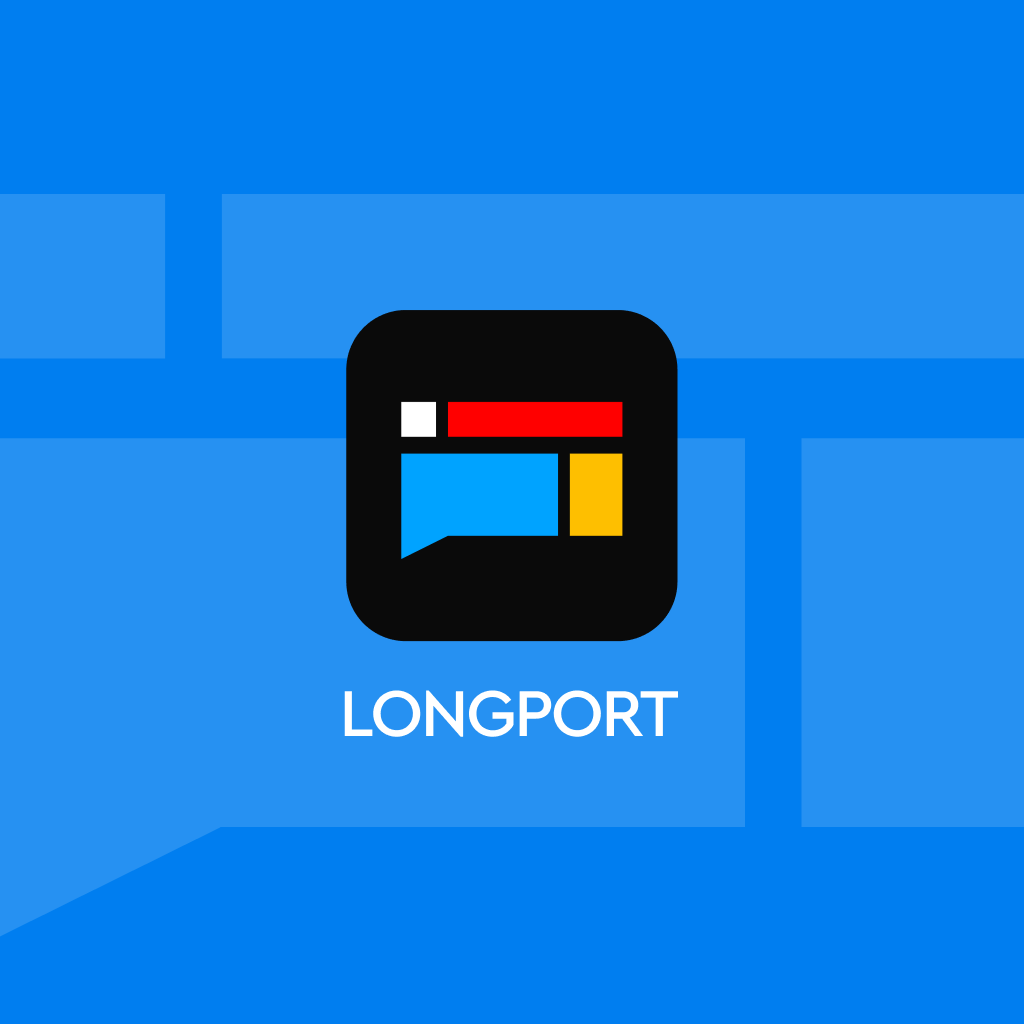
Google Gemini head explains: How to understand the next step of AI?
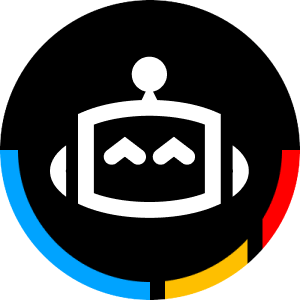
Oriol Vinyals, co-head of Google Gemini, discussed the future of AI models in a DeepMind podcast, emphasizing algorithm generality and data limitations. He pointed out that AI training includes two stages: pre-training and reinforcement learning. Although the details differ, the principles remain unchanged. He believes that the key to advancing AGI lies in giving computers a digital body to achieve more complex tasks and personalized services. At the same time, he mentioned that the accumulation of innovation is crucial for model iteration
Recently, Oriol Vinyals, Vice President of Drastic Research and Co-Head of Gemini, shared his views on AI models during a podcast interview at Google DeepMind, discussing the processes behind multimodal models, the importance of innovation, and the next steps for AI.
