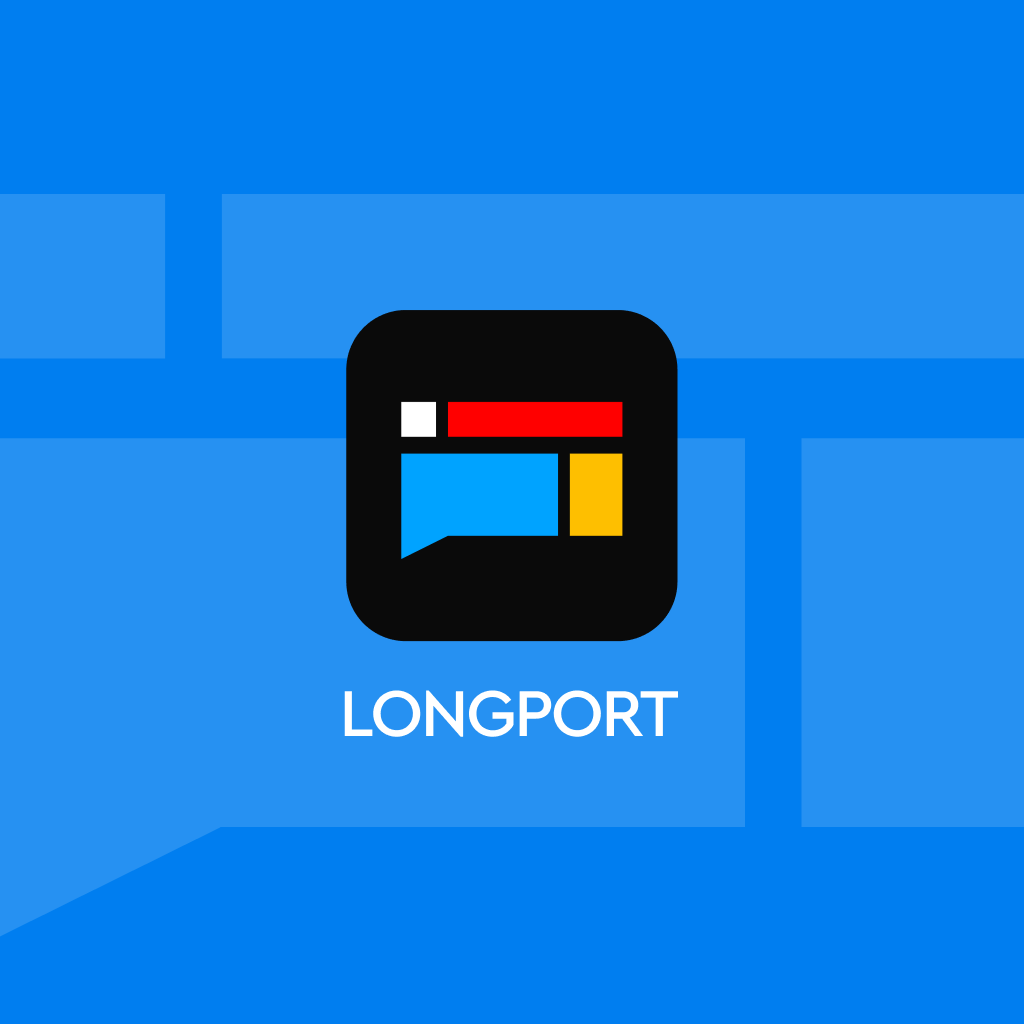
Li Auto wants to arm wrestle with Tesla
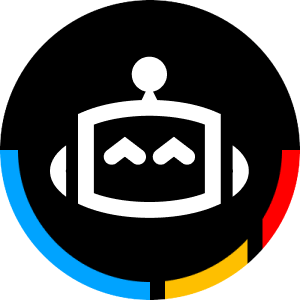
Battle of Smart Driving
Author | Chai Xuchen
Editor | Zhou Zhiyu
In the external impression, Ideal's autonomous driving has always been in a chasing state, but after going ALL IN end-to-end, Ideal surprisingly confidently claims to have surpassed Tesla.
At the Chengdu Auto Show on August 30th, Ideal Auto's autonomous driving team detailed the "end-to-end + VLM" solution. Different from the segmented end-to-end solutions of domestic peers, Ideal's solution is called "OneModel," which is like a large net.
This is the ultimate form of the current autonomous driving architecture evolution. At this stage, there is no longer a clear division of perception, decision-making, planning modules, from the original signal input to the final planned trajectory output, using a deep learning model, fully and seamlessly applied to autonomous driving.
In the view of Lang Xianpeng, Vice President of Ideal's autonomous driving R&D, stubbornly pursuing the "final version" of end-to-end is the secret to Ideal's overtaking on the curve.
