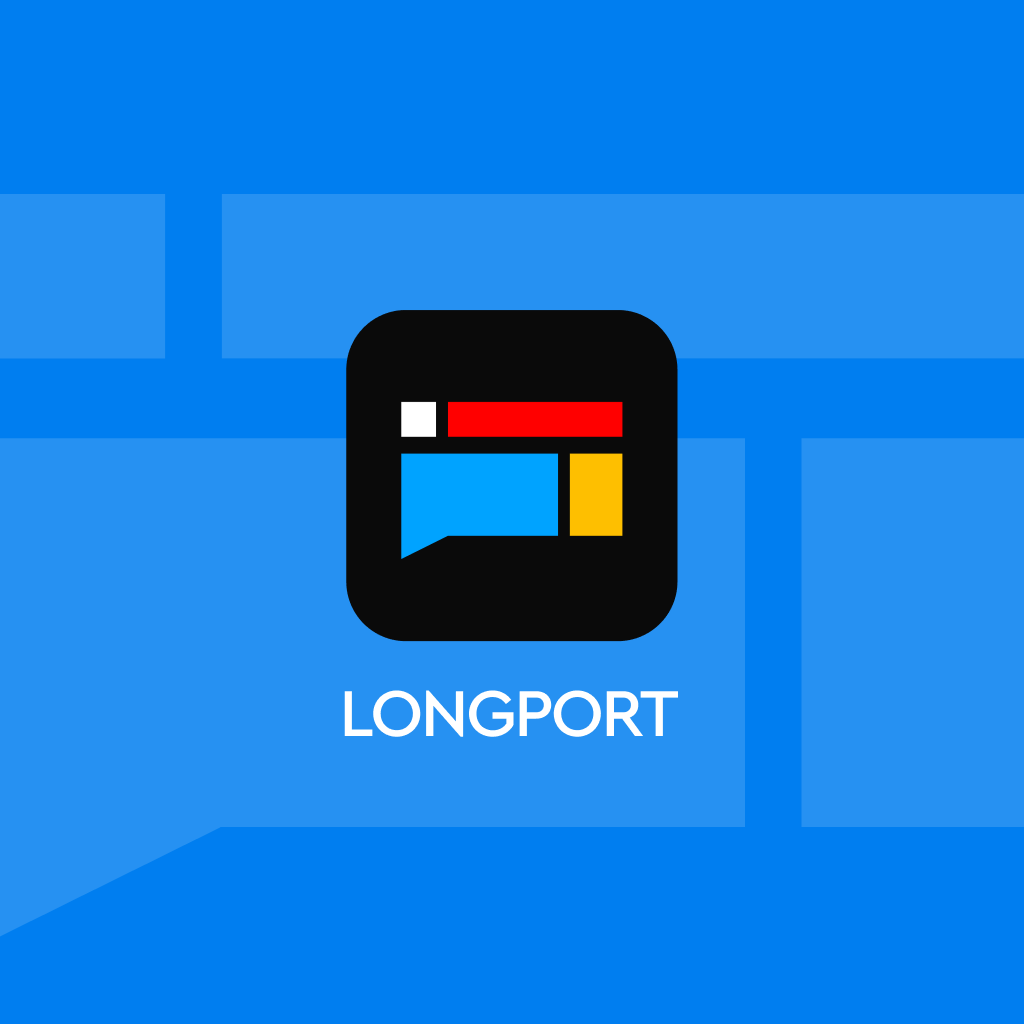
Scaling Law is diminishing, piling up data and computing power to compete with AI models is no longer feasible? Major companies are seeking a way out
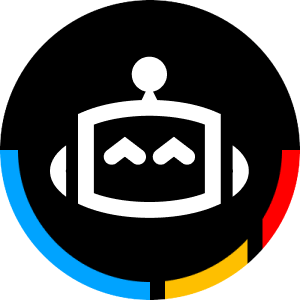
Analysis indicates that compared to traditional methods of training that rely on stacking computational power and data, a new approach known as "Test-time Compute" is more beneficial for enhancing the predictive capabilities of AI models. This method allows AI models to have more time and computational resources for "thinking" before answering questions. Experts point out that if test-time compute becomes the next step in scaling AI systems, the demand for AI chips focused on high-speed inference may significantly increase
Author: Zhao Yuhe
Source: Hard AI
AI laboratories moving towards superintelligent systems are realizing that they may need to change course. Analysis indicates that a new method known as "Test-time Compute" is more beneficial for enhancing the predictive capabilities of AI models compared to traditional methods that rely on stacking computational power and data.
According to TechCrunch, several AI investors, founders, and CEOs have revealed that the "AI scaling laws" used by AI laboratories to enhance model capabilities in recent years are showing signs of diminishing returns. Their views align with recent reports indicating that the pace of model improvement in top AI laboratories is not what it used to be.
Now, almost everyone is beginning to acknowledge that the approach of pre-training large language models solely by increasing computational power and data volume, and expecting them to become some sort of omniscient model, is not feasible. This may sound obvious, but scaling laws were a key factor in developing ChatGPT and enhancing its performance, and they may have influenced many CEOs' bold predictions that artificial general intelligence (AGI) would arrive within a few years.
