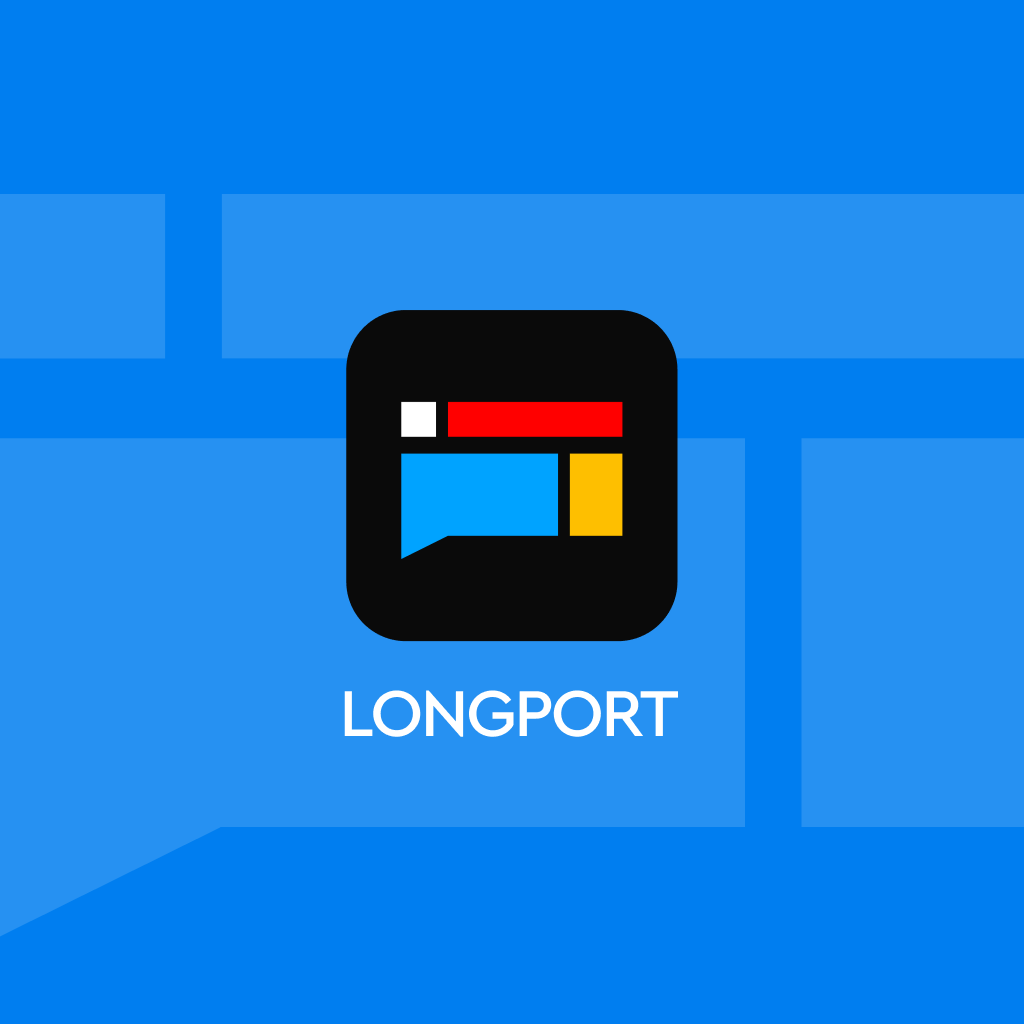
Is the era of self-driving GPT here?