"In the past autonomous driving solutions, whether it's light mapping or no mapping, the underlying technical architecture has a human-designed component. If you want to run through various scenarios throughout the year, it is impossible to achieve without one or two years. So we iterated on the end-to-end + VLM technology architecture," Lang Xianpeng believes that this architecture is grown by AI itself, "truly becoming the car driving itself."
Not only that, Ideal is starting to build a "world model" to accelerate the training of autonomous driving AI. "The world model can generate, simulate scenes, which is tens of millions of scene tests," Ideal's senior algorithm expert Zhan Kun said. This is the most important and necessary guarantee for achieving rapid iteration of autonomous driving, and the "world model" will also crush the existence of end-to-end in the future.
"It can predict the future based on the current environment, can infer future scenarios. For example, if a ball rolls to the middle of the road, end-to-end will only brake, but will the world model think a child will rush out from behind? It has a more macro comprehensive judgment of the world." Zhan Kun said that while Ideal is already on board with end-to-end, it has already pre-researched next-generation technologies.
Therefore, Lang Xianpeng boldly stated, "We are not much different from Tesla, even a bit ahead."
Daring to compete with Tesla's FSD, the global benchmark for autonomous driving, is not only due to Ideal's advanced dual-system architecture, but also due to Ideal's leading sales and financial strength among new forces. Lang Xianpeng said that achieving true end-to-end depends on two abilities, "whether there is enough data and sufficient computing power, because it is AI training."
He said that in order to train their own autonomous driving system well, Ideal has extremely high requirements for data quality, only selecting 3% of "experienced driver" data to feed AI, but with a base of 800,000 car owners, the data volume is already large enough; and to process this data, Ideal plans to increase its computing power to 800 million EFLOPS by the end of this year, "this is a spending of 2 billion RMB per year."
In Lang Xianpeng's view, advanced autonomous driving is a game that only giants can afford to play, "in the future to the L4 stage, the growth of data and computing power is exponential, requiring at least $1 billion annually, if a company's profits and earnings cannot support the investment, it will be very difficult." Relying on the end-to-end initial boarding, Li Auto has already achieved a rapid conversion of sales volume. Next, it still needs to continue to exert efforts on this "top project", which may be a key step in leading it to be on par with BYD and Tesla.
The following is a transcript of the conversation between Wall Street News and Li Auto's Vice President of R&D Lang Xianpeng and Senior Algorithm Expert Zhan Kun (edited):
Question: What is true end-to-end? How do you evaluate if it is truly end-to-end? What kind of effect is the best?
Zhan Kun: End-to-end is a research and development paradigm, from the initial input end to the final output end, without any other processes in between, implemented with a single model. Currently, Li Auto is an integrated OneModel end-to-end, directly inputting sensor data, and after the model completes the inference, it is directly used for trajectory planning to control the vehicle, which is end-to-end integration.
There is another type of end-to-end on the market, where the model is divided into two in the middle, with a signal bridging the models. However, we believe this is not truly end-to-end. If there is a human information digestion process in the middle, the efficiency may not be as high or the capability may be constrained.
Lang Xianpeng: Many people now claim to have end-to-end models, but truly achieving end-to-end depends on two capabilities: having enough data and sufficient computing power. Otherwise, I think it is difficult to truly achieve end-to-end because it involves AI training.
Question: Many brands claim to be leaders now, and Li Auto also says it has entered the first echelon of intelligent driving. How do you evaluate the technical level of your own end-to-end technology?
Lang Xianpeng: Ordinary consumers do not care about the technology but the experience, and we do not compare ourselves with anyone.
Previously, we considered using high-precision maps for urban NOA, but later decided to switch to mapless due to experiential factors. However, at that time, the mapless solution still involved perception, planning, and modularization, with a lot of manual rules and real-world testing.
Not to mention the budget investment, time was very challenging. If we wanted to run through all kinds of scenarios throughout the year, it would be impossible without one or two years. So we iterated to end-to-end + VLM technology architecture, which is an AI solution that we developed ourselves.
Previously, assisted driving was about the system assisting people to drive, with humans as the main entity. But now, in the end-to-end + VLM stage, we believe that the car is driving itself. After training a complete model, the model itself has the ability to drive the car well. I supervise the car, intervene where necessary, but the main entity is always the car, with humans as a supervisory assistant role.
Question: How long is the research and development cycle for end-to-end?
Lang Xianpeng: Li Auto started seriously working on end-to-end + VLM from last year. During the research and development phase, we had a very small and refined team. When we were working on mapless, we were already doing preliminary research on end-to-end, and now that we are actually implementing end-to-end, we are already researching the next generation of technology. When the conditions are mature and the initial verification is successful, we will move to the mass production stage.
Question: End-to-end was first proposed by Tesla. Are we inspired by Tesla? How do we ensure that the process will definitely work? Jan Kun: End-to-end was not first proposed by Tesla. In 2016, NVIDIA had a model that mentioned this technology, but the effect was only average and only solved very simple scenarios given the computing power and model scale at the time. Everyone thought this approach was not feasible. By 2023, Tesla added supercomputing power on the new transformer architecture and made progress in a more promising direction.
