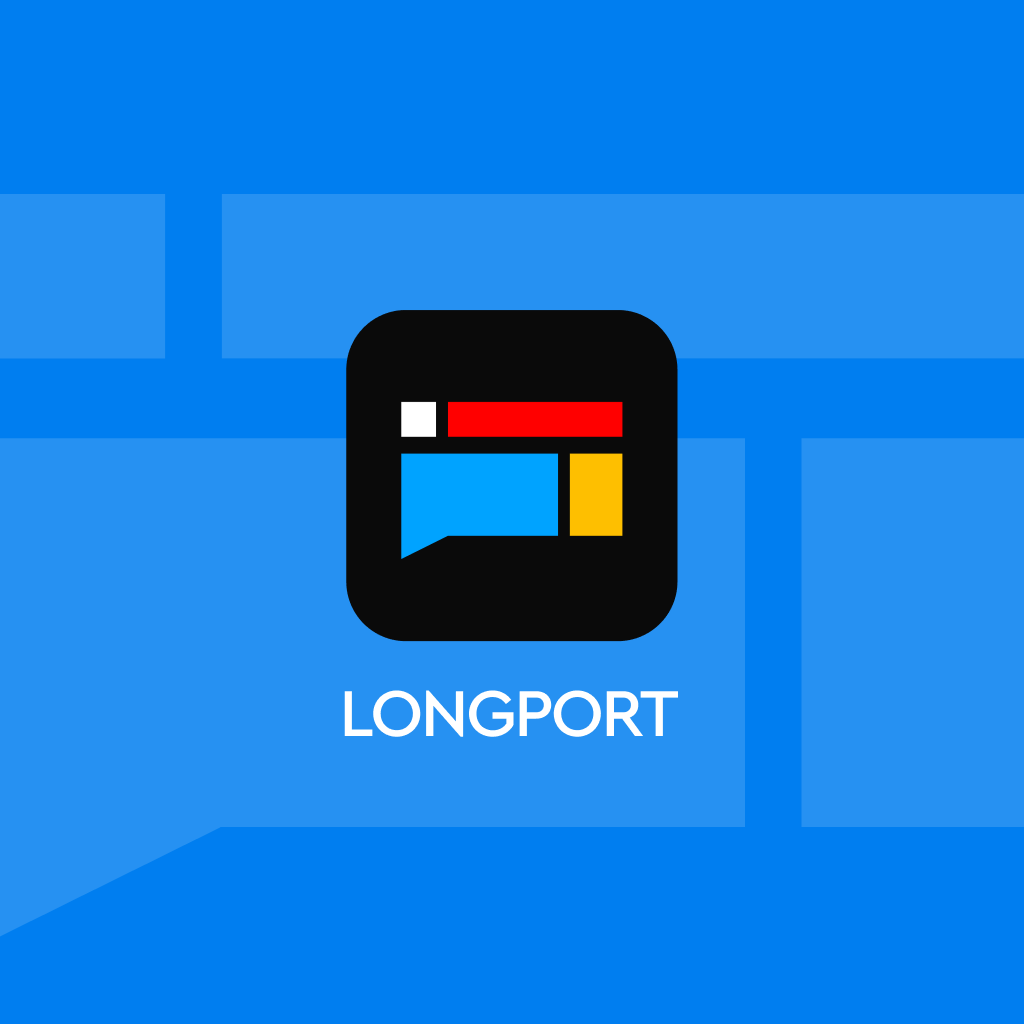
NVIDIA GPU, the alarm bell rings
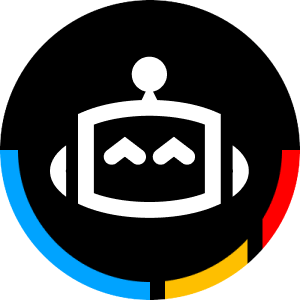
NVIDIA's AI chip supply is facing a significant bottleneck, with regulatory agencies concerned that its market dominance may face restrictions. AI companies need to earn around $600 billion to cover their infrastructure costs, but revenue growth has not materialized yet
Shortly after the news that France will launch an antitrust investigation against NVIDIA, more bad news has emerged.
According to Bloomberg, European Commissioner for Competition Margrethe Vestager warned that NVIDIA's AI chip supply is facing a "huge bottleneck," but regulatory authorities are still considering how to address this issue.
