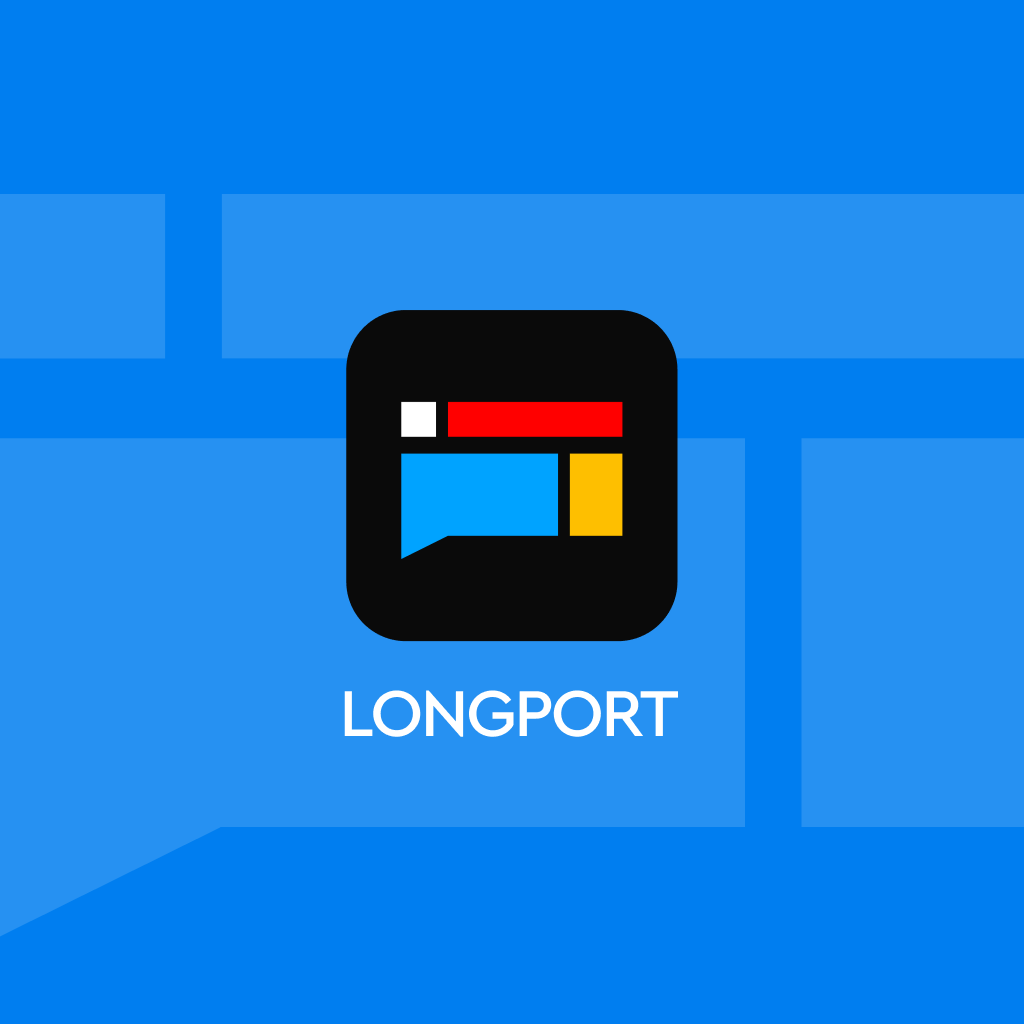
Microsoft CTO: How far can the "Scaling Law" of AI large models go?
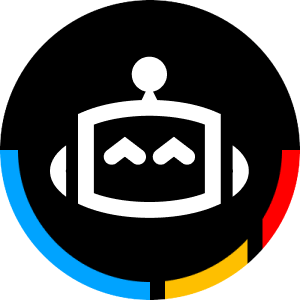
Kevin Scott stated that the most important advancements in the field of artificial intelligence over the past 20 years have been related to "scale"; the potential of OpenAI lies in its future ability to become the foundation for building AI platforms; the quality of data is more important than quantity
Author: Li Xiaoyin
Source: Hard AI
In the AI era, Large Language Models (LLMs) are prevalent.
As early as 2020, OpenAI proposed a law in a paper: Scaling law. This law states that the final performance of large models is mainly related to the size of computational resources, model parameters, and training data, rather than the specific structure (number of layers/depth/width) of the model.
Since then, OpenAI has risen in the AI field, with many startups and even tech giants taking this law as a guide.