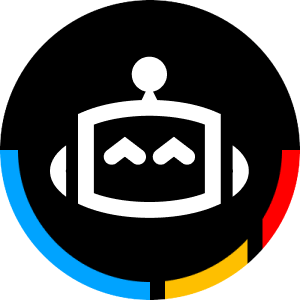
The autonomous driving industry has made significant progress recently, with Tesla launching the V12 version of FSD, and RoboSense testing on the roads in Wuhan, causing a sensation across the internet. The commercialization of autonomous driving technology is influenced by technological advancements, capital market reactions, and business models. The end-to-end learning approach in autonomous driving demonstrates advantages in providing a smoother driving experience. Tesla has unique advantages in the field of autonomous driving, including the integration of data systems and computing power. The commercialization of Robotaxi faces challenges such as technology, costs, regulations, and societal acceptance. The safety issues of autonomous driving also need to be emphasized. The autonomous driving industry is developing rapidly, but it still needs to overcome multiple challenges
Recently, many major companies have released their latest developments in the field of autonomous driving. Tesla recently launched the V12 version of FSD, which has greatly improved the driving experience. "Luobo Kuai Pao" has been testing on the roads in Wuhan and has become popular across the internet, leading to a surge in orders for autonomous driving taxis. Robotaxi has become an important scenario for the commercialization of autonomous driving. So, is the autonomous driving industry entering a rapid development stage? How far away is the commercialization of autonomous driving taxis (Robotaxi), and what challenges will it face?
On July 19th, Tencent Research Institute, in collaboration with Tencent Technology, invited AI and autonomous driving industry experts to launch the live broadcast "AGI Roadmap: Is it the GPT Moment for Autonomous Driving?".
Guests in this episode:
Xiao Yanghua, Director of Shanghai Data Science Key Laboratory/Fudan University Computer Science Professor
Wang Qi, Head of Tencent's Autonomous Driving Decision Planning Control Team
David, CEO of Whill Intelligent, host of the million-fan podcast "DaXiao Ma Talks Tech"
Zhang Junwei, Engineer/Angel Investor at a major company in Silicon Valley
Key Points Summary
Significant progress in autonomous driving technology: In recent years, significant progress has been made in autonomous driving technology, especially the maturity of large-scale neural network end-to-end learning technology, which is crucial for the development of the autonomous driving industry.
Integration of technology and commercialization: The commercialization of autonomous driving technology not only depends on technological progress but also is influenced by capital market reactions and business models. Different companies have differences in technology roadmap choices and commercialization strategies.
Importance of end-to-end learning: The end-to-end approach in autonomous driving demonstrates its advantages, being able to better handle complex interactions in traffic environments and provide a smoother driving experience.
Tesla's unique advantages: Tesla has unique advantages in the field of autonomous driving, mainly reflected in its data system and computational integration, as well as the uniformity of its vehicle platform.
Commercialization challenges of Robotaxi: The commercialization of Robotaxi not only faces technological challenges but also involves factors such as costs, regulations, and societal acceptance.
Relationship between humans and vehicles: When driving, humans are not only completing the driving task but also managing the entire vehicle.
Safety issues of autonomous driving: The safety of autonomous driving remains a key issue, especially in handling long-tail scenarios and special cases, requiring a combination of end-to-end and traditional expert system approaches to enhance safety.
Vision of the future of autonomous driving: In the future, autonomous driving may change urban traffic and parking patterns, reduce urban congestion and parking demand, and improve the efficiency of urban space utilization.
Future of human-machine coexistence: The development of autonomous driving technology may not completely replace human drivers. In the future, it may be a situation of human-machine coexistence, where autonomous driving systems can serve as an "external brain" to enhance driving safety and efficiency.
Maturity of technology and societal adaptation: The maturity of autonomous driving technology may progress faster than expected, but the adaptation of social structures and relationships is a slow process, which may affect the large-scale popularization of autonomous driving technology
Current Status of Autonomous Driving
Xu Siyan: For autonomous driving, many people are both familiar and unfamiliar with it. The trend of autonomous driving started several years earlier than this wave of large models. However, in the past few years, we have also encountered many lows. But with the re-emergence of Robotaxi in everyone's sight, we are pleasantly surprised to find that autonomous driving has made a lot of progress in the past few years. Today, we are very happy to have four industry experts to discuss the progress of autonomous driving together. David, as a witness in the field of autonomous driving, how do you see the development of the autonomous driving industry in the past few years?
David: I think the development of autonomous driving can be viewed from three perspectives: technology, the reaction of the capital market, and the commercialization process.
Firstly, technically, if we continue to use the solution of rooftop-mounted LiDAR and cameras, which is different from Tesla's vision-based approach, it directly affects the commercialization process due to cost differences. Many domestic startups, including Baidu, use similar methods, whether it's large models or small models, the underlying technology is similar.