"We have been asking them questions, but this is still just preliminary questions," she told Bloomberg during her trip to Singapore. So far, "the conditions for regulatory action are not yet met."
Since NVIDIA has become the biggest beneficiary of the AI spending boom, regulatory authorities have been keeping a close eye on it. Its Graphics Processing Units (GPUs) are favored by data center operators for their ability to handle the massive amounts of information required to develop AI models.
Chips have become one of the hottest commodities in the tech industry, with cloud computing providers competing for these chips. It is estimated that the high demand for NVIDIA's H100 processor has helped them secure over 80% of the market share, surpassing competitors Intel and AMD.
Despite the supply constraints, Vestager suggests that the secondary market for AI chip supply may help stimulate innovation and fair competition.
However, she mentioned that dominant companies in the future may face certain behavioral restrictions.
"If you have this kind of dominant position in the market, there are things you cannot do that small companies can do," she said. "But other than that, as long as you do your business and respect that, you're fine."
The $600 Billion "Big Challenge"
Despite the significant investments by tech giants in AI infrastructure, the revenue growth from artificial intelligence has not materialized yet, indicating a significant gap in the ultimate user value of the ecosystem. In fact, according to analysis by Redpoint Ventures analyst David Cahn, AI companies need to generate around $600 billion annually to cover the costs of their AI infrastructure (such as data centers).
Last year, NVIDIA's data center hardware revenue reached $47.5 billion (with most hardware used for computing GPUs for AI and HPC applications). Companies like AWS, Google, Meta, Microsoft, etc., have invested heavily in AI infrastructure for applications like OpenAI's ChatGPT in 2023. However, can they recoup this investment? David Cahn believes this could mean that we are witnessing the growth of a financial bubble.
According to David Cahn's calculations, the $600 billion figure can be derived through some simple mathematical calculations All you have to do is to multiply Nvidia's utilization revenue forecast by 2 to reflect the total cost of AI data centers (with GPUs accounting for half of the total ownership cost, and the other half including energy, buildings, backup generators, etc.). Then you multiply by 2 again to reflect a 50% gross margin for GPU end users (for example, startups or enterprises purchasing AI computing from Azure, AWS, or GCP also need to make a profit).
Let's see what has changed since September 2023 (at that time, he considered artificial intelligence a $200 billion challenge)?
1. Shortages have subsided: The end of 2023 was the peak of GPU shortages. Startups were calling venture capital firms, calling anyone willing to talk to them, seeking help to get GPUs. Today, these concerns are almost completely eliminated. For most people I've talked to, getting GPUs in a reasonable delivery time is relatively easy now.
2. GPU inventory continues to grow: Nvidia reported in the fourth quarter that about half of its data center revenue comes from large cloud providers. Microsoft alone could account for about 22% of Nvidia's fourth-quarter revenue. Large-scale capital expenditures are reaching historic levels. These investments are a major theme in the first quarter of 2024 for large tech companies, with CEOs effectively telling the market, "Whether you like it or not, we will invest in GPUs." Hoarding hardware is not a new phenomenon, and once the inventory becomes large enough that demand drops, it becomes a catalyst for reset.
3. OpenAI still holds the largest share of AI revenue: The Information recently reported that OpenAI's revenue is now $3.4 billion, up from $1.6 billion at the end of 2023. While we have seen a few startups with revenue scales of less than $100 million, the gap between OpenAI and other companies remains significant. Besides ChatGPT, how many AI products do consumers really use today? Think about how much value you get for $15.49 a month from Netflix or $11.99 a month from Spotify. In the long run, AI companies need to provide significant value to consumers to continue to pay.
4. The $125 billion gap has now turned into a $500 billion gap: In the final analysis, I generously assume that Google, Microsoft, Apple, and Meta can each generate $10 billion annually from new AI-related revenue. I also assume that Oracle, ByteDance, Alibaba, Tencent, X, and Tesla each have $5 billion in new AI revenue annually. Even if this is still true, and we add a few more companies to the list, the $125 billion gap will now turn into a $500 billion gap Not over yet - B100 is coming: Earlier this year, Nvidia announced the launch of the B100 chip, which offers a 2.5x performance improvement at only a 25% increase in cost. I anticipate this will lead to a significant surge in demand for NVDA chips. Compared to the H100, the B100 shows significant improvements in cost and performance, and with everyone looking to buy the B100 later this year, another supply shortage is likely to occur.