With the continuous development of AI technology, more and more people have begun to question the Scaling law. A mainstream opposing view is that this training logic may lead large models to become servants of data, moving further away from "humanity".
Against this backdrop, on July 9th, Pat Grady and Bill Coughran from Sequoia Capital, along with Microsoft's CTO Kevin Scott, held a discussion on AI themes, Microsoft's AI strategy, progress in cooperation with OpenAI, and the future development direction of large models.
After watching the interview video, this article summarizes Kevin Scott's insightful views as follows:
-
Microsoft's AI strategy is to build a platform, a system, rather than a substitute technology.
-
The most important advances in the field of artificial intelligence in the past 20 years have been related to "scale" (especially data scale and computing power scale). We have focused our investments on scaling up.
-
The Scaling law still applies to the current industry format - while expanding large models, the marginal benefits have not decreased.
-
Microsoft values the potential of OpenAI, as with the expansion of models, OpenAI is expected to become the foundation for building a platform.
-
The quality of data is more important than the quantity, as it can provide templates for AI training algorithms and an economic framework for future collaborations.
-
Obtaining valuable training data for training, and then reasoning the model, will lead to two forms of business models. We are exploring a business model that combines AI recommendations with advertising.
-
The next generation of large models is about to emerge, cheaper and more powerful than before.
Here are the highlights of the interview:
Host: Kevin Scott, Microsoft's Chief Technology Officer. We are pleased to have known Kevin for decades, dating back to his time at Google, where he intersected with our partner Bill Coughran. Bill will join us for a special program today, and we hope you have a great time.
Kevin Scott: Glad to be here.
Host: First of all, I know you've talked about this before, but for our listeners who may not be familiar with your story - how did a kid from rural Virginia become Microsoft's CTO? Who would have thought?**
Kevin Scott: I do think this is an experience that cannot be replicated. When I look back on my life story, it just happened at the right time, in the right place. I am now 52 years old, so when the PC revolution began to erupt, I was 10 to 12 years old, and at that time, I was like when you are still a child, trying to figure out what you are doing and where your interests lie.
In general, an objective lesson is: if you happen to be interested, and very motivated to learn more, do more things, and develop rapidly, you may end up in a reasonable place. I was very interested in computers and was the first college student in my family, so I was the first to obtain a bachelor's degree. I majored in computer science and minored in English literature. So at some point, I would think about where to go and what to do after I got my bachelor's degree.
I was broke, tired of being caught all the time, so I chose the pragmatic path. I thought having a Ph.D. in English literature would be great, but I chose computer science between the two degrees, and for a while, I thought I would become a computer science professor. I decided to become a compiler, optimization, and programming language person, and after years of graduate study, I almost persisted to the end, I think, I don't think I want to be a professor. Writing a paper through six months of hard work to improve some synthetic benchmarks by 3% - I don't think this is a way for me to make a big impact in the world. And I don't want to keep repeating this work.
So in 2003, I applied to Google, and I received an email from a person: Craig Neubauer Manning, who had just opened Google's first remote engineering office in New York. I had a great interview experience, I don't know if it was intentional or just my luck, but it seemed like every compiler at Google was on my interview list, which was amazing.
The opportunity brought by this interview is that I eventually joined the advertising team of this startup company, which was still in its early stages, just as mobile devices were about to take off. I helped build the mobile advertising infrastructure for the company, then returned to Google headquarters, where I helped LinkedIn go public, managing its engineering operations team, and then we were acquired by Microsoft.
Host: You are in the right place, at the right time, and trying your best, doing the most interesting things on things that are truly growing. Now we turn our focus to AI machine learning.
Obviously, you have done a lot of work at Microsoft and been involved in collaborations with OpenAI and other companies, how do you view the AI practice team?
Kevin Scott: I think if you are going to build a very complex AI platform, such as large distributed systems for training and inference, requiring components like networks, silicon, and system software, I think a Ph.D. is very helpful. You need a lot of foundational knowledge to jump into the problem and be able to move forward quickly, or, you definitely need a Ph.D., but you need to be smart enough, generally people with Ph.D.s are very smart So, I think this is a major contributing factor because you have gone through a rather rigorous training program where you cram a bunch of previous techniques into your brain, can handle a very complex project, and you have a doctoral degree.
