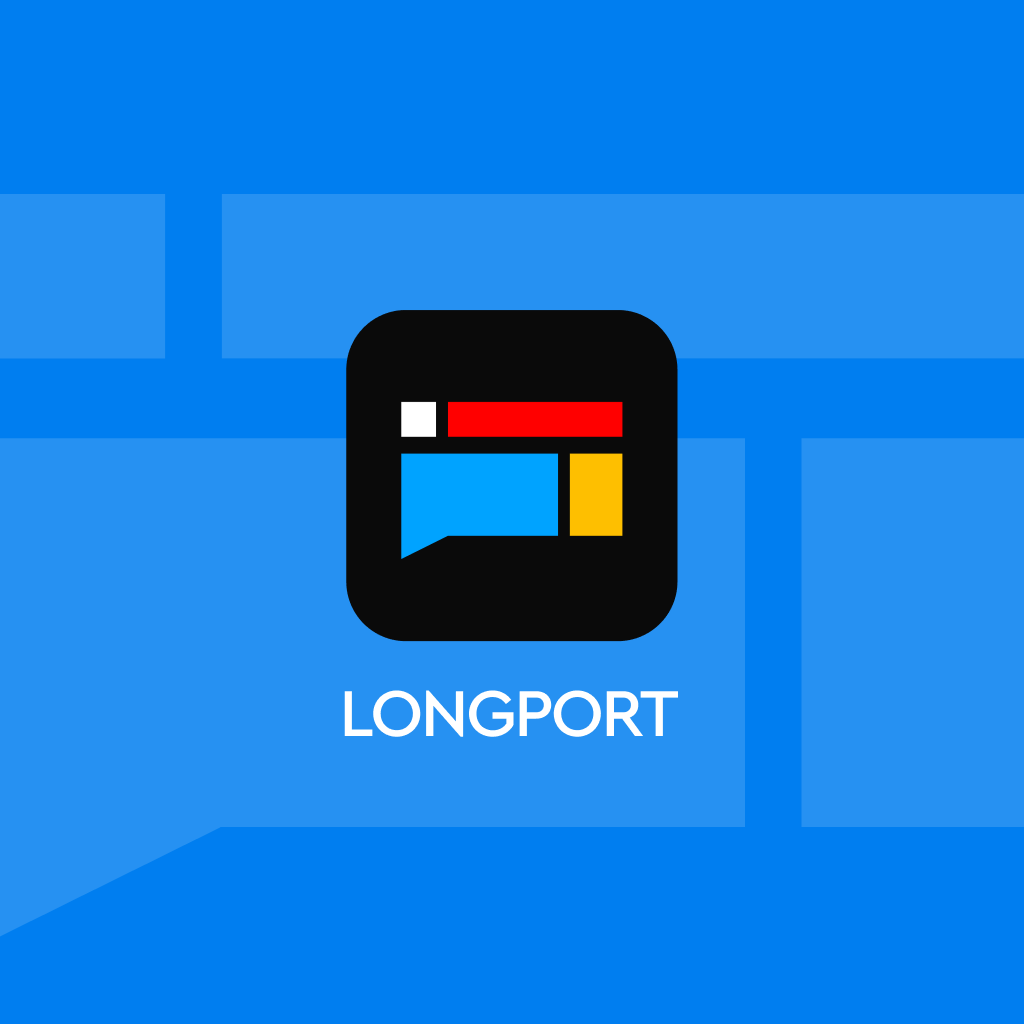
Former OpenAI Chief Scientist: What is the next step for AI?
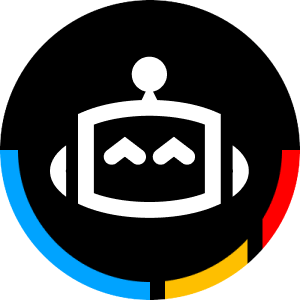
Bob McGrew believes that the progress of large models is a very slow process, for example, GPT-5 may only be ten times stronger than GPT-4, and will not be as significant as previous generations of models. He predicts that the quality of video models will see significant improvements in the future, and fully AI-generated award-winning films may appear within two years. He also believes that robotics technology will become widespread within five years and thinks that now is a good time to create robotics companies
In the field of artificial intelligence, few are as prominent as Bob McGrew. As the former Chief Research Officer of OpenAI, McGrew has been a key figure in achieving breakthroughs in AI technology over the past six and a half years. On Wednesday, more than a month after leaving OpenAI, McGrew rarely accepted an interview from the outside world.