When discussing GPUs previously, one of the main counterarguments David Cahn received was that "GPU capital expenditure is like building a railway", eventually the train will come and the destination will arrive - new agricultural exports, amusement parks, shopping centers, etc.
David Cahn stated that he agrees with this to some extent, but he believes this argument overlooks a few points:
1. Lack of pricing power: In the case of physical infrastructure construction, the infrastructure being built has some intrinsic value. If you have a track between San Francisco and Los Angeles, you may have some kind of monopoly pricing power because there can only be so many tracks laid between A and B. In the case of GPU data centers, pricing power is much smaller. GPU computing is increasingly becoming a commodity measured by the hour. Unlike CPU clouds that become oligopolies, new entrants building dedicated AI clouds continue to enter the market. In the absence of a monopoly or oligopoly, enterprises with high fixed costs + low marginal costs almost always see price competition down to marginal costs (e.g. airlines).
2. Investment waste: Even in the railway industry, as well as many new technology industries, speculative investment frenzies often lead to high levels of capital waste. "The Engines that Moves Markets" is a great textbook on technology investment, with its main point (indeed, focusing on the railway industry) being that many people suffer heavy losses in speculative technology waves. It's hard to pick winners, but picking losers (in the case of the railway industry, canals) is much easier.
3. Depreciation: From the history of technological development, we know that semiconductors tend to get better and better. Nvidia will continue to produce better next-generation chips, such as the B100. This will accelerate the depreciation of the previous generation chips. Since the market underestimates the rate of improvement of the B100 and next-generation chips, it overestimates the value of the H100 purchased today in 3-4 years. Similarly, physical infrastructure does not have this similarity, it does not follow any curve like "Moore's Law", so the relationship between cost and performance continues to improve.
4. Winners and losers: I believe we need to carefully study the winners and losers - in times of infrastructure overbuilding, there will always be winners. Artificial intelligence is likely to be the next wave of transformative technological waves, and the decline in GPU computing prices is actually beneficial for long-term innovation and for startups. If David Cahn's predictions come true, it will mainly harm investors. Founders and company builders will continue to develop in the field of artificial intelligence - they are more likely to succeed because they will benefit from lower costs and the experience accumulated during this experimental period V. Artificial Intelligence Will Create Enormous Economic Value. Companies that focus on providing value to end users will reap substantial rewards. We are in the midst of a technological wave that may define a generation. Companies like NVIDIA play a crucial role in driving this transformation and are likely to play a key role in the ecosystem for a long time to come.
However, David Cahn also reiterated that speculation is part of technology, so there is nothing to fear. Those who keep a clear head at this moment have the opportunity to create extremely important companies. But we must ensure not to believe in the delusion that has spread from Silicon Valley to the entire country and even the world, thinking that we will all get rich quickly because AGI will arrive tomorrow, and we all need to stockpile the only valuable resource, which is the GPU.
"In fact, the road ahead will be long. It will have its ups and downs. But it is almost certain that it is worth it," emphasized David Cahn.
Potential Challengers
Although this has been discussed many times, it seems that there is a conclusive argument. As Daniel Newman, CEO of Futurum Group, said, "Currently, there is no archenemy of NVIDIA in the world."
The reasons are as follows: NVIDIA's Graphics Processing Units (GPUs) were originally created in 1999 for ultra-fast 3D graphics in PC video games, and later proved to be very suitable for training large-scale generative AI models. Models driven by companies such as OpenAI, Google, Meta, Anthropic, and Cohere are becoming larger in scale, requiring a large number of AI chips for training. For years, NVIDIA's GPUs have been considered the most powerful and in-demand.
Of course, these costs are not cheap: training top generative AI models requires tens of thousands of highest-end GPUs, with each GPU priced at $30,000 to $40,000. For example, Elon Musk recently stated that his company xAI's Grok 3 model needs to be trained on 100,000 top NVIDIA GPUs to become "something special," which will bring NVIDIA over $3 billion in chip revenue.