He believes that the current challenge for AI is achieving algorithmic generality. He also stated that there is no such thing as an infinite data state in pre-training; data is limited. He tends to believe that in the future, it may be possible to slightly push the limits of data beyond the current boundaries and break some scaling laws and limitations observed in pure pre-training.
Here are the key points from the interview:
In terms of the algorithms themselves, we strive to make them very general so that we can continue to climb the difficulty ladder, game the curriculum, and do more complex things.
AI training has two fundamental steps that have remained relatively unchanged: the first step, which is pre-training or imitation learning, and the reinforcement learning or fine-tuning part, which is the second phase of training. These two steps are essentially the same from AlphaGo to AlphaStar to the current large language models. Of course, there are some important details, and this field has evolved, but the principles have hardly changed.
The computational units are neurons, and the connections between neurons are essentially weights. So you can imagine having a neuron with several other neurons connected to it. You are essentially multiplying the activation values of all incoming neurons by the weights.
These models actually do some things, take actions, and learn anything new that is available, which is quite powerful. This is the biggest factor driving generality, which is what many people refer to as AGI, feeling closer.
To push the frontier, you need to give the computer a digital body so that it can not only think, give instructions, or produce text output, but also do some things online or on files you might upload, or ask very complex questions and personalize for you, etc.
Because the process of training models is expensive, we need to be very careful in accumulating innovations so that ultimately, when we are ready, we have enough innovations and possibly better scales to run the next iteration of the model. We run it, and then we achieve algorithmic breakthroughs not only through data and computation.
In pre-training, we do not have what is called an infinite data state; data is limited.
We only have limited data to train this arbitrator, and the real standards may require expert judgment. However, this approach is not scalable.
By providing these tools to the model, they can begin to achieve more higher-order functions that go beyond the training corpus, such as relying on the latest news to explain or summarize significant events from the previous day.
We hope that by extending the model's processing time, it can better summarize news, write poetry, and even solve math problems. But this is definitely another scaling axis that we are starting to unlock, and we hope to unlock. Similarly, we will break some scaling laws and the limitations we see in pure pre-training. **
When you need to consider personalization and scheduling, the model needs to integrate data from multiple information sources to provide the best answer. This is no longer a simple question of 'what color is the sky.'
A breakthrough this year is the ability to handle millions of tokens in context, allowing you to retrieve something from the past and bring it into the future for very detailed analysis.
The following is the original interview text, with some content slightly edited:
Two Basic Steps of AI Training: Pre-training and Reinforcement Learning
Host:
The last time I saw you, you were researching an intelligent agent that could use a keyboard and mouse to draw, paint, or play StarCraft. Since then, a lot has progressed.
Oriol Vinyals:
What we were doing at that time was developing a series of increasingly difficult tasks. When we talk about the video game StarCraft, it is one of the most complex modern strategy games today. Of course, DeepMind is known for pioneering the trend of Atari games, which is a simple game of moving a paddle left and right to hit a ball.
This is the algorithm itself, and we strive to make them very general so that we can continue to climb the difficulty ladder, game courses, and do more complex things. What is happening now is that the models we train have a broader range of applications than the models we developed back then.
So think about the process of creating this digital brain hasn't changed much. But the capabilities of that brain at the time were relatively limited, although very complex, such as playing StarCraft or Go. Now, these models can do a much wider range of applications, and of course, there are chatbots that chat with us, and so on.
Host:
At that time, reinforcement learning was your main leverage. I wonder what is different now?