Host: It sounds a bit like an AI platform system project. However, when you earn a doctoral degree, you usually work relatively independently on your specific thing. So, one thing people have to learn is how to integrate themselves into a team and be able to collaborate effectively with some other people. Your advice is very helpful. But besides building the platform, AI has a lot of other things to do. Such as figuring out how to apply it to education, how to apply it to healthcare, how to develop developer tools around it, and so on,
Speaking of which, Microsoft seems to have the most influential and ambitious AI strategy. Can you briefly describe what Microsoft's AI strategy is? If you were to rate yourself, what is the best work you have done in it? What work may not be as good?
Kevin Scott: We have actually been talking about this topic. I think Microsoft is a platform company, and we have been involved in or helped drive some large platform computing waves. We are deservedly one of the pillar companies in the PC revolution, and we played an important role in the internet revolution, although I think that was a distant role.
What we are considering is, how to build a technical platform for this particular technological era that allows others to build on that platform, make useful things for others - that's our AI strategy. From cutting-edge models to small language models, to highly optimized inference infrastructure, we are constantly expanding the scale of training and inference, making the entire platform more accessible, making each generation of models cheaper and more powerful. Like all other developer tools, ensuring secure infrastructure and testing and everything necessary to build robust AI applications so you can fill in the technical gaps. That's our strategy, and I think we're doing well.
I am an engineer, and I think most engineers are short-term pessimists and long-term optimists. For example, "I don't like these, I have a lot of things to solve, I'm frustrated, but I still have to deal with all these things and believe they will eventually be resolved." So, there are many things I think we are doing very well. One thing is absolute: together with OpenAI, we are making very powerful AI more accessible to more people. Through our work with OpenAI, we have found many new customers who would not otherwise build powerful AI applications. So, I think we are doing well in collaborating with OpenAI. We currently hold a concept, although it may change in the future, about what an AI platform should look like, and we are working to make it as complete as possible.
I think we actually fell in love with some basic AI a bit late. So it's not that we didn't invest in AI. You can look at some of the work done by Microsoft Research over the years (MSR can be said to be an early leader in AI).
In fact, **perhaps the most important advances in the field of artificial intelligence over the past 20 years have been related to some kind of scale - usually a combination of data scale and computing scale - that allows you to do things that are impossible at lower scales. At some point, data and computing power are at an exponential scale, so you can make scattered bets. From an economic perspective, it's impossible to bet on 10 different things, all of which are expanding at an exponential rate, or have a trend of expanding at an exponential rate at the same time.
So I think there's one thing we're a bit late in doing, and that is we didn't quickly put all our eggs in the right basket. We've spent a lot of money on AI, but it's spread across a bunch of different things. Because we don't want to hurt the feelings of smart people. Anyway, I don't even know what the endgame of these projects is, because a lot of them were done before I came to Microsoft, and our actions just haven't been as fast as we said, but now we've shifted our investment focus to scaling up.
Host: When did you start to become a believer in "scale first"? Was there a specific time or event?
Kevin Scott: I had been working at Microsoft for about seven and a half years, and if when I became CTO, my job was to scan Microsoft and the entire industry from left to right, trying to see where we were just lacking in execution, about two or three years later, Microsoft's biggest problem would be the lack of progress in AI. So I would say, around mid-2017, I had faith in scaling up, which was an important part of my work and helped us figure out what the strategy was.
Shortly thereafter, I reorganized a bunch of things internally at Microsoft to make us more focused on AI. About a year later, we made our first deal with OpenAI. Yes, we've been accelerating investments, trying to be more focused, clearer, and more purposeful.
Host: What potential of OpenAI did you see earliest?
Kevin Scott: We believe, or at least I believe, that as these models expand, they will become the basis for building a platform.
You have a data pool, a bunch of machines, and an algorithm, and you can train a model, but this model is like training something specific. Like another thing I did at Google, it's like predicting ad click-through rates, precise and effective, right? But before GPT, most of the work was about those narrow use cases. It's like building models for narrow things, and it's hard to scale before GPT
If you want to copy all of this, you must have a Ph.D. in a different data application direction and a Ph.D. in the AI direction. And, as long as you want to build artificial intelligence in applications, you need different processes. At that time, these large language models of OpenAI were applicable to many different things, so you don't need to build separate models for machine translation and sentiment analysis. I thought at the time, well, this is really unusual.
Therefore, with the expansion of scale, transfer learning works better. We know that large language models can do addition and subtraction, so when you reach the next scale point, their capabilities become slightly or significantly more general. And, we and OpenAI share the same belief. They have conducted very principled analysis of how the platform features evolve over time as a function of scale and have conducted a lot of experimental verification, proving their conjecture to be correct.