Secondly, the capital market, autonomous driving has experienced multiple fluctuations. From the entrepreneurial boom of 2017-2018, to the cooling at the beginning of the epidemic, to the re-heating in 2021, and recently cooling down again due to the emergence of other new technologies. The reaction of the capital market is very important for autonomous driving companies, directly affecting whether the company can develop in the long term. Because the technology is still difficult to sustain through commercialization.
Thirdly, the level of commercialization of autonomous driving. Recently, RoboTaxi has been very popular, but its commercial data is inflated, only covering the operating costs of a single vehicle, still far from real profitability. Traditional companies focusing on L4 have seen some commercial prospects by shifting to L2, L2+, or L3 projects. Especially in the fiercely competitive electric vehicle market in China, technological development far exceeds North America. For example, the Korean Hyundai IONIQ 5 I am driving now is weak in autonomous driving and driver assistance, but the price is close to $60,000. In China, the experience of a car at this price is completely different.
I'll stop here.
Xu Siyan: From a technological perspective, what is the mainstream development path of autonomous driving now?
Xiao Yanghua: Looking at the development history of artificial intelligence, autonomous driving technology has been developing for many years. Recently, people feel that the landing speed of autonomous driving is accelerating, and an important reason is the maturity of large-scale neural network end-to-end learning technology. Especially after the emergence of Sora, its powerful world modeling capability is very important for the autonomous driving industry.
This is an important turning point. Most previous autonomous driving solutions were non-end-to-end, with various modules stacked together. Even if the accuracy of each module reaches 99%, the overall solution's accuracy is still unacceptable. Therefore, end-to-end environmental modeling and reconstruction are important reasons for the realization of autonomous driving technology. This is mainly due to the development of deep artificial intelligence in recent years. Therefore, when talking about the landing of artificial intelligence now, it cannot be separated from this background, which is fundamentally different from previous AI technologies Wang Qi: The biggest change in the past two years is the transition from traditional methods to end-to-end. Traditional methods are now facing some bottlenecks. Traditional methods separate various modules, such as perception, decision planning, and control, especially between perception and decision planning. Traditional methods have a clear definition, which is a more explicit definition. The output of perception is some objects, such as vehicles, pedestrians, etc., usually output in physical quantities, such as bounding boxes, directions, accelerations, etc.
During our testing process, we found that the interaction between elements, people, and vehicles in the traffic environment is not so simple. Let me give you an example: one of our test vehicles experienced a takeover event. It happened at a very small intersection. When we looked into the takeover in the background and analyzed why it happened, we couldn't understand why it took place when looking at all the physical quantities and data. Because we couldn't figure it out, we asked the safety driver. The driver said that at that time, a car from the opposite direction wanted to make a left turn. The driver from the opposite side made a gesture in advance to indicate that he wanted to turn left and hoped to pass, but there were no visible changes in the physical quantities of the vehicle. So the driver stopped to let the other car pass.
This is an example, including when passing through a pedestrian crossing during routine testing, there is communication with pedestrians on the roadside, but it cannot be reflected in the changes in physical quantities. If you want to define the postures or even the head orientations of these people through traditional methods, it is too complex, almost unimaginable.
The end-to-end approach may be a good solution to achieve more advanced autonomous driving. For example, when there is interaction, the vehicle can stop in advance, with less deceleration, providing a better driving experience. If the response is delayed until the physical quantities change, it may lead to abrupt braking.
From this perspective, I think the end-to-end approach may be a good way to enhance the driving experience in the future. Considering the complexity of the traffic environment, the interaction between people is not cold. Traditional modular solutions, such as perception, define the interface, and the cost of training human annotations for these objects is very high. It is hoped that modularization will eventually achieve single modularization, inputting an image and outputting the driver's driving trajectory or throttle and brake, eliminating the cost of annotations for all intermediate processes.
There is a gray area between traditional modular perception and PNC, or perception and control, often leading to technical communication issues. These can all be eliminated with an end-to-end approach. Thank you.
Xu Siyan: After experiencing several different autonomous driving products in Silicon Valley, what was your own experience like?