Oriol Vinyals:
Yes, so algorithmically, AlphaGo and AlphaStar used the same sequence of algorithms to create this digital brain. It is not much different from how current large language models or multimodal models are created. In many of the projects we are involved in, there are two basic steps that have remained relatively unchanged, the first step, which is pre-training or imitation learning.
That is to say, starting from random weights, there is an algorithm that tries to imitate the vast amount of data created by humans for playing games, or in this case, to imitate all the knowledge we can access on the internet. In this first stage, you simply adjust the weights to mimic that data as well as possible.
Host:
These weights are essentially a series of numbers inside each neuron that describe its connections to other things?
Oriol Vinyals:
Yes, so basically the computational units are neurons, and the connections between neurons are actually the weights. So you can imagine there is a neuron with several other neurons connected to it. You are essentially multiplying the activation values of all incoming neurons by the weights. And these weights are the only things that will change. The input stimulates the neurons, which is very similar to how the brain works, with some degree of creative freedom Host:
Well, if we make an analogy, it's like you have neurons, and water flows through them, while the weights are like the width of the pipes between the neurons?
Oriol Vinyals:
Yes, exactly. Then you can imagine millions of neurons and billions or even trillions of pipes. This is where we spend most of our computational resources training these models, especially language models, during pre-training or mimicking all the data we can obtain.
Host:
Okay, so now you have a huge network with many pipes connecting all the neurons. That's your imitation phase completed. Next, if you're doing something like AlphaGo or AlphaZero, you would let it play against itself.
Oriol Vinyals:
Yes, of course, these sentences make logical sense in English. Or if it's playing a game, it will reasonably click on things to move pieces on the board, and so on. But what this model hasn't learned yet is that learning these behaviors will yield rewards.
That's the reinforcement learning or post-training part, which is the second phase of training. So you can write a poem by asking, "Hey, what does poetry on the internet generally look like?" But the next question is, "I only want the good parts."
So how do I further adjust these pipes based on some signal? Now, after writing a complete poem, it will give a score of 0 or 1.
For example, if it's a mediocre poem, you get 0 points; if it's a good poem, you get 1 point. Using a game analogy, this is where we traditionally use reinforcement learning: if you win the game, you get 1. If you lose, you get 0, and then you further adjust the weights.
But now, it's no longer about mimicking humans; it's about saying, forget the past, I want to surpass what humans can do, trying to make all my poetry perfect poetry, or all my chess games perfect games. In language models, this second phase, the reinforcement learning post-training phase, is often relatively short because we don't get super clean rewards.
Host:
So once that's done, that's all the behind-the-scenes work. Then you say, everyone hold still. We are going to take a snapshot of the entire network, and this is what you as a user can actually access.
Oriol Vinyals:
Yes. So now this amazing process is complete. These weights are very precious, so the configuration you find, you really spent months perfecting it, tuning everything. So the training is over, and you no longer change the configuration.
You might want to make it very efficient. For example, if you find that this neuron is not very useful, it doesn't contribute to anything, you remove it, and everything becomes faster and cheaper to run at scale.
Then as a user, you get the same weights; everyone gets the same weights that we have trained. This is what we call Gemini 1.5 Flash. It simply means a set of frozen weights that will not be further trained or anything So these two steps are actually almost the same from AlphaGo to AlphaStar to the current large language models. Of course, there are some important details. And this field has developed, but the principles have hardly changed.
AGI is the biggest driver of generality
Host:
This is the example of Atari, or the type of algorithms used in AlphaGo, or in large language models, the architectures are different, right?
Oriol Vinyals:
Yes. So there are some components that make up the digital brain. One of them is the architecture, so there are these neural networks. Now we have transformer models, which we definitely didn't have in the DQN era. So there are always some architectural breakthroughs that allow us to learn better from data.
But from transformer models to today, it's almost all small adjustments. Even if you look at AlphaFold, it is also driven by transformers, and the team sometimes needs years just to find some small adjustments, like, "Hey, let's remove this set of neurons, let's add another layer, let's make this a bit wider," so you shape the brain's form, it changes slightly, and sometimes this affects the performance realization.