On the 18th local time, Bob McGrew discussed the future of AI on the RedPoint AI podcast, including whether large models have hit a bottleneck, how robots and AI videos will develop in the future, and other issues.
McGrew first responded to the current debate about whether the capabilities of large models have reached their limits, stating that there is still a lot of room for improvement in large models, but this is a slow process that will take years to refine.
He pointed out that there is a significant difference between external perceptions of model capabilities and those within the lab. To the outside world, the development of large models seems to be a rapid process, but for those inside the lab, every advancement requires a huge amount of computational resources. For example, from GPT-3 to GPT-4, computational power increased by 100 times, and this growth came not only from adding more chips and data centers but also from algorithm improvements.
McGrew emphasized that advancements in pre-training require enormous computational power, which often involves building new data centers, and this is a slow process. He mentioned that the evolution from GPT-4 to GPT-5 may only see a tenfold increase in GPT's capabilities.
McGrew also predicted that the quality of video models will see significant improvements in the future, stating that fully AI-generated, award-worthy films may emerge within two years, and costs will decrease significantly.
When discussing robotics technology, McGrew expressed great enthusiasm. He believes that robotics technology will become widespread within five years and considers this a good time to create robotics companies, as foundational models have made breakthrough progress in rapid deployment and generalization.
McGrew also believes that AGI (Artificial General Intelligence) may not have a clear turning point but will be a series of gradual developments. He predicted that as AI progresses, we will enter a world where intelligence is ubiquitous and free, at which point agency will be one of the scarcest resources.
Wall Street Insights has summarized the highlights of this interview:
- From GPT-2 to GPT-3, or from GPT-3 to GPT-4, the effective computational power increased by 100 times. This is what this increment represents. You can achieve this by increasing floating-point operations, adding chips, expanding data centers, and improving algorithms. Algorithm improvements can yield some benefits—50%, 2 times, or 3 times is already impressive. But fundamentally, you have to wait for new data centers to be built.
- Fundamentally, this is a very slow process that takes years. In fact, before you see a complete generational transition, such as from GPT-4 to GPT-5, you will see something with only a tenfold improvement. People often forget that we transitioned from GPT-3 to GPT-3.5 and then to GPT-4
- I think we have to wait and see when the next generation model is released. If you look at something like O1, we have already been able to make progress using reinforcement learning. By various metrics, O1 represents a computational capacity that is 100 times greater than GPT-4. Some people may not realize this because it was decided to name it O1 instead of GPT-5. However, in reality, this is a next-generation model.
- So if I consider the difference between today's video models and video models two years from now, the first thing is that the quality will be better. The instantaneous quality right now is already very good. On the other hand, another analogy is that I actually think it will be very much like large language models; if you want a token of GPT-3 quality, it will be 100 times cheaper than when GPT-3 first came out. The same will be true for Sora; you will be able to see these very beautiful, realistic videos, and their cost will be almost zero.
- (AI-generated movies) winning an award is somewhat too low a threshold, right? I think there will be many award ceremonies... I feel we will see it in two years, but it will actually be less impressive than what I just said because the reason you want to watch it is not because of the video itself, but because there is a director with a creative vision who uses the video model to realize his creative vision.
- I believe robotics will be widely adopted in five years, although there will be some limitations. Therefore, I think now is a good time to start a robotics company. I won't predict when robots will enter households, but I think you will see them being widely applied.
- I find it hard to understand the concept of AGI. And I think, if anything, I have a deep critique of AGI, which is that there is no clear turning point; in fact, these issues are fractal. And we will see more and more things being automated. But somehow, we—I don’t know. I have a feeling it will become very mundane, and somehow we will all be driving autonomous cars to the office, where we will command an army of AIs. Then we will feel, oh, this is a bit boring. It still feels like being in the office, and my boss is still an idiot. That’s probably the future of our AGI.
- We are transitioning from an era where intelligence may be the most scarce resource in society to an era where intelligence will be ubiquitous and free. So what are the scarce factors of production? I guess it’s agency. What right questions do you need to ask? What right projects do you need to pursue? I think these types of questions are difficult for AI to solve for us. I think these will be the core issues that humanity needs to figure out.
