cases that traditional paths find difficult to solve, and represents a way to reduce reliance on manual coding and a more efficient approach.
Based on this path, perhaps it can lead to a higher stage of smart driving.
Now, including academia, car companies, and smart driving suppliers, everyone is heading towards end-to-end.
From the perspective of the main segments, the emphasis and division of roles in the development path of end-to-end smart driving are still somewhat different.
Academia focuses on exploring algorithm architecture and technical paths, as seen in the BEVFormer architecture open-sourced by the Shanghai Artificial Intelligence Laboratory, which is a common visual perception algorithm structure today; and Tsinghua MARS Lab was the first to publish a "mapless" autonomous driving solution, achieving the integration of autonomous driving map memory, update, and perception.
The eruption of academic ideas is projected into the industry, thereby driving the implementation and development direction of technology. For example, Tsinghua MARS Lab's BEV detection algorithm, BEV tracking algorithm, etc., are widely used in Li Auto's products.
However, for smart driving suppliers and car companies linking to the commercial end, besides the systematic nature and feasibility of the solution, more importantly, it is about seizing the advantage in the race against time.
Currently, many smart driving suppliers have launched their own end-to-end mass production solutions in the past two years.
In April last year, Momenta released the smart driving generative large model DriveGPT (Xuehu·Hairuo), which is an important technical carrier for achieving end-to-end smart driving.
As of May this year, over 20 vehicles equipped with Momenta's HPilot smart driving have surpassed 160 million kilometers of user-assisted driving mileage.
Xiaoma Zhixing also launched an end-to-end smart driving model in August last year, which has been synchronized into L4 level autonomous driving taxis and L2 level assisted driving passenger cars.
In April this year, DeepRoute demonstrated the upcoming mass-produced advanced smart driving platform DeepRoute IO and the end-to-end solution based on DeepRoute IO During the same period, SenseTime Jueying launched UniAD for mass production, achieving high-precision map removal, and also released the next-generation autonomous driving technology DriveAGI, which is an automatic driving solution built on a multimodal large model.
Obviously, the mass production and implementation of end-to-end solutions are already imminent.
Especially after Tesla's FSD signal was released in China, car companies are even more restless.
XPeng announced the mass production of end-to-end solutions in May, while Nio and Li Auto have also accelerated their plans to implement end-to-end models in the first half of this year.
However, 2024 can only be reluctantly referred to as the first year of mass production and implementation of end-to-end solutions, with the real large-scale implementation expected in 2025.
SenseTime Jueying stated that a more reasonable time for the implementation of end-to-end solutions will be in the second half of next year, reaching a state of mass production introduction. Because for end-to-end technology solutions to mature and go online, they need to undergo extensive reliability verification.
An industry insider specializing in end-to-end solutions also pointed out, "End-to-end implementation is feasible, but the actual effects after implementation are another matter. It will be very difficult to achieve the same effects as Tesla within this year."
Nevertheless, end-to-end solutions have indeed sparked a new competition to test the strength of autonomous driving technology, and now the competition has entered the second half.
The academic and industry sectors are racing while also collaborating to explore the implementation stage of end-to-end solutions together.
Currently, the exploration direction presents three major trends, mainly corresponding to the three major challenges of end-to-end implementation, namely:
-
How to control costs for end-to-end solutions?
-
How to address the black box issue for end-to-end solutions?
-
How to standardize verification for end-to-end implementation?
The first is the optimization of end-to-end solutions.
As a new technological path, end-to-end solutions have high requirements for large computing power, big data, and advanced algorithms, setting a high threshold for players. Most companies find it difficult to have the determination and strength of Tesla to invest billions, or even tens of billions of dollars, all in end-to-end solutions.
Moreover, considering the trial and error costs of new things, careful consideration is needed in algorithm architecture to balance efficiency and cost.
According to Momenta CEO Cao Xudong, Momenta's approach is to divide the end-to-end architecture into two branches, one being the end-to-end large model, analogous to human long-term memory; the other branch is the perception and cognition stage, analogous to human short-term memory.
By first verifying the correctness and data effectiveness in the form of short-term memory, and then transferring to the branch of the end-to-end large model, efficient training is ensured. Compared to directly applying end-to-end models, this training method can reduce costs by 10-100 times.
The second is the safety net for end-to-end solutions.
End-to-end autonomous driving is akin to human-like driving, but when it comes to actual implementation, there is still the issue of the inexplicability of the black box that urgently needs to be addressed, especially when facing the complex urban road conditions in China, where complete safety is difficult to guarantee.
For example, Li Auto has introduced a dual-system solution to provide a safety net for end-to-end solutions. System 1 adopts end-to-end technology, corresponding to normal driving capabilities; System 2 carries the VLM model, corresponding to generalization capabilities.
This means that System 1 only needs to handle simple road condition issues, while System 2 can handle complex logical reasoning and unknown problems. This system can enhance the spatial understanding ability of large models and avoid the inference speed issues of large models Third, end-to-end verification.
The implementation of an end-to-end solution first needs to go through a mature verification process. However, direct vehicle verification is obviously too costly, and open-loop test conditions based on data re-injection (offline data regression testing) do not match the interactivity required for end-to-end intelligent driving verification.
Therefore, implementing closed-loop testing verification of models based on simulators has become a feasible path for current verification. The report points out that the development of closed-loop simulation tools is a necessary condition for on-board end-to-end implementation.
Currently, the industry is actively exploring closed-loop simulation tools:
-
The academic community generally uses CARLA as a closed-loop simulation simulator for end-to-end development;
-
Intelligent driving generative AI company Lightwheel Intelligence combines generative AI to develop a data and simulation end-to-end solution for algorithm research and development;
-
Another similar company, Excellent Technology, has also created a large multimodal visual generative model called the "world model".
Although the "snow in front of the door" for the implementation of end-to-end has not been completely cleared, the industry's confidence in end-to-end has reached a peak.
After all, the emergence of end-to-end has led the field of artificial intelligence from being primarily "rule-driven" to crossing over to being driven by "deep learning", representing a technological leap in a chasm-like manner.
Intelligent driving, undoubtedly, has become the important gateway in the physical world to experience and demonstrate this transformation first.
Author: JiaYi Liu, Article Source: Autohome, Original Title: "Deciphering the End-to-End Puzzle: Computing Power Miracle, Diverse Architecture, and Implementation Challenges"