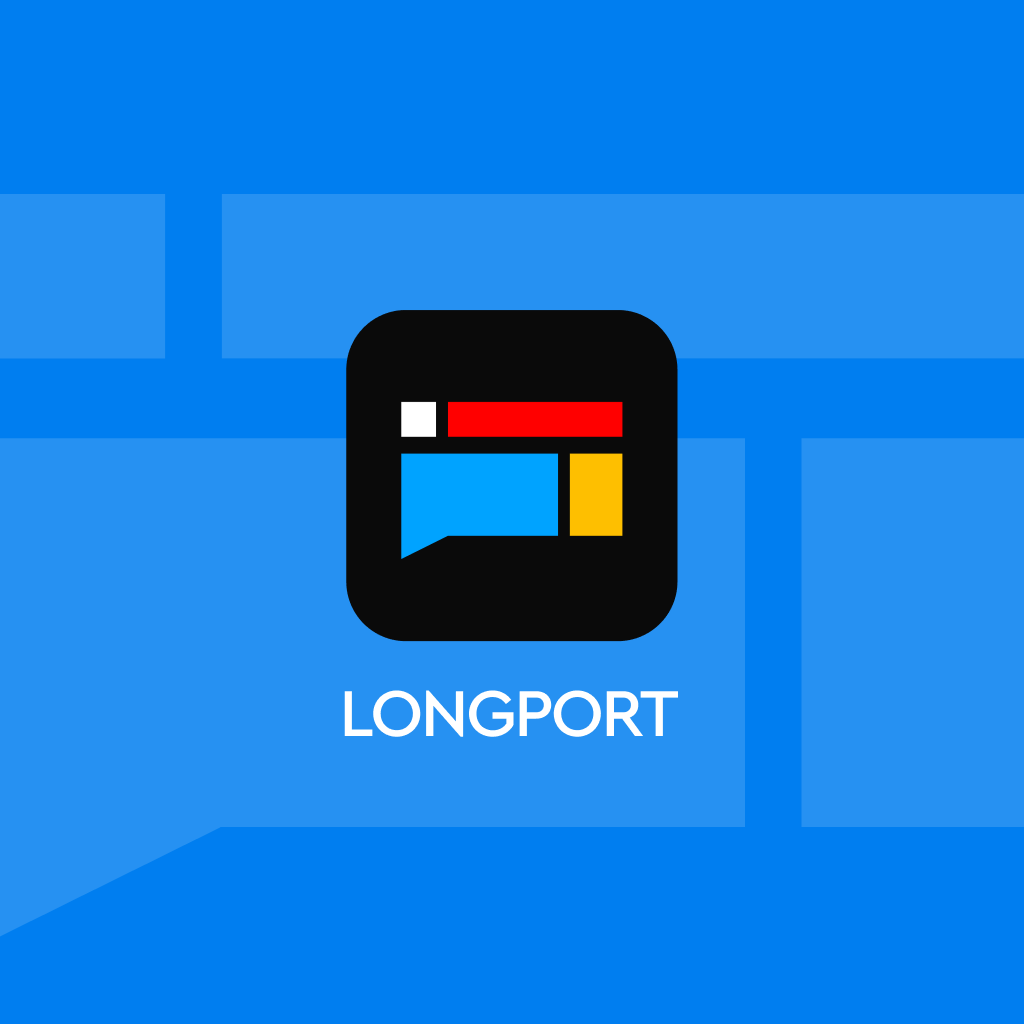
That quantitative big shot who hoarded tens of thousands of chips has really started to take action
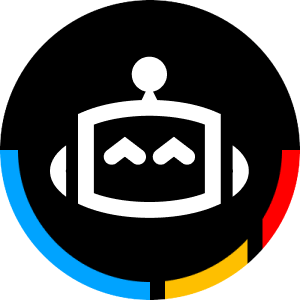
Key "Role Playing"
In China's quantitative circle, Huanfang Quantitative and its founder Liang Wenfeng are both "unique" entities.
They not only engage in asset management but also conduct proprietary investments and dare to directly challenge the artificial intelligence business.
Months ago, the large model product led by the Deep Exploration Company initiated by Liang Wenfeng participated in market competition with the image of "the Pinduoduo of the AI world," directly prompting major internet companies like Alibaba, ByteDance, and Baidu to "reduce prices in response" for their AI products.
However, news from third-party channels indicates that Huanfang's latest management scale has been surpassed by a "rising star."
What are they thinking?
"Diminishing" Asset Management Scale?
Huanfang Quantitative was once the first quantitative giant in China to break through the trillion yuan mark in private equity, and accurately speaking, it is still the only quantitative private equity firm in the industry that has crossed the trillion yuan threshold.
However, sources close to Huanfang Quantitative have revealed to Zhi Shi Tang that this former trillion yuan private equity firm has seen its latest management scale drop to the range of 20 to 30 billion yuan.
Moderately controlling scale has been a practice among leading quantitative firms over the past year. But currently, Huanfang is the "most resolute" in this regard.
At the same time, the AI large model company "Deep Exploration," founded by Liang Wenfeng, continues to expand, with ongoing internal team growth and external recruitment.
