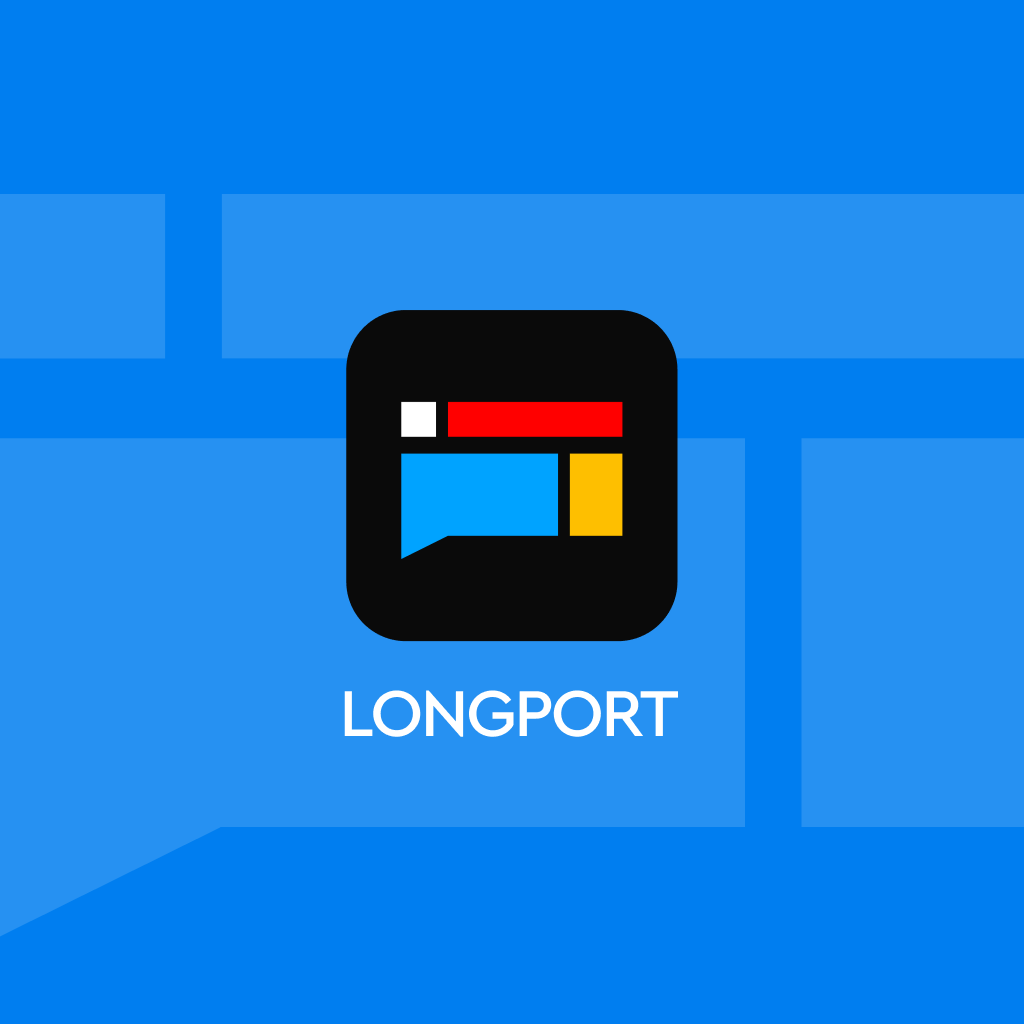
The $200 ChatGPT Pro is officially launched, introducing the new model Strawberry that is N times smarter
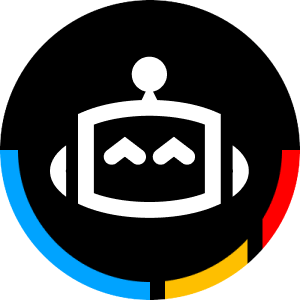
ChatGPT Pro membership is officially launched at a price of $200 per month. The membership is divided into three tiers: Plus, Team, and Pro. The Pro membership will provide support for the upcoming new model, Strawberry. Although the Pro membership currently does not have any new features, the usage of GPT-4o is almost unlimited, while Plus members are limited to 80 queries every 3 hours. The specific details of the Strawberry model have not been finalized yet, but it is expected to be based on Self-play RL technology
At 10 o'clock in the middle of the night, The Information released a piece of news, revealing OpenAI's new model, named Strawberry, is coming.
Two hours later, my good friend @solitude (Eastern Time) who always has first-hand information, told me that ChatGPT Pro membership is now available, priced at $200 per month, and he has already paid for it.
I checked my own account and indeed found nothing.
So, even before he started using it, I managed to get hold of this prestigious Pro account from him.