So, finding a partner who shares the same platform belief as you and is able to train and verify through these scale points is not like many things I have done in the past. I have more reservations about past investments, but I have a high belief in this collaboration, even though many people disagree with this view.
Host: You mentioned investment, and now many industry media are speculating on the cost of training models. There are rumors that it will cost tens of billions, hundreds of billions of dollars, and so on. I think, based on my own background, I believe that training will soon be replaced by inference, otherwise, no one will know how to use the models we build, right? Such investments may not be cost-effective.
So, how do you view the development of the computing field? Where is it heading? I think people will joke that all the money is flowing to NVIDIA now.
Kevin Scott: NVIDIA is doing very well. In terms of the efficiency of scale expansion, an interesting thing happening now is that the price performance of each generation of hardware is higher, usually exceeding the application of Moore's Law in general computing. You know, the cost performance of A100 is three and a half times better than V100, and H100, although not as good, is very close. From the current descriptions, the next generation looks very good as well. So for various reasons, the hardware you have can be reused in parts such as process technology and architectural composition.
So, you don't need 64-bit arithmetic operations, but you need arithmetic operations with lower precision. As a result, parallelism has become embarrassingly large. The ability to extract parallelism in hardware architecture is getting stronger, and there are many innovations in networking as well. Just like we have passed the stage of frontier models, at least you can do anything interesting on a single GPU. Training and inference have been like this for years.
In fact, since 2012, we have not had effective power scaling, more and more transistors, but the temperature is getting lower. We have a lot of density issues, it's just the power dissipation problem we have to deal with
Host: Does this kind of inference drive different data center architectures?
Kevin Scott: We have built our training environment and inference environment in different ways. From silicon to network architecture, you need different things for inference, which is easier than training. We are now building an environment through inference that would take years to build.
If someone comes up with better silicon architecture, better network architecture, better cooling technology, it's like an experiment that is easier to run, you just need to swap out some racks. I mean, it's easier than building a large capital project like a training environment. So, intuitively, you would think this would lead to a more diverse inference environment, more intense competition, and faster iteration speed.
In terms of software, we see the same situation with the inference stack, as it takes up a large part of the computational space and is currently in short supply, it is constrained. So, there is a strong incentive to optimize the software stack to extract more performance.
Host: Do you think we will soon be in an environment where the demand-supply balance changes? Not necessarily at Microsoft, but it feels like we are seeing this at the market level.
Kevin Scott: Building cutting-edge models is a very resource-intensive thing. As long as people like to build cutting-edge models and make them accessible, like they may not be in a way that people want them to be acceptable and accessible, you know, like only accessible through APIs, like something not open-source, you can instantiate, you know, mess around everywhere, but. You've seen the trend.
Building cutting-edge models is a very resource-intensive thing, as long as people like to build cutting-edge models and are committed to making them accessible, a lot of money has to be spent on this. If you are going to start a company now, the premise is that you must build your own cutting-edge models.
For example, it's like I have to build my own smartphone hardware and operating system in order to deliver this mobile application.
I think, for the market, what makes sense is that you would want to see a lot of people doing a lot of model inference, because that means a lot of products have found product-market fit, which means these things are expanding, but like a lot of speculative capital flowing into infrastructure development.
In terms of expansion, Microsoft recently published a paper, stating that the quality of training data is at least as important as quantity. I think what you are seeing in the industry now is speculation that we are running out of sources of high-quality training data. You have probably read some articles claiming to be building various partnerships to acquire training data, and these data may all be behind paywalls, etc. How do you think this situation will develop? Because we feel that computing power is getting stronger, but training data may be getting scarcer I think this is almost inevitable. In my opinion, the quality of data is more important than the quantity, which is a good thing because it can provide templates for training AI algorithms and provide an economic framework for future collaborations.
You know this will lead to more intelligent models. To be honest, this will not waste a lot of computing resources on trivial matters. From an infrastructure perspective, one thing that people have always been confused about is that large language models are not databases. If you need it to be your retrieval engine, you shouldn't think of it as "hey, I have this thing, I have to put everything in it."
We believe that the way things will develop is that you have data that is valuable for training models, and then you need access to the data or applications to infer the model. These are two different things. I think, around these things, they may be two different business models.
All of our data is now in the search engine, not in random weights, but very clearly located in the index, waiting to be retrieved like in Google. You enter a query, and then you either send traffic, do search engine optimization and advertising, like a bunch of business models around these things.