Host:
If these are the achievements so far, my understanding is that the goal is to create more agent behaviors that allow these things to make autonomous decisions. How do these help achieve that goal?
Oriol Vinyals:
Yes. So let's dive a bit deeper into the current trends. We call them large language models, but they are multimodal.
Imagine being able to add images and then ask questions, continue to ask questions, how great that would be. So this skill, we will still improve it. These weight sets can make these amazing inferences about the input. What is this image? What is the user asking? Can I write a better poem? Can I make it longer or something else?
Just like the interactions we have now, we can all play with these things, but this is just one component, and we can think, hey, this is now our central processing unit, and we can add more content around it.
What if the model could do research for you? For example, one example I gave, we started thinking about this a long time ago. I could ask a language model or a vision-language model to learn to play StarCraft. This is a very different approach from creating an agent that plays the game; in another example, it could go online, watch videos about the game. Of course, it could download the game and start interacting to learn.
Research online, go to forums, read forums. Go play and discover where it is weak and improve it. After a few weeks, it might send you an email saying, "I now know how to play this game, let's play." This is not too far-fetched a reality.
But these models actually do some things, take some actions, learn anything new that is available, which is quite powerful. This is the biggest driver of generality, and this is what many people refer to as AGI, feeling closer.
Digital Bodies Can Expand Answers
Host:
So if I understand correctly, what we currently have, large language models, multimodal models, whatever you call them, is the core. But the next step is to build something on top of this core, allowing it to shed its stabilizers and do its own thing.
Oriol Vinyals:
Yes, that's indeed the case. If it can access all knowledge and utilize its time for some real research, formulating hypotheses, writing some code, etc., and spend time truly answering very, very complex questions, the possibilities have greatly expanded.
Although, of course, we don't need this for everything. If we ask a question like, "Hey, I like rice. What should I prepare tonight?" it may not require deep thinking or three weeks of research; you might be dissatisfied with the wait time.
But I think, to push the frontier, you need to give the computer a digital body, so it can not only think, give instructions or text output, but also do some things online or on files you might upload, or ask very complex questions, and personalize for you, etc.
Host:
I love this idea; you have an electronic brain, and now you give it a digital body. I know one of the big ideas behind large models is to scale them up, make them larger and larger. Do you think the results seen through scaling have stabilized now?
Oriol Vinyals:
Yes, that's a very important question. We have studied how, as models grow larger, meaning how many neurons these models have, they excel in certain tasks where we have clear metrics. For example, a very easy-to-understand example is machine translation, so when you scale from millions to billions and possibly even trillions of neurons, you see performance continuously improving.
This means, over the past three years, we've made some progress, and you shouldn't expect the same level of progress in the next three years. In fact, this path is becoming increasingly difficult. This means that computational input is also advancing at a superlinear rate, but perhaps not as well as these trends suggest, and you will see some diminishing returns.
Because simply scaling the x-axis, i.e., the number of parameters, you need to increase it tenfold to see the same improvement. This puts some pressure on us, saying, hey, maybe we can't scale that much, and we need to consider other ways to scale to make the models better.
In fact, this analogy can even apply to the performance of the models. Even if you have very good performance, if you want these models to be completely accurate and not fabricate facts. But in reality, sometimes testing can reveal that they produce inaccurate content. Achieving complete accuracy remains very challenging, which presents some interesting challenges for large-scale deployment.
Host:
I hear what you're saying about diminishing returns. But in terms of how to make these things better, how to improve these models, is it just data, computational power, and scale? Are these the only levers that need to be pulled? Oriol Vinyals:
Yes. If you freeze the architecture, for example, if there is no innovation in the next year and we just expand because better hardware comes out, there will definitely be a trend that looks good. But what happened, especially with Gemini, is that we have other innovations, other tricks, technologies, details about how to present model data in what order, details about the architecture, how to run the training process, how long to run it, what kind of data we actually show to the model? How do we filter? We show more high-quality data and show less low-quality data, all the different things we call hyperparameters.