Currently, ChatGPT memberships are divided into 3 tiers, namely Plus, Team, Pro.
The way they are divided, it feels like OpenAI is learning from Apple, wondering if there will be a ChatGPT Pro Max in the future...
Unfortunately (for the early adopters), there are no new features at the moment, no new models either. The only difference is that the usage limit for GPT4o is basically unlimited. I tested hundreds of queries in a short period of time, and it worked smoothly.
In contrast, for ChatGPT Plus members, the usage limit for GPT4o is 80 queries every 3 hours.
Unlimited usage naturally doesn't justify the 10x price increase, from $20/month to $200/month. If OpenAI really goes down this path, it's basically like Ultraman being taken over by Musk.
Combining The Information's news, it can be basically confirmed that this ChatGPT Pro membership is being prepared for a completely new model, Strawberry.
For those who want to use Strawberry in the future, it's better to first sign up for a $200 Pro membership.
What exactly is Strawberry? There is currently no definitive conclusion, but based on the information I know, this thing, Strawberry, might be:
Based on the new paradigm of Self-play RL, extremely strong in mathematical and coding abilities, and capable of autonomously performing browser/system-level operations for users.
More intelligent, slower, and more expensive.
I will try to explain in the simplest language possible so that everyone can understand, what exactly this new Strawberry is and why it is being sold for $200/month First of all, let's talk about some issues with GPT-5.
As far as I know, the training of GPT-5 has been very difficult.
One observable point is that the "miracle of great power" based on data size and model size is starting to experience diminishing returns, and it is no longer a surefire solution.
The Scaling Law of large language models describes the relationship between model performance L, model parameter size N, training data size D, and computational complexity C.
With the increase in computational complexity, model parameters, and dataset size, the performance of the model usually significantly improves, leading to better performance in tasks such as language understanding and generation.
However, now, computational complexity, parameter size, and dataset size have all encountered bottlenecks, especially for closed-source models. The pace of progress has slowed down compared to the past, and the gap between open-source and closed-source models is gradually narrowing.
In other words, relying solely on the "miracle of great power," the model's capabilities are reaching their limits.
Fundamentally, all training of large models is the ultimate utilization of existing human knowledge. We provide data, feedback data from humans, or annotated data, and you will find that large models do not "discover" the rules of language through self-exploration, but rather extract useful information directly from the content we provide.
This is like a student who initially improves his grades by constantly memorizing books, but after a certain point, there are no more books to memorize, and the grades have reached their limit. No matter how much rote memorization is done, significant progress is difficult to achieve, which is the current dilemma.
One issue is that the magnitude of existing knowledge is no longer sufficient.
Another point is that all knowledge is memorized directly from existing sources, rather than explored from scratch, so in this process, large models learn only correlation, not causality.
Explaining correlation and causality is very simple.
Correlation: If you find that it rains every time you bring an umbrella, that's correlation. Umbrellas and rain seem related, but bringing an umbrella does not actually cause rain.
Causality: You bring an umbrella because it's raining, this is causality, because rain causes you to bring an umbrella.
So this is why when asked to do complex reasoning, the logic of the reasoning process is often messy and full of errors, it's because of this reason.
They are like an encyclopedia-style top student, knowing a lot of facts, but may not truly understand the principles behind these facts and the real cause-and-effect relationships.
If you ask a student who only memorizes things: "Why does an apple fall to the ground?" he might immediately answer, "Because of gravity."
But if you continue to ask, "What is gravity? Why does gravity exist?" he may not be able to provide a deeper explanation The current large models are not much different from this phenomenon. They can tell you that the Earth is round, but may not be able to truly explain why the Earth is round, or what impact the shape of the Earth has on our lives.
What they learn is that the words "Earth" and "round" often appear together, showing a strong correlation, rather than understanding the causal relationship of why the Earth is round.
Correlation tells you that two things always happen together, while causality tells you why they happen together.
Therefore, this is why we need new methods and paradigms to break this pattern.
And the solution to this, as I have observed, is the consensus among OpenAI, Google, Anthropic, Ilya, and others:
Self-play RL.
The full name is self-play reinforcement learning, which may sound complex, but can actually be understood with a simple analogy: a child learning to play Go.
What is the learning method of current large models? They look at game records, memorize opening setups, and memorize some fixed tactics. They learn from a large amount of data, knowing many possible solutions, but may not truly understand why they should play in a certain way.
On the other hand, Self-play RL lets this child play against themselves continuously. At the beginning, they may play poorly, but through trying different moves, observing the results of each move, slowly, they will discover which strategies are more effective and which moves will lead to losses.
In this process, the child is not just memorizing game records, but truly understanding the changes in the game, understanding why each move is made.
This is a leap from learning correlation to learning causality.