Question: How big is the gap between Li Auto and Tesla's autonomous driving technology currently?
Lang Xianpeng: Last year, the gap was about half a year, and this year it may be even smaller. In terms of technical architecture, we are not much different from Tesla, and even slightly more advanced because we have VLM while Tesla only has end-to-end. At least from the perspective of training computing power and training data in China, we are currently ahead of Tesla because Tesla still needs computing power deployment in China.
In addition, we have also adopted the world model, which can generate and simulate scenarios. This involves testing tens of millions of scenarios, which is the most important and necessary guarantee for rapid iteration of autonomous driving. This method of model iteration is much more reliable than the previous whole vehicle or road test methods, and it covers all kinds of scenarios throughout the year.
Jan Kun: The world model can predict the future based on the current environment and can infer future scenarios. For example, if a ball rolls into the middle of the road, end-to-end will only brake, but the world model will consider if a child might run out? It has a more macroscopic and comprehensive judgment of the world. In fact, VLM in our system serves this purpose, but of course, our model scale is still very small and limited in capability.
Question: Recently, someone raised the view that "500 billion cannot achieve good autonomous driving." What is Li Auto's opinion on this?
Lang Xianpeng: Regarding the 500 billion, it is necessary to determine whether it is a one-time investment or a long-term investment. Just like today, as mentioned, we invest $1 billion annually in autonomous driving research and development. If this continues for 10 years, it will exceed 500 billion.
The end-to-end + VLM technical architecture is a watershed, starting from this generation is truly using AI in a way.
Previously, we were still using traditional methods for autonomous driving. The ultimate effect of all products has "design" in it, and scenarios that are not designed may not be realized. Not only is it impossible to achieve purely data-driven results, but the manual workload is also significant.
The integrated end-to-end model, although challenging in terms of model structure and training methods, the biggest advantage is that we provide data to train the model, and the model outputs results, naturally leading to an AI training process.
From the perspective of our own end-to-end model, we only need to tell it to achieve a driving experience similar to that of an "experienced driver." By inputting all the driving data of "experienced drivers" among Li Auto owners, it will give you the results. Data selection is very strict, as out of 800,000 car owners, only 3% have genuine data of experienced drivers.
After establishing this premise, the core competition in R&D lies in whether there are more and better data and corresponding computing power to train the model. The acquisition of computing power and data depends on how much money and resources are invested. Some things cannot be bought with money, such as training data and training mileage, each car company has its own resources, and they do not share them with each other Another area that requires investment is computing power. We currently have 539 million EFLOPS of computing power, and it is expected to reach 800 million EFLOPS by the end of this year, which amounts to an annual expenditure of 2 billion RMB.
In the future, as we enter the L4 stage, both data and computing power will grow exponentially each year, meaning that at least 1 billion USD will be needed annually. After 5 years, continuous iteration will be required. At this scale, if a company's profits and earnings cannot support the investment, it will be very difficult.
Therefore, the focus now should not be on how many billions are invested in autonomous driving, but rather on whether there is sufficient computing power and data support at the core, and then consider how much money needs to be invested.
Question: In the past few years, the rapid technological changes in the smart driving industry have undergone several major iterations. Will there be more significant changes in the future?
Lang Xianpeng: The end-to-end + VLM dual system architecture simulates human thinking and cognition, because in AI, we ultimately hope to achieve human-like or humanoid capabilities. The current AI framework is very reasonable, and many companies have begun to try to catch up.
The dual-system theory can be applied not only in autonomous driving but also in the paradigm of future AI and even intelligent robots. Autonomous driving can be seen as a wheeled intelligent robot, with its work scope being the road. Therefore, I believe there is a certain long-term behavioral power, but technological development is endless. We will maintain agility in perceiving advanced technologies and track them if there are new technologies.
Question: How much incremental sales volume can be brought after mass production and delivery of end-to-end systems?
Lang Xianpeng: After the full push of NOA without graphics, our test drives doubled in the past two months. The proportion of AD Max models above 300,000 reached 70%, originally AD Pro had more, and L9 AD Max even accounted for over 90%.
Question: Does Li Auto have any plans to charge for advanced autonomous driving features? What are the good business models?
Lang Xianpeng: Standard configuration and free of charge have been the strategies that Li Auto has set since day one of entering intelligent driving. "Supervised autonomous driving" is not charged for all AD Max owners, and it can provide more vehicle training mileage for autonomous driving. Therefore, with good delivery volume and stable business operations, there are sufficient resources to invest in intelligent driving research and development.
Zhan Kun: Li Auto has very rich data, and we believe that this data can support our advantages. So we chose to challenge a larger and more difficult end-to-end integrated architecture, which has a high ceiling, but the downside is that training is more difficult. This includes exploring and mining data ratios and training methods, but we still resolutely chose the difficult but correct path