Zhang Junwei: I think the driving experience was very smooth. The road conditions in San Francisco are somewhat similar to Chongqing, with many ups and downs, so it is a challenge to operate there. I have discussed with my former colleagues, and they believe that achieving the current performance in San Francisco is already very good. Waymo has mostly reached this level before its official launch. This indicates that they were not aggressive in their promotion, and regulations may have been ahead. Secondly, Tesla's FSD (Full Self-Driving) is indeed very smooth, especially on simple roads, where you can basically let it drive without much intervention.
There are many reasons for this. Over the past decade, many people have been working on CV or now on ARP. Everyone has been improving the technology, and finally, there is a unified CVARP transformer model, combining sales attention and cross attention In addition to the computing power support from Huang, now with the synergy of end terminals and cloud, Horizon Robotics in China also has chip manufacturing experience. With shared resources from various aspects, coupled with the push from capital and academia, transformer has many advantages compared to the previous rule-based systems.
For example, in the summer, if a person suddenly activates the wipers while making a turn, self-attention can understand that it was just a mistake, whereas the code written by engineers in the past could not handle such situations.
Due to the adaptive mechanism and increased computing power of transformers, many previously hidden problems can now be identified, making the driving experience smoother and more human-like. Now we have large-scale language models (LLM) and vision language models (VLM). In autonomous driving, safety requirements are very high, and there cannot be any errors. Traditional algorithms in the past could not handle many edge cases, such as the incident where Waymo was hit by a donkey, which was hard to predict.
Multi-modal inputs, such as images and point clouds, can be processed through a single architecture, and VLM can help identify edge cases. For example, if a truck drops a BBQ grill in front of you, you need to avoid it, but if it drops a plastic bag, you can continue driving. Previously, point cloud detection could only recognize 21 objects, but now VLM can enhance the recognition of edge cases in autonomous driving technology. We can see that efforts from various aspects such as capital, academia, and engineering have finally led to a breakthrough.
Xu Siyan: After listening to everyone's analysis, we may infer that end-to-end is a more reasonable direction for technological evolution. So, besides Tesla, will other manufacturers consider using an end-to-end solution?
David: I think it's difficult for most companies to transition to end-to-end now. Why do I say this? Because autonomous driving can be divided into two aspects. One is like Waymo that we know. In China, it's companies like Baidu's Apollo, or services provided by companies like WeRide and Pony.ai. These companies provide mobility services, not just technology. You don't care about the radar and cameras on the roof of the car, or the algorithms behind them.
Waymo's trunk contains two A100 graphics cards, each priced at nearly $20,000, so just the graphics cards alone cost almost $40,000. The entire Waymo car costs at least $150,000. It's like driving a Mercedes S600 on the road. In China, this cost is basically over 500,000 RMB. So, mobility service companies must use a large number of sensors and powerful computing power to support algorithms and vehicles to ensure enough redundancy to get you from point A to point B.
For most car companies, such as Tesla or XPeng, their goal is not to provide mobility services but to sell cars. The number of cars sold directly affects the stock price. We need to look at this as two separate worlds: car-selling companies are very sensitive to costs, every additional 100 RMB is important to the OEMs because it directly affects profit margins and whether consumers are willing to buy into this system of large-scale models, or a system mainly based on vision without expensive sensors like LiDAR and high-precision maps This system saves money because the long-term training cost of large models is smaller than that of traditional rule-based systems.
I think that ride-hailing service companies are unable to transform anymore, or have gone too far on this path to turn back. Now, to do something based on large models, it may require overturning previous work. Whether the market and capital can support starting over is a question.
For automakers, I think they still have a window of opportunity, especially for companies that have done well in data platforms. The system has been established, with many cars on the road, able to achieve data closed-loop and then algorithm closed-loop. This is feasible. If cars are not selling well, it will be difficult to implement large models because the gap is too large.
Xu Siyan: Can we infer that currently only Tesla in the world has the capability to do end-to-end models, and even in the future become a company that provides transportation services?
