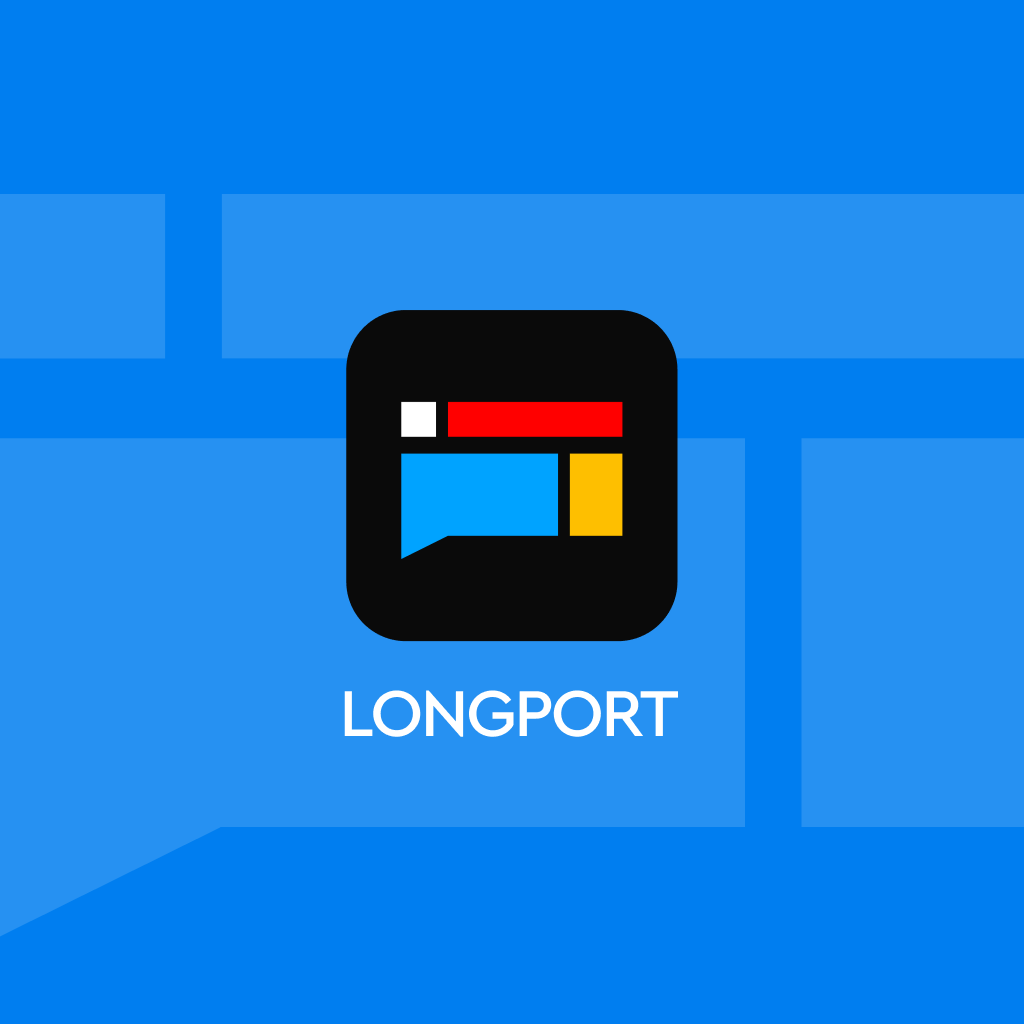
From NVIDIA to Broadcom, a "paradigm shift" in the AI industry
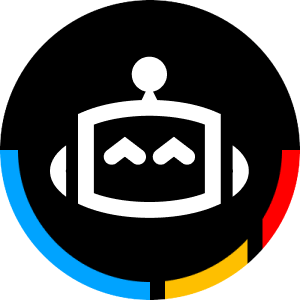
As AI large models transition from the pre-training phase to the logical reasoning phase, dedicated chips represented by ASICs may gradually replace general-purpose chips represented by GPUs, becoming the "new favorite" of major AI companies. Analysis suggests that if Broadcom's CEO's predictions about the ASIC market are accurate, Broadcom's ASIC-related AI business is expected to achieve annual growth that doubles over the next three years
Author: Li Xiaoyin
Source: Hard AI
Last Friday night, the U.S. stock market witnessed a “Buy Broadcom, Sell NVIDIA” phenomenon: Broadcom's stock price surged by 27%, setting a record for the highest single-day increase in history, with the company's market value surpassing $1 trillion, while chip leader NVIDIA's stock price recorded a 3.3% decline.
The catalyst for the buying spree came from Broadcom CEO Hock Tan's bold prediction during the earnings call that day: by 2027, the market demand for custom AI chips (ASICs) will reach between $60 billion and $90 billion.
