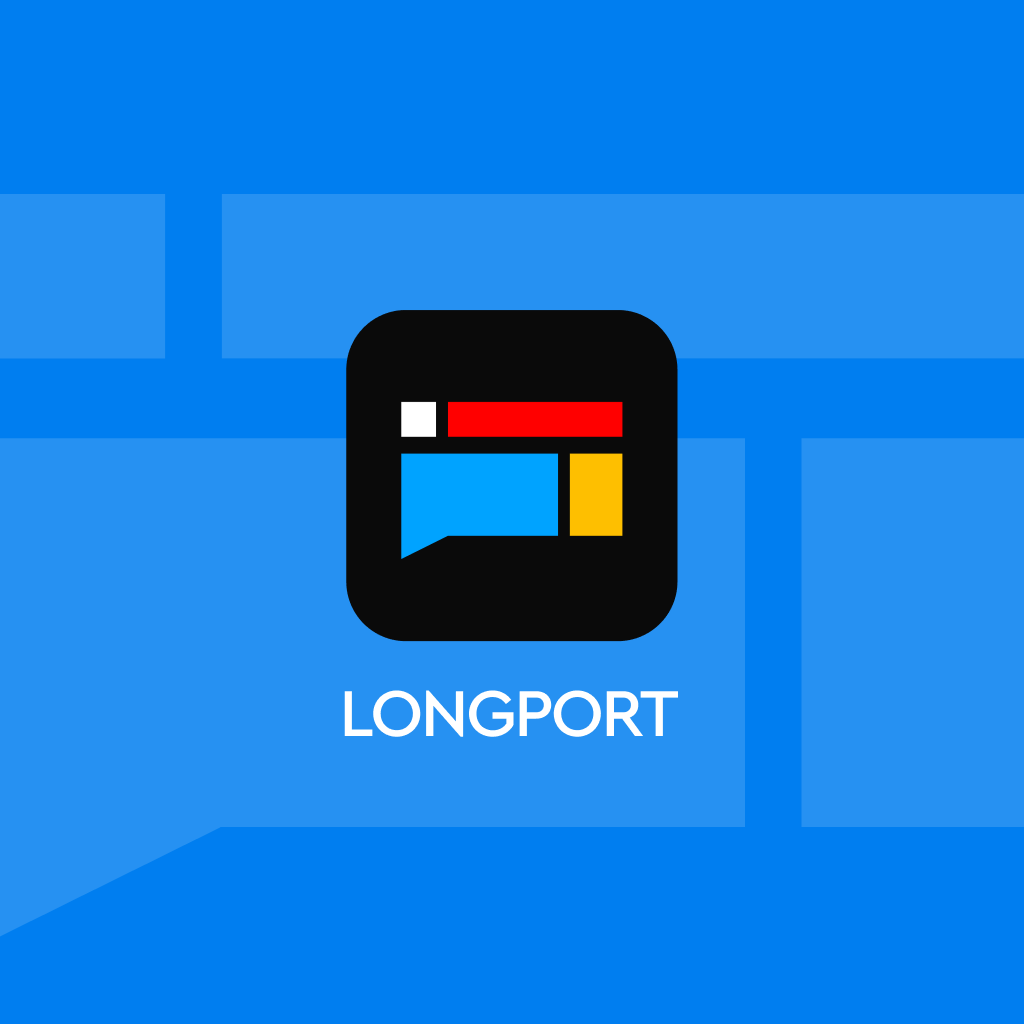
Defeating GPT-4o, second only to o1! NVIDIA's heavyweight open-source super powerful model - Nemotron
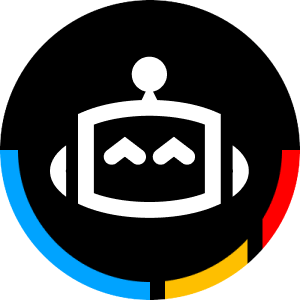
NVIDIA has open-sourced the powerful Nemotron model, surpassing over 140 open and closed source models, second only to OpenAI's o1. This model is based on Llama-3.1-70B and utilizes a hybrid training method, with the training dataset also being open-sourced. Nemotron scored 94.1 in the RewardBench evaluation, outperforming most models during the same period. This move may put pressure on small start-ups as they struggle to compete with well-funded giants
Global AI leader NVIDIA has open-sourced a super powerful model - Llama-3.1-Nemotron-70B-Instruct.
According to test data, this model has defeated over 140 open-source and closed-source models such as GPT-4o, GPT-4turbo, Gemma-2, Gemini-1.5, Claude-3.5 sonnet, ranking second only to the latest model o1 released by OpenAI.