David: This is a very good question. To be honest, I think Tesla does indeed stand out in this aspect. They have used in-car hardware, now called HW4.0, and of course, there is also HW5.0. Compared to companies using Nvidia, from a data perspective, the absolute computing power of HW4.0 is much lower than Nvidia's, even similar to Horizon's J5. Why does it perform so well? Because it has a huge system, its data system and computing power are all its own. Whether DO comes out or not, all these GPUs are its assets, in addition to millions of cars on the road continuously providing data. In fact, the most correct thing Tesla has done is not to launch too many SKUs. Unlike Nio, XPeng, or other domestic new energy vehicle manufacturers aggressively launching new cars.
In the end, for Tesla, the Model 3 and Model Y are on the same platform, you can even understand that the Model Y is just the Model 3, just increased in height, with the Z-axis of the front camera slightly higher. Most BEV data can be shared on this platform. From the beginning of entrepreneurship, for each model Tesla has on the road, there are millions of car owners continuously contributing data to it. At this level, Tesla has no competitors in the world. Although Toyota and Honda sell more cars, most of those cars are non-intelligent. Companies in China that may sell well like Li Auto, one issue is that they have quite a few SKUs, such as L9 and L6, or even Mega are not on the same data platform. Although these cars sell over ten thousand units per month, which is considered good sales in China, they are still too small compared to the "miracle car" Model Y. So, if we answer Siyan's question at this point, perhaps only Tesla has the unique technology and foresight.
The Commercialization Path of Robotaxi
Xu Siyan: In the first part just now, everyone has reviewed the entire development process of autonomous driving, and I think the guests all have a fairly consistent judgment that the end-to-end architecture is very advanced in autonomous driving and is a future development direction. In the second part, we want to discuss the current development status and commercialization path of robotaxis David: The earliest thing we did was unmanned delivery, called the last mile delivery. Delivering takeout or products from warehouses or restaurants to doorsteps, rather than doing robot taxis. We found that robot taxis are not a good business, but I am not against peers doing this. I still have great respect for those companies, just sharing my feelings.
In the United States, the situation is that there is insufficient capacity and high unit prices. In the United States, it's about a little over a dollar per mile, and the difference in China lies in the exchange rate. The taxi industry in the United States may be more profitable. In Japan, due to aging, taxi drivers are mostly elderly, and unmanned taxi services will be an incremental market.
There is also a misconception that equates L4 with unmanned taxis. L4 is a classification of vehicle automation, meeting certain conditions is L4. Tesla's FSD is a bit misleading in its promotion, although it performs well at L2, it cannot fully meet the requirements of L4. So Tesla users often have surprises, but this does not mean the technology is very mature.
Finally, L4 does not equal unmanned taxi services. Unmanned travel services involve multiple dimensions inside and outside the vehicle. The comfort and service quality inside the vehicle are also important. For example, if you set a location on the mobile app, but the car cannot reach it accurately, it will affect the user experience. So I think L4 technology still has a long way to go.
Eddie Wu: What level of automation does our unmanned taxi service really need? How should future safety officers and autonomous driving systems cooperate?
David: Behind the unmanned taxi of "Luobo Kuai Pao" is a real-time control system. When encountering problems, such as an elderly person falling in front of you, you can use this steering wheel to control the car. The car will respond to your operation in real-time. This control module is connected to the car's CAN bus, connected to the server via 5G or 4G, and then to your steering wheel Just like live streaming, it's real-time. However, this is very dangerous. Once the signal is interrupted, there will be problems with controlling the vehicle.
American companies have two technological routes. One is semi-autonomous driving for vehicles. It involves re-planning the route on a high-precision map. If you are not familiar with this technology, I can explain it to you. The vehicle has a planned line on a high-precision map, with each point marked based on high-definition maps and semantic maps, and the vehicle moves along these points.
One technological route is like Zoox in the United States, where they help you reconnect these points. For example, if there is a traffic cone or an elderly person blocking the way ahead, the remote controller does not control the steering wheel in real-time but reconnects the points. The vehicle does not switch from autonomous driving mode to manual driving mode but continues in autonomous driving mode, starting from the newly connected points. The other method is used by our customers, where the vehicle has autonomous driving capabilities. You confirm a command, such as turning left or right, and after turning, the vehicle continues in autonomous driving mode. Neither of these methods involves real-time control like a carrot running fast. In my opinion, controlling a vehicle in real-time is very dangerous.