Does this description sound familiar?
This is the famous AlphaGo Zero from 2017.
Back then, AlphaGo defeated Ke Jie with a score of 3:0 in Wuzhen, causing a sensation worldwide.
And AlphaGo Zero is an advanced version of AlphaGo.
The official description of AlphaGo Zero is as follows:
"At the beginning, AlphaGo Zero was very weak and even made self-suicidal moves.
After 3 hours, AlphaGo Zero successfully entered the world of Go.
After 36 hours, AlphaGo Zero had explored all basic and important Go knowledge, with a record of 100:0, crushing the AlphaGo v18 version that defeated Lee Sedol.
21 days later, AlphaGo Zero reached the level of a Master, which is the version that achieved 60 consecutive wins online at the beginning of the year, and Master later defeated Ke Jie After 40 days, AlphaGo Zero's win rate against Master reached 90%, which means AlphaGo Zero has become the strongest Go AI that is unbeatable.
This is the terrifying power of Self-play Reinforcement Learning.
Self-play RL allows AI to continuously "play against itself", whether it's playing Go, solving math problems, or engaging in conversations.
In this process, AI is not just repeating what it has seen before, but actively exploring, trying, and learning.
In contrast to the learning method of large models, where large models excel in "rote memorization", Self-play RL excels in "self-improvement".
The data remains the same, but one is provided by humans while the other is self-generated.
By memorizing what is provided by humans, you can never become a super AI that surpasses humans. However, by creating and learning from oneself, there is a great possibility.
The fields of Go and Dota2 have already proven this point.
With large models + Self-play RL, the large model continuously plays against itself, receives feedback, optimizes model weights, adjusts its own level, and continues to battle.
Thanks to the capabilities of the large model itself, during the self-play process, it no longer needs to provide only final result feedback. This reward feedback actually has significant limitations in improving AI reasoning abilities.
Because unlike specific tasks like Go and Dota2, the capabilities of large models are too generalized. We need more causal relationships, not just results.
For large models, we can use a "thinking chain" to record every step of the AI's reasoning process. Then, each step can be scored so that the AI knows the quality of each reasoning step. This method allows the AI to not only learn how to give the correct answers but also improve the entire reasoning process, thereby understanding true causality.
Moreover, not only scoring, but also due to the capabilities of large models, textual evaluations can be provided. This is similar to when you are doing homework, where the teacher not only gives you a score but also writes comments to tell you what was done well and where improvements are needed. Surely, knowing more than just a score result makes you more powerful, right?
And each learning experience gains valuable feedback from the reasoning process.
When the model answers a complex question, it goes through a process similar to Self-play. The model generates multiple possible approaches, evaluates the quality of these approaches, and selects the best one.
In an article about overseas unicorns, a calculation was once made. For a large model with 100 billion parameters, if it generates ideas using Self-play, producing 32 ideas each time, with 5 steps in each idea, the total task consumption for one reasoning answer is 100K tokens, nearly 6 USD. Expensive and slow, but truly intelligent.
The best data will be saved and the model will be iterated at fixed intervals for continuous evolution.
That's why in the exposure of Strawberry, it is said:
"The biggest difference between Strawberry and other models is that it can 'think' before responding, rather than immediately answering queries. This thinking phase usually lasts 10 to 20 seconds."
Also, at the beginning of the article, we see that a ChatGPT Pro membership is now $200 a month.
The cost of reasoning is too damn high.
This is a typical case where in the diminishing marginal returns of miracles, reasoning costs are used to exchange for training costs to continue iterating the model.
This is also why OpenAI has always said, Strawberry is for the next generation of large models, used for synthetic data, because it is the carrier of Self-play RL.
So looking back, what might Strawberry be?
It is based on the new paradigm of Self-play RL, extremely strong in mathematical and coding abilities, and capable of autonomously executing browser/system-level operations for users.
More intelligent, slower, more expensive.
The last question is, why is Strawberry so explosively strong in mathematical and coding abilities?
The answer is very simple.
Because...mathematics and code are very easy to verify. In Self-play, clear results can be obtained. Mathematics aside, for code, whether you can run it or not is the verification, right?
So, these two things must have taken off first.
Why is Claude3.5's coding ability so awesome? It's done with Self-play RL.
I remember a few days ago, chatting with a very professional and awesome friend who specializes in AI investment. She had just returned from Silicon Valley and met with people from OpenAI.
An internal researcher at OpenAI described Self-play RL like this:
"There are no obstacles on our way to AGI."
After nearly a year of silence, we may be about to usher in a new cycle of large model technological breakthroughs.
Really.
I'm looking forward to it.
Digital Life Kha'Zix, original title: "ChatGPT Pro at $200 officially launched, the smart new model Strawberry is coming."