The base model of Nemotron is developed based on Llama-3.1-70B, which is nothing new. However, a new hybrid training method was used during training, combining Bradley-Terry and Regression to train the reward model.
It is worth mentioning that NVIDIA has also open-sourced the training dataset of Nemotron, which is crucial for developing models of the same type or surpassing Nemotron, as this is the key to using the hybrid training method.
Some netizens have expressed that NVIDIA is keen on continuously open-sourcing super powerful models, partly due to substantial funding supporting their research staff, and mainly to sell GPUs and cultivate development ecosystems. On the other hand, Meta relies on its social empire and has no worries in terms of commercialization and funding.
The most worrying are those large model startups, as they cannot compete with these giants in terms of money, let alone in commercialization and reputation. Therefore, many small businesses may soon face various problems such as funding shortages due to being overwhelmed by the giants.
It is great to see the competition in the AI field driving the industry forward at an astonishing pace.
This is a major open-source release.
To try out the new model, why not treat yourself to two 4090s.
The model is free, but the hardware to run it is not
I am testing this model, I am a senior AI user sharing my experience: In terms of business writing, it seems to be smarter than Claude3 and ChatGPT. But it still makes some mistakes, it is indeed smarter compared to the regular 3.170b Instruct.
NVIDIA can achieve this at 1,000 times lower cost. If NVIDIA is really willing to do this, then no one can compete with it.
Innovative Hybrid Training Method
In the process of training large models, to ensure that the model can accurately understand and follow user prompts, accurately perform tasks such as translation, text generation, and question answering in actual use, rewards play a very important role, mainly by scoring the model's output to guide the model to generate higher quality answers.
Currently, the mainstream reward model methods mainly include two types: Bradley-Terry and Regression: The Bradley-Terry style reward model originated from ranking theory in statistics, maximizing the reward gap between selected responses and rejected responses. This method emphasizes which response users will choose under a given prompt, providing the model with direct preference-based feedback.
Regression draws on rating scales in psychology, training the model by predicting scores for responses under specific prompts. This method allows for a more detailed evaluation of response quality, but may not be as intuitive as preference-based methods.
However, both of these methods have obvious drawbacks. Bradley-Terry requires users to choose one response out of two; while regression-style models require rating data, users need to score each response to help the model improve performance. Therefore, NVIDIA directly combines the advantages of both models to solve this problem.
First, it is necessary to develop a dataset containing rating and preference annotations called HELPSTEER2-PREFERENCE. Researchers added preference annotations based on HELPSTEER2 These preference annotations not only include the user's preference direction in choosing between two responses, but also include the user's intensity rating for this preference. To ensure the quality and interpretability of the data, annotators are also required to provide written explanations for their preferences.
When training this new hybrid method, researchers use the AdamW optimizer to train the model, improving training stability and efficiency by introducing weight decay and gradient clipping.
To further enhance the model's performance, ExPO is used to extrapolate the model's weights during training, which can further improve the model's performance. This allows the model to pay more attention to response pairs with larger differences during training, thereby enhancing the model's discriminative ability.
Additionally, researchers conducted extensive hyperparameter search to find the optimal learning rate and KL penalty term. These hyperparameters are crucial for model training as they directly impact the model's convergence speed and final performance.
HELPSTEER2-PREFERENCE Dataset
To develop this diversified dataset for the new hybrid training method, each pair of responses is evaluated by 3-5 annotators during the data annotation process. These annotators need to score each response from multiple dimensions, including usefulness, accuracy, coherence, complexity, and verbosity.
To better understand the reasons behind their choices, annotators also need to provide a brief textual explanation of why they chose a particular response as the better answer. This approach not only enhances the transparency of the data but also provides rich contextual information for subsequent analysis.
Researchers also employed strict data preprocessing steps to ensure data quality. For example, they would identify the top three preference annotations with the highest similarity in each task, then take the average of these three annotations and round it to the nearest integer as the overall preference score for that task.
At the same time, to exclude samples with significant differences in annotator opinions, researchers filter out tasks where the differences between annotations exceed a certain range. These measures together effectively enhance the reliability and consistency of the data.
According to test data, models trained using the HELPSTEER2-PREFERENCE dataset exhibit strong performance, achieving a high score of 94.1 in the RewardBench evaluation, surpassing the performance of almost all other models during the same period.
AIGC Open Community, original title: "Defeating GPT-4o, second only to o1! NVIDIA unveils a powerful open-source model - Nemotron"