Wang Qi: I tend to agree with David's point, that is, if Robotaxi and L4 technologies themselves have certain differences in their implementation. One issue we are discussing now is what a person really means to a vehicle. In my opinion, when a person is driving, it is not just about completing the driving task, but he is actually the manager of the entire vehicle. Just like a train, it has a train conductor. The conductor's role is not just driving, but also paying attention to the vehicle's movement and managing many other tasks. He has to manage various situations on the vehicle.
For example, if you pick up a passenger today, and the passenger starts sleeping after drinking at night. When getting off, if there is no one around, can you wake him up just by voice? You might have to push him a bit. If after pushing him, he still doesn't wake up and his breathing is abnormal, then you may have to take him to the hospital. As a vehicle manager, we see some scenarios where there is a traffic jam at an intersection, and a traffic policeman comes to direct traffic. If there is no one, you cannot interact effectively with the traffic policeman. In some cases, both sides of the road are jammed, and only one lane can pass through, and there are oncoming vehicles. In this situation, if there is someone, you might roll down the car window, talk to the other party, or even get out of the car to negotiate with the other party, come up with a plan to make way for each other to pass through.
A person is the manager of the vehicle, and driving is just a part of all his work, although it is the most important task, there are many other tasks besides that. Like the example of drinking mentioned earlier, the probability of it happening is very low, maybe you will encounter it once every one or two months, but if it happens, you must solve it, you cannot ignore it. These are issues that current technology development has not yet solved well. My view on Robotaxi is that if you really want to replace the existing taxi business, these issues must have a reasonable solution.
Xu Siyan: Will we see driverless taxis without steering wheels in the future? Wang Qi: We have an office park under construction in Shenzhen. Tencent Headquarters Park is a peninsula mainly used for office and commercial purposes. Our goal is to provide shuttle services within the park to facilitate employee commuting and tourist sightseeing. Penguin Island may become a tourist attraction in the future, so there will be visitors on weekends. We plan to launch autonomous sightseeing vehicles and apply some existing technologies on these vehicles.
During the research and development process, we encountered an interesting scenario. Our vehicle is independently designed and different from regular cars. It has no front or back, can be driven forwards or backwards, and does not have a steering wheel. To achieve end-to-end, human-machine co-driving is required initially. The prerequisite for human-machine co-driving is the need for a steering wheel and driver input. The current issue is that I believe in the future, autonomous driving will evolve to a stage similar to the problem we are facing. Your autonomous driving is already very good and does not need to learn from humans anymore. At that point, you will remove the steering wheel. The iteration of technology in this scenario becomes distant. Currently, we are mainly addressing the end-to-end issue. In the future, without driver input, does it stop iterating after removing the steering wheel? It will definitely continue to iterate, and it will do better than humans.
There is another issue. When evaluating end-to-end, people often use a phrase: "his driving behavior is more like a human." But does being like a human mean better? Of course, human driving is often reasonable, but humans have blind spots when focusing inside the car. Driving instructors often say during lessons: "Give way, not speed." Why? Because humans can only focus on one direction at a time. For example, if there is a sudden accident in front of you and you need to brake suddenly, you cannot turn the steering wheel to another lane because when you focus on the front, you cannot focus on the left or right rear. This is the limitation of human attention.
Does this situation apply to autonomous driving? It does not. Why? Because autonomous driving is 360 degrees, equipped with many sensors that can see everything. I believe end-to-end can learn from excellent human driving behavior, but the limitations of human driving may also be reflected in the end-to-end model. As Professor Xiao just mentioned, in the future, we may need to combine these two methods to achieve the best driving experience!
Xu Siyan: For this autonomous driving, end-to-end can currently make it drive more like a human faster, but this is not the ultimate solution. Musk himself has said that he hopes his Robotaxi will have no steering wheel or rearview mirror. Its design should be completely different from current cars. How far away is this future from us?