Some analysts pointed out that if this data is realized, it means that in the next three years (2025-2027), Broadcom's AI business related to ASICs will achieve annual doubling growth, significantly enhancing market expectations for ASICs, which may indicate that ASICs are likely to enter a period of explosive growth.
Data Depletion, Diminishing Marginal Returns... Large Models Shift from Training to Inference
As the first phase of AI models, pre-training is a process of continuously “feeding” data to the model and iteratively updating it.
To enhance model performance, adhering to the principle that larger data volume, computational power, and model parameter scale are better (i.e., Scaling law), major tech giants are scrambling to acquire the most powerful NVIDIA GPUs on the market, using the accumulated GPU quantity to “guarantee” the utility of AI models.
However, intensive and large-scale model training is “draining” global databases, and while the marginal returns of model expansion are diminishing, the cost of computing power remains high, sparking discussions on whether the AI training phase is about to come to an end. Recently, Ilya Sutskever, co-founder of OpenAI and founder of SSI, stated during a speech at the NeurIPS 2024 conference that the era of pre-training is coming to an end, and data, as the fossil fuel of AI, is limited. The data currently used for AI pre-training has already reached its peak.
OpenAI expert Noam Brown also recently responded that the incredible achievements of AI from 2019 to now have come from the expansion of data and computational power, but large language models still cannot solve simple problems like tic-tac-toe.
The ensuing question is: Is Scaling All you Need? Do we really need to incur higher costs to train better AI?
The external focus is beginning to shift to the next stage of large AI models—logical reasoning.
As the next stage of large model pre-training, logical reasoning refers to the development of AI applications in various segmented vertical fields based on existing large models to achieve end deployment.
From the large model products on the market, including Google's Gemini 2.0 and OpenAI's o1, AI Agents have currently become one of the main focuses of major companies.
As large AI models mature, some believe that inference chips represented by ASICs (Application-Specific Integrated Circuits) will gradually replace training chips represented by GPUs, becoming the "new favorite" of major AI companies.
The optimistic expectations of Broadcom's CEO regarding the ASIC market somewhat confirm external expectations for a shift in the AI paradigm, leading to a surge in stock prices last Friday.
What is ASIC? More "Specialized" than GPU
Semiconductors can generally be divided into standard semiconductors and Application-Specific Integrated Circuits (ASICs). Standard semiconductors have standardized specifications and can be applied to any electronic device as long as they meet basic requirements, while ASICs are semiconductors manufactured by semiconductor manufacturers according to specific product requirements.
Therefore, ASICs are generally applied in devices designed and manufactured for specific purposes to perform necessary functions.
AI computation has thus derived two paths: one is the general path represented by NVIDIA GPUs, suitable for general high-performance computing, and the other is the specialized path represented by custom ASIC chips.
As a standard semiconductor product, GPUs perform excellently in handling large-scale parallel computing tasks, but when processing large-scale matrix multiplications, they face memory wall issues, which specially designed ASICs can solve. Once mass-produced, ASICs will offer better cost-performance ratios In simple terms, GPUs are expensive because their products and supply chains are mature, while ASICs have the advantage of being more "specialized," achieving higher processing speeds and lower energy consumption when handling single computation tasks, making them more suitable for inference and edge computing.
Custom AI Chips for Tech Giants Become Marvell and Broadcom's "Cash Cows"
Due to tight GPU supply and high prices, many tech giants have begun to join the ranks of self-developed ASIC chips for their own use.
Some believe that Google is a pioneer in AI ASIC chips, having released the first generation of TPU (ASIC) products in 2015. Other representative ASIC chips include Amazon's Tranium and Inferentia, Microsoft's Maia, Meta's MTIA, and Tesla's Dojo.
In the upstream supply chain of self-developed AI chips, Marvell and Broadcom are the two dominant manufacturers.
Marvell's rise is inseparable from the successful strategy of its new leadership. As if foreseeing the future, the company's CEO Matt Murphy has shifted the company's strategic focus to custom chips for tech giants since taking office in 2016, successfully seizing opportunities in the AI wave.
In addition to major clients Google and Microsoft, Marvell recently signed a five-year cooperation agreement with Amazon AWS to help Amazon design its own AI chips. Industry insiders believe this will boost Marvell's AI custom chip business to achieve double growth in the next fiscal year.
As Marvell's main competitor, Broadcom also has three major clients: Google, Meta, and ByteDance.
Some analysts predict that by 2027-2028, each client will reach an annual procurement scale of one million ASICs, and as the fourth and fifth largest clients also begin to rise rapidly, these tech companies' chip customization orders will bring considerable AI revenue to Broadcom in the coming years.
As AI large models enter the "second half," the real inference phase has just begun, and another fierce battle over chips is about to break out. As Broadcom's CEO Hock Tan previously predicted:
"In the future, 50% of AI Flops (computing power) will be ASICs, and even CSPs (cloud service providers) will use 100% ASICs internally."