Moreover, it is said that this AI company has rented several thousand square meters of office space in a high-end office building near Peking University, operating in the same area as Silicon Valley giants, demonstrating a strong ambition.
Is Liang Wenfeng shifting the future business focus to AI?
From "Peripheral" to "Core"
Deep Exploration was once thought to be a "peripheral company" of Huanfang Quantitative.
It has since been proven that it is just another company initiated by the actual controller of Huanfang Quantitative.
In the quantitative field, the actual controller may initiate multiple companies, each bearing its own business direction and function. However, there are often subtle clues regarding personnel and shareholders.
Deep Exploration's AI products officially debuted to the public in 2023, but in fact, the company had been internally "incubating" for several years prior.
This company even recruited liberal arts talents externally, with the position titled "Data Encyclopedia," providing knowledge sources related to human history, culture, science, etc., to build a comprehensive world language knowledge base together with data engineers.
This type of recruitment once attracted the attention of the Chinese fund circle.
Another noteworthy matter is that there have been industry rumors that as early as 2019, Huanfang and its backing funds began to invest heavily in deep learning training platforms. By 2021, Huanfang Quantitative's investment in supercomputing cluster systems increased to 1 billion yuan, equipped with over 10,000 NVIDIA A100 graphics cards.
This may have made it one of the companies in mainland China with the most AI graphics cards at that time, and it could even be counted among the single digits globally.
According to public information: Microsoft's Azure cloud service built an AI computing cluster for ChatGPT with over 10,000 NVIDIA A100 chips. In earlier years, companies including Tesla and Facebook utilized A100 chips to establish AI computing clusters, with procurement scales exceeding 7,000 units Liang Wenfeng has stated externally: There are no financing plans for the large model business in the short term; the issue has never been money, but rather the acquisition of high-end chips.
Latest "Details" on Large Models
On December 10th, DeepQiuSuo Company disclosed details on the capability enhancements of large models in various application fields, mentioning six application scenarios:
Role-playing, reasoning, writing, coding, mathematics (specifically mentioning high school mathematics competitions).
Among these, the iterative improvement in the writing scenario is the most significant.
The organization also introduced that the models developed have surpassed many well-known peers in authoritative evaluations such as the American Mathematics Competition and global top programming competitions.
In September of this year, DeepQiuSuo Company also "showed off" that it achieved the best historical results for domestic models in many subcategories, such as ranking second in the world for coding scenario applications in third-party evaluations and third in the world for mathematics scenarios.
This company also announced that multiple application subcategories ranked first in China on its own platform.
Due to technical capabilities and professional information limitations, we cannot provide high-dimensional commentary on the "ranking performance" disclosed by DeepQiuSuo Company.
What is "Role-Playing"?
In the above image, "role-playing" ranks as the top item in DeepQiuSuo's application enhancement display.
ZiShiTang has found that in all articles published by DeepQiuSuo's official WeChat account, "role-playing" is mentioned five times, but without in-depth explanation.
Information shows that users can set a specific role or behavior for the model through specific system prompts, allowing it to exhibit behavior, tone, knowledge, and skills consistent with the designated role during conversations.
In simpler terms: it allows the large model system to play/pretend to be an identity, such as a fund manager, doctor, teacher, or detective, and then think, speak, and solve problems according to that identity.
In fact, this scenario has a certain demand for public and private fund institutions, as the large model can "transform" into a fund manager or financial manager, which is a key application of "simulating a real person," or directly impact the team configuration of fund institutions.
What Happens When "Playing the Fund Manager"?
ZiShiTang issued a command to the large model, conducting a "fund investor dialogue with the fund manager" role-playing experiment to see how this "fund manager" would "persuade" the investors.
Investor's question: Given the current market volatility, should I sell my equity funds?
"Fund Manager" replied: Whether to sell equity funds during market volatility depends on your investment goals, risk tolerance, and market judgment.
If your investment goal is long-term returns, then short-term market fluctuations may just be a temporary phenomenon, and there is no need to rush to sell. Historical data shows that holding quality equity assets for the long term usually outperforms market volatility The "fund manager" further responded: If you still feel uneasy, you might consider redeeming in batches rather than selling all your fund shares at once. This approach can reduce losses caused by misjudgment. At the same time, consider transferring some funds to low-risk assets (such as bond funds or money market funds).
A fund investor asked: I bought the CSI 300 ETF. How should I adjust my strategy during significant market fluctuations?
The "fund manager" replied: If you are a long-term investor, continue to hold or gradually increase your position. If you have idle funds, consider gradually buying in during market corrections, and it is recommended to invest more funds in stages when the index declines significantly (such as a 5%-10% adjustment).
This "fund manager" also suggested: You might consider allocating to industry ETFs, transferring some funds to lower volatility industry ETFs (such as consumer and healthcare), to diversify risks while retaining the return potential of equity assets. They also mentioned increasing allocation to policy-benefiting industries and reducing exposure to cyclical industries.
The above content is just a partial transcript of this role-playing scenario, and the operational advice provided by the "fund manager" contains more detailed content.
From this perspective, it can be seen that the role-playing scenario can further replace many of the "scripts" used by bank wealth management managers and the marketing departments of fund companies.
In this regard, although AI companies are relatively independent, there is considerable potential for deep exploration and development, and many applications can be found in the asset management industry