Zhang Junwei: Mr. Ma previously mentioned August 8th, but now it's been postponed to October. He doesn't know the reason, maybe the technology is not mature, or he wants to delay it himself. He said he wants to build his own prototype based on the robot taxi. Mr. Ma always comes up with big plans, although with limited delivery, he is already stronger than many others. When he mentioned 100 before, we can give him a discount.
Although Dr. Wang Qi just gave many examples of drunk driving, we have to admit that technology is indeed helpful in normal driving situations. For example, when engineers write code, people worry that large models will lead to unemployment and reduce entry-level jobs. For example, in Southeast Asia, our company used to have 100 customer service writers, but now we can reduce it to 50 Driving is the same way. After technological progress, people can use their time and energy elsewhere. This is a fact, whether we like it or not. We need to keep progressing, not just discussing issues. Next year, we must make progress in skills compared to this year. Since entering the agricultural society, humans have been progressing, which is inevitable.
Xu Siyan: I agree with Junwei's view, as various aspects are driving the development of this matter. In addition to car manufacturers like Tesla, there are also new operating companies that will strive in this direction. It's not just China and the U.S., other countries will also participate. When more and more people feel that the cost of Robotaxi is lower than traditional taxis, they will try Robotaxi services under normal circumstances. It doesn't necessarily have to replace all taxi services now, but it may start by replacing 10% to 20%.
Social Impact of Robotaxi
Xu Siyan: But the third part we are going to discuss next is the social impact and challenges faced by Robotaxi. Besides technology, there are many other issues, such as policies and laws. In the U.S., trucks have always been seen as a good application scenario because long-haul truck drivers are prone to fatigue and have high accident rates. However, so far, we have not seen large-scale application of autonomous driving among truck driver groups. Is there an influence from unions or other factors?
David: Any AI technology, not just autonomous driving, may affect labor. Is it a supplement or a replacement? First of all, the U.S. is a capitalist society, and strong union power does not mean irreplaceability. For example, the average salary of American truck drivers is about $160,000. This is because they spend a lot of time on the road, away from their families, and cannot work more than a certain distance in a day. For companies like Walmart and Coca-Cola that rely on trucking capacity, if new technology is cheaper, they will definitely adopt it.
Also, I want to emphasize why there is no conflict in the U.S. Baidu's RoboTaxi used high subsidies during the demonstration. On one hand, it attracted ordinary consumers, on the other hand, it squeezed the market. Chinese companies have different business logic from American companies. For example, in San Francisco, Waymo and Uber have the same prices. Waymo, backed by Google, did not use low-price subsidies because it does not fit the business logic. American companies are unwilling to do this because it would raise the pressure threshold and harm the market in the long run.
This is a business logic issue. In the past, with the development of human society, jobs like telephone operators and typists disappeared, but these people did not starve, they went on to do other jobs. Every industrial revolution is an incremental market, not a zero-sum game.
Xiao Yanghua: I believe that society as a whole needs to consider how to place the labor force replaced by new technologies. We should seize the development opportunities of this wave of artificial intelligence, but we should not follow the old path of the Internet. Back when the Internet was developing, such as e-commerce, regardless of whether offline stores needed it, we directly replaced many offline jobs, seemingly improving efficiency, but also bringing many problems. For example, many students now like to order takeout, their physical fitness has declined, the time spent eating takeout has not been used to exercise, but to do other things. Although efficiency has improved, it has also brought social problems I believe that the application of large-scale models or artificial intelligence to C should consider social costs and governance costs. However, in industries such as manufacturing, aerospace, and aviation, AI can be massively promoted to enhance productivity. In applications targeting ordinary consumers, especially in fields involving a large number of jobs, we need to be cautious.
Xu Siyan: Finally, I would like everyone to imagine the future. In five to ten years, if the safety of autonomous driving technology surpasses the benchmark, it may be widely popularized, even changing the definition of cars. Musk envisioned that his Robotaxi could bring additional income to car owners during idle times, becoming a productivity tool. Is such a future possible?
Wang Qi: It's difficult to predict the timing of realization. Although we have made great progress, there are still some practical issues. But looking at a span of ten years, it is still possible.