However, NVIDIA's success is not just the result of chips, but also the software that makes the chips easy to use. NVIDIA's software ecosystem has become the preferred choice for a large number of AI-focused developers, who have little incentive to switch. At the company's annual shareholder meeting last week, NVIDIA CEO Jensen Huang called the company's software platform CUDA (Compute Unified Device Architecture) a "virtuous cycle." With more users, NVIDIA has the ability to invest more funds to upgrade the ecosystem, attracting more users.
In contrast, NVIDIA's semiconductor competitor AMD controls about 12% of the global GPU market share, and the company does have competitive GPUs and is improving its software, Newman said However, although it can provide an alternative for companies that do not want to be bound by Nvidia, it lacks the existing developer user base who find CUDA easy to use.
In addition, large cloud service providers such as Amazon's AWS, Microsoft Azure, and Google Cloud all produce their own proprietary chips, but they do not intend to replace Nvidia. Instead, they hope to have a variety of AI chips to choose from to optimize their data center infrastructure, reduce costs, and sell their cloud services to the widest potential customer base.
J. Gold Associates analyst Jack Gold explained, "Nvidia has the early momentum, and when you build a rapidly growing market, it's hard for others to catch up." He noted that Nvidia has done well in creating a unique ecosystem that others do not have.
Matt Bryson, Senior Vice President of Stock Research at Wedbush, added that it will be particularly difficult to replace Nvidia's chips used for training large-scale AI models. He explained that most of the current spending on computing power is flowing into this area. "I don't think this dynamic will change in the near future," he said.
However, an increasing number of AI chip startups, including Cerebras, SambaNova, Groq, as well as the latest Etched and Axelera, see an opportunity to grab a share from Nvidia's AI chip business. They focus on meeting the specific needs of AI companies, especially in the area of "inference," which involves running data through already trained AI models to get model outputs (for example, each answer from ChatGPT requires inference).
For example, just last week, Etched raised $120 million to develop a dedicated chip for running transformer models called Sohu. Transformer models are an AI model architecture used by OpenAI's ChatGPT, Google's Gemini, and Anthropic's Claude. The chip will be produced by TSMC using its 4nm process, and the company claims to have obtained high-bandwidth memory and server supply from "top suppliers," without disclosing their names. Etched also claims that Sohu is "an order of magnitude faster and cheaper" than Nvidia's upcoming Blackwell GPU, with an eight-chip Sohu server capable of processing over 500,000 Llama 70B tokens per second. This judgment was made based on the released Nvidia H100 server MLperf benchmark data, which showed that an eight-GPU server can process 23,000 Llama 70B tokens per second. Etched CEO Uberti stated in an interview that one Sohu server will replace 160 H100 GPUs Dutch startup Axelera AI is developing chips for artificial intelligence applications. The company announced last week that it has raised $68 million in funding to support its ambitious growth plans. Based in Eindhoven, the company aims to become the European version of Nvidia, offering AI chips that are reportedly 10 times more energy-efficient and 5 times cheaper than competitors. The core innovation of Axelera is the Thetis Core chip, which can perform an astonishing 260,000 calculations in one cycle, compared to regular computers that can only perform 16 or 32 calculations. This capability makes it ideal for AI neural network calculations, primarily vector matrix multiplication. Their chips provide high performance and availability at a fraction of the cost of existing market solutions, which can make AI more accessible for a wider range of applications and users.
Meanwhile, Groq, which focuses on running models at lightning speed, is reportedly raising new funds at a valuation of $2.5 billion, and Cerebras is said to have secretly filed for an IPO shortly after releasing its latest chip, claiming that the chip can train AI models 10 times larger than GPT-4 or Gemini.
These startups may initially focus on a niche market, such as providing more efficient, faster, or cheaper chips for certain tasks. They may also focus on specialized chips for specific industries or AI devices like personal computers and smartphones. "The best strategy is to carve out a niche market rather than trying to conquer the world, which is what most of them are trying to do," said Jim McGregor, Chief Analyst at Tirias Research.
Therefore, a more pertinent question may be: how much market share can these startups capture alongside cloud providers and semiconductor giants like AMD and Intel? This remains to be seen, especially as the chip market for running AI models or inference is still relatively new.
References
https://www.bloomberg.com/news/articles/2024-07-05/nvidia-ai-chips-are-huge-bottleneck-eu-s-vestager-warns
https://www.sequoiacap.com/article/ais-600b-question/
https://fortune.com/2024/07/02/nvidia-competition-ai-chip-gpu-startups-analysts/