Ilya Sutskever, co-founder of OpenAI and Safe Super Intelligence, stated to the media last week, "Everyone is looking for new ways to scale AI models." Earlier this month, Marc Andreessen, co-founder of Andreessen Horowitz, mentioned in a podcast that current AI models seem to be approaching their limits in capability.
However, CEOs, researchers, and investors in the AI field have begun to claim that the industry is entering a new era of scaling laws: "Test-time Compute" is considered a particularly promising new method that allows AI models more time and computational resources to "think" before answering questions.
"We are seeing the emergence of a new scaling law," said Microsoft CEO Satya Nadella on Tuesday at the Microsoft Ignite conference, referring to the test-time compute research supporting OpenAI's o1 model.
Additionally, Anjney Midha, a partner at Andreessen Horowitz, board member of Mistral, and former angel investor in Anthropic, stated in a media interview, "We are now in the second era of scaling laws, which is test-time scaling."
The Failure of AI Scaling Laws?
Since 2020, the rapid advancements in AI models achieved by companies such as OpenAI, Google, Meta, and Anthropic have primarily been attributed to a key judgment: using more computational resources and data during the pre-training phase of AI models.
During this phase, AI identifies and stores information by analyzing patterns in large datasets. When researchers provide sufficient resources for machine learning systems, the models typically perform better in predicting the next word or phrase The first-generation AI scaling law allows engineers to improve model performance by increasing the number of GPUs and the amount of data. Although this approach may have reached a bottleneck, it has changed the landscape of the entire industry. Almost every major tech company is betting on AI, and NVIDIA, which provides GPUs for these companies, has now become the world's most valuable publicly traded company.
However, these investments are made based on the expectation that scaling can continue to develop. After all, the scaling law is not a law of nature, physics, mathematics, or government; it is not guaranteed by anyone or anything to continue at the same pace. Even the famous Moore's Law has gradually failed after running for a long time.
Robert Nishihara, co-founder and former CEO of Anyscale, stated to the media,
"If you just invest more computational resources and data, and make the model larger, the returns will gradually diminish. To maintain the operation of the scaling law and keep the pace of progress, we need new ideas."
"When you have already read a million Yelp reviews, reading more reviews may not bring much gain, but that is pre-training. The methods regarding post-training are still relatively immature and have a lot of room for improvement."
Nevertheless, AI model developers may still continue to pursue larger computing clusters and larger datasets for pre-training, and these methods may still have some room for improvement. For example, Musk recently completed a supercomputer named Colossus with 100,000 GPUs for training the next generation of xAI models.
But trends indicate that simply using more GPUs with existing strategies cannot achieve exponential growth, so new methods are beginning to gain more attention.
Test-Time Compute: The Next Big Bet in the AI Industry
When OpenAI released the preview of its o1 model, it announced that this belongs to a new series of models independent of GPT.
OpenAI primarily improved its GPT models through traditional scaling laws (i.e., using more data and more computational power during the pre-training phase). However, it is said that the gains from this approach are no longer significant. The o1 model framework relies on a new concept—test-time compute, named so because computational resources are used after receiving prompts (rather than before). Analysts believe that this technology has not been explored much in the context of neural networks but has already shown potential.
Some have already viewed test-time compute as the next method for scaling AI systems.
Midha from Andreessen Horowitz stated,
Many experiments show that even if the "pre-training" scaling law may be slowing down, the "test-time" scaling law—providing more computational resources to the model during inference—can still significantly enhance performance.
Renowned AI researcher Yoshua Bengio remarked,
"OpenAI's new 'o series' further promotes [coherent thinking], requiring more computational resources, and thus more energy. We are seeing a new form of computational scaling: not just more training data and larger models, but also spending more time 'thinking' about the answers." For example, in a time frame of 10 to 30 seconds, OpenAI's o1 model repeatedly prompts itself to break down a complex problem into a series of smaller questions. Noam Brown, who is currently responsible for OpenAI o1, is attempting to develop a poker AI system that can beat humans. In a recent speech, Brown noted that human poker players take time to consider different scenarios before making a move. In 2017, he introduced a method that allows the model to "think" for 30 seconds before making a move. During this time, the AI simulates different sub-games and deduces possible outcomes of various scenarios to determine the best action. Ultimately, the predictive performance of this AI improved sevenfold compared to his previous methods.
It is important to note that Brown's research in 2017 did not use neural networks, as they were not yet widespread at that time. However, last week, researchers from MIT published a paper indicating that computational improvements during testing significantly enhanced the AI model's performance on reasoning tasks.
It remains unclear how to scale up the computational testing. This could mean that AI systems require a very long "thinking" time to solve difficult problems, potentially hours or even days. Another approach might be to allow AI models to "think" about problems simultaneously on many chips.
Midha stated that if computational testing becomes the next step in scaling AI systems, the demand for AI chips focused on high-speed reasoning may increase significantly, which is good news for startups like Groq or Cerebras that focus on fast AI reasoning chips. If finding answers requires as much computational resources as training models, then "mining tool" providers in the AI field will benefit once again.
Regardless of the cutting-edge developments in AI research, users may not feel the impact of these changes for some time. However, AI developers will spare no effort in rapidly launching larger, smarter, and faster models, which means that several leading tech companies may adjust their approaches to pushing the boundaries of AI.
This article is from WeChat public account "Hard AI". For more cutting-edge AI news, please click here.