I saw a foreign expert sharing a vision of future autonomous driving on a video website. He mentioned many things, but one point attracted me. If all vehicles in the city are autonomous, whether taxis or private cars, there would be no need to park around offices or homes. This means there would be no need for parking lots in the city. You can imagine that many places in the city are occupied by parking lots. Especially in narrow areas like some neighborhoods in Beijing, the streets are filled with cars. If autonomous driving is implemented, cars can park in suburban parking lots, come to pick you up in the morning, drop you off at the office, and then leave without parking around the office. This would free up a lot of space in the city, which could be turned into parks or activity areas, expanding the space for activities. This is something I find very appealing, as it would bring significant changes to the urban environment.
Another issue is Musk's shared rental cars. My understanding is that when the car is idle, it can run rentals for you. But I find it hard to imagine how this would work, because a private car is a personal space, like a phone or a computer. Can you imagine renting out your idle phone or computer to someone else? Most people cannot. Similarly, for many people, a car is like a second home, especially with children, where the trunk may have diapers or tents. How would these things be resolved if the car is rented out during idle times?
Xu Siyan: Perhaps it will evolve like when Didi first appeared, where people talked about the concept of the sharing economy, that you could drive for Didi when you have free time. But later you will find that most are still professional drivers. Self-driving taxis may evolve in a similar way, starting with the concept of sharing, and eventually becoming something people invest in as a financial product, maybe not buying one, but buying ten. If this thing is profitable, this is my guess.
David: I think within a certain range, we can achieve purely commercial operations. For example, in downtown San Francisco, or in the Nanshan and Futian districts of Shenzhen, these high-value-added areas, it is technically feasible, although it may sacrifice some efficiency. For example, a robot taxi may take 20 minutes to complete a journey that originally took 15 minutes, but the price will be cheaper. I am not sure which specific year commercialization can be achieved. Previously, we said 2018 or 2020 was the year of autonomous driving, but now it's 2024, so the specific year is not meaningful However, we will see that L4-level technology will significantly improve the safety of passenger cars and commercial vehicles. Many cars now have advanced driver assistance systems, allowing you to do more things in the car, such as chatting or watching short videos, without having to constantly focus on the road. This is also an enhancement of the driving experience. At the same time, L4-level technology will also greatly enhance safety, as advanced driver assistance systems can digitize and visualize the driving behavior data of each car owner, which may even change the insurance industry.
Previously, the auto insurance industry estimated insurance rates based on statistical data, such as the accident rate and driver profile of Toyota Camry. However, Tesla has started customizing insurance based on the specific behaviors of car owners, which is a more precise method. Advanced driver assistance can improve vehicle safety and customize insurance based on the specific behaviors of individual car owners. These are changes that will occur in the future, but the widespread adoption of Robotaxi will not happen all at once.
Xu Siyan: Thank you, I think David's summary is very good. We don't need to stand on the two opposite sides of this matter, it's not that Robotaxi will definitely replace drivers once it matures. The future we may first face is likely to be a coexistence of humans and machines. Previously at WAIC, we introduced a concept called "machine external brain", the autonomous driving system is also the external brain when we drive, which can make our driving process safer.
Xu Siyan: Finally, let's ask Teacher Xiao to give us a simple summary of this online roundtable!
Xiao Yanghua: Autonomous driving is an advanced technology and productivity that will eventually be realized. From a technological perspective, I believe the speed of technological maturity may be faster than expected. Technological progress in recent years far exceeds demand. The key is who can integrate existing technologies into mature products, and use large-scale data to find effective implementation models. I believe technology will mature quickly. I don't think it will take ten years.
What really hinders the large-scale use of autonomous driving is more social factors, such as the insurance industry. After the promotion of autonomous driving, both the insurance industry and the transportation industry will undergo changes. These changes are social issues that need to be adjusted and transitioned slowly and orderly, otherwise it may cause social upheaval. The future issues are more about whether or not, rather than whether or not. Social structures and relationships need to gradually adapt to this advanced productivity, which may be an important issue in the future. This consideration may make the widespread adoption of autonomous driving a phenomenon that takes a decade.
Author: Tencent Research Institute, Source: Tencent Research Institute, Original Title: "The GPT Moment of Autonomous Driving, Has It Arrived?"