Of course, there are other algorithmic advancements that we are also studying very carefully, because the process of training models is expensive. So we need to be very careful in accumulating innovations so that ultimately, when we are ready, we have enough innovations and possibly better scale to run the next iteration of the model. We run it, and then we not only achieve algorithmic breakthroughs through data and computation.
The data in training AI is actually limited
Host:
I think another thing about this scaling is that there are no real limits; the number of nodes you can input is actually unlimited, and perhaps theoretically, the computational power input has no limits. But the data you can input is limited. The number of human languages is finite.
Oriol Vinyals:
Good point. So I think the nodes are limited because the way you scale these models is that they cannot run on a single chip. So now you have a chip grid. They are communicating. There are some limitations, like the speed of light, etc. So there comes a point where the efficiency of training such a large model is not worth it, even from the utilization of the hardware at hand.
Another key point in this pre-training is that mimicking all the data, we do not have what is called an infinite data state; the data is limited. Therefore, once the model needs—imagine, let’s train on all the data. If you want to train on everything, the entire internet. So we are just starting to think that we are running out of data. Are there some techniques, like synthetic data, can we write or rewrite existing data in multiple ways?
Language is an obvious avenue; you can write the internet in different ways. It is primarily written in English, but there are ways to rewrite the same knowledge in different ways. We are exploring these. This is a research area that many people are starting to invest in. Because if you run out of data, scaling laws will further penalize you.
Host:
So, for example, can you have Gemini write its own version of the internet and then use it to train a new version of Gemini? If you start inputting the outputs of the same model, is there a danger of creating small, less helpful feedback loops?
Oriol Vinyals:
They can certainly do some interesting experiments to test the ideas you just mentioned. Indeed, on the surface, it does not seem like a good idea. If you let the model recreate the entire internet, the model will suffer. ** Indeed, from the perspective of information and content, this dataset has the information it possesses. How can you create new information, right? I don't know, these ideas might be helpful; we have not yet reached the fundamental ability to truly extract all information from the internet. We have good algorithms, but they are not perfect.
Host:
If you could find the E=MC² of human concepts and then generate new data solely from it, that seems more realistic.
Oriol Vinyals:
Yes. Do these language models just repeat content online and cannot create anything new? Or are they learning a world model, and then you can extract principles based on it, possibly surpassing the scope of the data contained? In a more optimistic version, I tend to believe that we can slightly push the limits of data beyond our current limits.
However, there are some data sources where we have not seen breakthroughs, such as video data. Despite the vast amount of this data, we have not found a breakthrough that can extract a lot of knowledge and physical laws from all videos, even when there are no textual descriptions in those videos. Even so, I don't think we have fully utilized that source.
Host:
Isn't it supposed to work that way? Or you don't know?
Oriol Vinyals:
Yes. It feels like it should. There was some early language learning, but we also learn by observing the three-dimensional world, and so on. So there may be more knowledge that we have not extracted yet. Obviously, we have done well; you can see through testing the model that it connects concepts in videos. Then you can do some great things, like, "Hey, extract three interesting moments from this complete video." But the model itself, has it really utilized that information? Probably not yet.
Host:
If I understand correctly, currently it can tell you what is in the video, but it cannot then say "E = MC²." Or if you show it a photo of the night sky, it won't suddenly be able to predict planetary motion like a human astronomer.
Oriol Vinyals:
Yes, that's true. The shortcut we take here is that when we train images or videos, they almost always come with textual descriptions. So it might be an explanation of what this image or video contains, and so on. Of course, that's amazing.
You can put a photo of homework and a small concept diagram, and it will connect them and make a lot of good logic based on that. But what I'm saying here is, can we train a model to understand what is happening solely through video, without the aid of language, and even derive a kind of language (of course not our language) and extract concepts? This has not been achieved yet, but it might be possible.
Host:
Going back to the model built by DeepMind that you mentioned at the beginning, there are basically two stages.
Oriol Vinyals: Yes.
Host:
The imitation phase, followed by the reinforcement learning phase. Alpha Go and Alpha Zero, along with many other models, improve through self-play. Does this apply here as well?