I think, we will find a business model for recommending data, so that when agents or AI applications need to get some information from someone, they can reason and give the user an answer. We will find a business model for this. Either it's subscription, sharing, licensing, or a new advertising model. A few days ago, I was telling someone that if I were still in my twenties, for all of you entrepreneurs, we should have someone figure out what new ad units are for agents, just like a newly established company, because it will have the same characteristics and qualities as previous ad units, like people with information, products, and services, they want to get the attention of people who may need this data, products, and services. Quality is important, relevance is also important, and many other things.
Host: Speaking of which, one thing we often hear is that the value function is a bottleneck in broader reasoning capabilities in some respects. But as you enter broader domains, building a value function becomes more difficult. Are there practical solutions to this problem? Does it have practical implications? More broadly, where do you think the overall field of reasoning and elements is heading?
Kevin Scott: We are just trying to draw conclusions through a series of benchmark examples. One of the interesting things we have seen in the past few years is that we are saturating these benchmarks very quickly. In the first generation of models, you will completely or very close to saturating a specific benchmark, and then you have to find something else to help guide you. So, the problem you mentioned is actually a series of expensive experiments that run expensively at the finest granularity you can imagine, just like teaching a textbook, it's like part of a story contributing symbolically to the evaluation
Host: Where do you think the current model stands? I think Microsoft has launched a large number of collaborative pilot projects, trying to help end users use your products, and so on. On the other hand, I see many companies trying to establish autonomous agents. Now, the expected performance range of these models is very wide. Where do you think we are now, and where will we be in the next few years?
Kevin Scott: I think this is a very good question. You know, there is even a philosophical view that everyone's work will be replaced by AI. The reason we give AI the title of "co-pilot" is because we hope to at least encourage everyone at Microsoft who is building these things to think: How can I help those who are engaged in some form of cognitive work to enhance their cognitive abilities.
So, what we want to build is a system, not a replacement technology. The good news is that when you narrow the scope to a field, it is also easier to think about how to transform from rough frontier model capabilities into useful tools. Therefore, I think this is a reasonable deployment path. We already have some collaborative pilot projects, and now there is real market traction, with many people using them daily.
And in fact, the more general work the co-pilot can do, the harder it is to replace you taking high-precision actions independently, especially when you know what it is doing on your behalf. Once it makes a bunch of mistakes, the user's first reaction is "this doesn't work," "I won't try it again for a long time," and such errors are everywhere. This means that you have to optimize for use cases rather than super broad things. So, we prefer it to be very good before it is launched.
Host: Everyone starts playing with OpenAI in the same way, and then maybe they start using some other proprietary base models, which combine some open-source models, maybe they have some of their own things. There is a vector database. From an architectural perspective, it feels like people tend to go on a slightly different journey. But 12 or 18 months later, what we hear from them is that there is a huge 80-20 rule at work—you can automate most tasks very quickly and effectively, but the last mile, the last few percent, is hard to make you truly trust it.
Yes, for many tasks, this seems quite elusive. So one thing I am very curious about is, when will the base model itself be good enough to eliminate the final 2%?
Kevin Scott: I think, for a while, both will coexist. I know you may ask this question, no matter how others see it, we have not seen diminishing marginal returns as we scale up, and I have been trying to get everyone to understand this. In fact, we have a measuring parameter, but it can only be sampled every few years because building supercomputers and training models on them takes time
The next model is on the way, I can't tell you when, nor can I predict exactly how good it will be, but it's almost certain to be better. It can address those issues where you might think "Oh my God, this is a bit too expensive" or "This is too fragile", all of these will get better, and become cheaper, more durable, making more complex things possible. In every generation of model iteration, such stories have been unfolding.
Even within Microsoft, we are pondering this question. One mistake our developers may make when developing these AI products is that they think the only way to solve my problem is that I must leverage the current cutting-edge technology and supplement it with a bunch of things. But you do have to do that, but be very careful in the architecture, when you do this, it won't stop you from taking the next sample when the next sample arrives.
So, what everyone is thinking about is to architect these applications well so that when new good things come, you can apply them. I think this is a part that we have been refining repeatedly.
One thing that gives us a headache internally is that some teams within the company, after seeing cutting-edge models, will say, "Oh my, we can't deploy products on this because it's too fragile and too expensive." My advice to everyone is to give yourself enough flexibility so that when new frontiers emerge, you can quickly adapt to them. This way, you can retain your skepticism and believe in the field you believe in