The model will look for bugs to master the game
Oriol Vinyals:
Yes. This is one of the main open challenges, not just pre-training but also post-training or reinforcement learning. The beauty of reinforcement learning in games lies in the clear set of rules.
If you win, you know you’ve won. For example, in chess, if you win, the program verifies all the steps, confirms the checkmate, and congratulates you.
However, in language, it becomes trickier. For instance, is this poem better than that one? Even among us, it’s hard to reach a consensus. Therefore, this generalization makes precise calculation very difficult. How do you evaluate whether this is a better summary of a movie? Or is this the most interesting part of the video? It’s hard to quantify, but we try to do so. You train a model based on some people's preferences and ask it to generalize. Let the model critique its own output, and the results may not be too bad; perhaps it performs reasonably well 80% of the time. Although this is not perfect, it can provide some signals.
However, when you start training based on an imperfect reward model, the model will exploit the weaknesses of the reward. For example, in chess, suppose a pawn in a certain position always wins, and that position is one that no one would play. Then, the algorithm might discover this and exploit it to win the game. Even though the algorithm has mastered the game, from the researchers' perspective, this strategy is not ideal.
So that’s the challenge. Essentially, you are looking for loopholes rather than truly understanding what constitutes a good strategy.
Host:
Can you solve this problem by adding another model as the ultimate arbiter?
Oriol Vinyals:
Good suggestion, but the question is, how do you train that model? We only have limited data to train this arbiter, and the true standards may require expert judgment. However, this approach is not scalable.
Imagine if we completed parameter updates in 3 seconds and then asked experts to review these 10,000 items because that’s a reliable source. We don’t have enough data to train a sufficiently good reward model. Therefore, while there are some ideas, we cannot obtain real standards.
Breaking some scaling laws and limitations
Host:
And now we are building digital bodies. What kind of capabilities do you want this digital body to have, such as reasoning, because there’s a lot of work in that area as well, isn’t there?
Oriol Vinyals:
Yes. So when you start to think, can we give these models limited access so they can see beyond their weights, which are frozen, to gather knowledge or do something potentially more complex, rather than just predicting the next word based on what they have in context and what they have in weights? It is therefore obvious to grant them access to search engines. This is what we excel at in Google. Additionally, giving them the ability to run code they write themselves, and more broadly, enabling them to interact with browsers that have internet access.
In all these processes, you must be careful with the sandbox, which means protecting these environments to ensure that even if the models are not that advanced, they do not perform unintended actions. Therefore, when the models exceed their training, security issues become more concerning. But if we just dream about what is possible, by providing these tools to the models, they can begin to achieve more advanced functions that go beyond the training corpus, such as relying on the latest news to explain or summarize significant events from the previous day. For all these things, you need to give them these tools.
Host:
So, how does reasoning fit into all of this?
Oriol Vinyals:
Yes. Reasoning is interesting, right? What I just described can be summarized as: if I want to understand what happened yesterday, I can say, "Hey, model, I’m Oriol, I’m interested in these things, and my political views are this or that. Give me a positive perspective on yesterday's news." Then the model might search, retrieve all the news, and present it in a way that I like according to my request. If I’m not satisfied, I can also provide feedback saying I don’t like this or that joke, and then adjust in the conversation.
Now, reasoning is a different axis of expansion. So you can imagine the model determining which intermediate steps to take to give me a better answer. Imagine Google Search retrieving information from about a hundred news outlets; the model might decide not just to read and summarize everything simply, but to summarize each article one by one first. This means the model will summarize each article for itself rather than directly giving it to the user.
Then, it might group these summaries by topic and even further verify certain seemingly suspicious articles, such as checking online discussions. This multi-step research process can take a long time, and only when the model believes it has reached a higher quality answer will it provide a concise summary.
At this point, the model has ample time to process information and reason. We hope that by extending the model's processing time, it can better summarize news, write poetry, and even solve math problems. But this is definitely another axis of expansion that we are starting to unlock and hope to unlock. Similarly, we will break some scaling laws and the limitations we see in pure pre-training.
Host:
Does this also include planning capabilities? For example, can the model browse your calendar, calculate your payday, and remind you to postpone holiday bookings before the upcoming January discount season?
Oriol Vinyals:
This could become very complex. When you need to consider personalization and scheduling, the model needs to integrate data from multiple information sources to provide the best answer. This is no longer a simple 'what color is the sky' question. I recall an example mentioned in one of our early papers, where it was surprising that language models could spontaneously answer without programming instructions. However, when it comes to reasoning and planning involving planetary positions, time, weather, etc., the answers can become very nuanced. So thinking and planning, these models can do that.
Host:
I remember a conversation from 2019 where the other party talked about two thinking systems in the human brain: one is fast and intuitive, while the other is slow and calculative, like doing math and playing chess. The second is easier to implement with computers, but now we are also starting to see the possibility of fast intuitive responses. You are talking about combining the two, right?
Oriol Vinyals:
Yes, indeed. He might also be talking about systems, which is indeed an aspect you think about more. This is evident in games; you act on what feels right intuitively, but careful consideration may lead to better decisions. The challenge lies in the generality of these models.
To add thinking capabilities on a very general functional basis, you might need a universal way of thinking. Therefore, you use the model itself to generate how it should think about anything. Then the model will come up with, I want to summarize each article, I want to do this and that. This is not us programming; this is a very profound insight. Is this the only way? Is it the optimal way? It is still in the early stages, five years from now. We will wait and see.
AI Achieved Memory and Can Perform Deep Analysis
Host:
You are talking about planning and reasoning, and memory is another very important issue. Has it been achieved? People often talk about long and short contexts. I think this is somewhat related to working memory, isn't it?
Oriol Vinyals:
Yes, there are some techniques that can be applied to language models, at least three of them, and they are quite easy to explain. The first method we have for a system that remembers the entire internet is through executing pre-training steps. This is actually a specific format of memory step; we have these weights that are random, and then we assemble them into these amazing architectures.
The second level, I might have explained a bit. How to provide tools like Google and other search engines to the model. You could say this is what neuroscientists refer to as contextual memory, as humans, perhaps like we had these memories a long time ago.
They are not very precise, so they tend to be a bit vague. If I had to think about what my first day at Google was like? I remember some bits and pieces, being in a room, or someone I met, things like that.
Now, interestingly, these models might not have this limitation. You can find an article written online years ago that will contain all the images, and everything will be perfect, perfectly reconstructed. So the second mode is called contextual memory, and it is clear that when you integrate particularly powerful search engines into our models, we see this.
The third is something you could call working memory, which is actually one of the entire thinking processes I described. If we take every news article out, and then we want to create summaries, find out the relationships between them, critique some of them, this starts to combine working memory, which means I will have a draft of the summary This combines working memory, which can better facilitate reasoning when processing long and short contexts.
A breakthrough this year is the ability to handle millions of tokens in context, allowing you to retrieve something from the past and bring it into the future for very detailed analysis. For example, we can upload and summarize movies or long video content, and we can make quite a few associations within each frame, every object in the movie, and so on.
Host:
Is a longer context window always better? Because I'm just thinking, I don't know to what extent you draw inspiration from neuroscience in your work. But human working memory is limited. Of course, there are times when you feel like, my brain is full, I'm done.
Oriol Vinyals:
Sometimes the brain is an inspiration, but computers definitely have an advantage. We should leverage its strengths, so perhaps they can remember every Wikipedia article, no matter what, which we cannot do, but if the model can, then that's fine.
At the same time, even for these neural networks, too much information can be overwhelming. So compression might be a good idea. So you might want to draw some inspiration from that to do what we do, which is quite remarkable in terms of memory retrieval and so on.
Risk Warning and Disclaimer
The market has risks, and investment requires caution. This article does not constitute personal investment advice and does not take into account the specific investment goals, financial situation, or needs of individual users. Users should consider whether any opinions, views, or conclusions in this article align with their specific circumstances. Investing based on this is at your own risk