Here is the full transcript of this interview (translated by AI):
Host Jacob:
Bob McGrew served as Chief Research Officer at OpenAI for six and a half years. He recently left a few months ago, and we are fortunate to be one of the first podcasts he has appeared on since then on the "Unsupervised Learning" podcast. Therefore, we have the opportunity to ask him everything about the future of artificial intelligence We discussed whether the model has reached a bottleneck, as well as robot models, video models, computer usage models, and the future timeline and capabilities that Bob envisions. We talked about OpenAI's unique culture and what makes its research so effective, along with some key decision points and the feelings associated with making those decisions. We explored why AGI might feel no different from today, and Bob shared his reasons for leaving OpenAI and his next steps. I think everyone will really enjoy this episode. Without further ado, here’s Bob. Bob, thank you so much for joining the podcast. Thank you for the invitation, I’m looking forward to this conversation. I’m really glad you could come. I know we’ll cover a lot of different topics. I think we might as well start with what I believe is the most pressing question on everyone’s mind right now, which is the heated debate about whether the model's capabilities have reached a bottleneck. We’d love to hear your thoughts on this and how much potential you think there is left to tap in pre-training.
Bob McGrew: Okay, I think this is probably the area where there is the biggest divergence between external observers and those inside large labs. I think from the outside, many people initially started paying attention to AI because of ChatGPT. Then six months later, GPT-4 came out. It felt like everything was accelerating quickly and making progress. However, GPT-4 was released a year and a half ago, and everyone knows it was trained before that. So, what’s happening now? Why hasn’t anything new emerged, right?
The internal perspective is quite different. On the outside, people want to know if we’ve hit a data bottleneck. What exactly is happening? But you have to remember that to make progress in pre-training, especially, you need to significantly increase the amount of computation. The effective computation increased by 100 times from GPT-2 to GPT-3, or from GPT-3 to GPT-4. That’s what this increment represents. You can achieve this by increasing floating-point operations, adding chips, expanding data centers, and improving algorithms. Algorithm improvements can yield some gains—50%, 2 times, or 3 times would already be impressive. But fundamentally, you have to wait for new data centers to be built.
There are indeed many new data centers under construction. Just look at the news, and you’ll find that companies like Meta, X, and other cutting-edge labs are also building new data centers, even if these stories don’t always make headlines. But fundamentally, this is a very slow process that takes years. In fact, before you see a complete generational transition, like from GPT-4 to GPT-5, you will see something with only a 10 times improvement. People often forget that we went from GPT-3 to GPT-3.5 and then to GPT-4.
What’s interesting now is that pre-training is ongoing. I think we have to wait and see when the next generation of models will be released. If you look at something like O1, we’ve already been able to make progress using reinforcement learning From various indicators, O1 represents a computational capacity 100 times greater than GPT-4. Some people may not realize this, as it was decided to name it O1 instead of GPT-5. However, in reality, this is a next-generation model.
When the next generation, the hypothetical GPT-4.5, is trained, an interesting question is how this pre-training progress compares to the reinforcement learning process. I think we can only wait and see what news will be released.
Host Jordan: This raises a question, considering the multi-year process leading into 2025, do you think the progress in artificial intelligence next year will be as much as last year, or do you think things will start to slow down?
Bob McGrew: Well, I think there will be progress. I think it will be different progress. One thing is that when you enter any next generation, you always encounter problems that were not seen in the previous generation. So even if the data centers are built, people need time to solve problems and complete the training of the model.
The reinforcement learning process we used to train O1, which is the process OpenAI used to train O1, created a longer and more coherent chain of thought, effectively integrating more computational capacity into the answers. So, you know, if one model takes a few seconds to generate an answer, and another model takes, say, several hours to generate an answer, then if you can really leverage it, that’s 10,000 times the computational capacity, right?
To be honest, we started thinking about how to use test-time computation around 2020. Ultimately, I think this is actually the real answer to how to do it, that is, how to do it without wasting a lot of computational resources. The benefit of doing this is that it does not require new data centers. There is a lot of room for improvement here because this is a new technology that has just begun, and there are many opportunities for algorithmic enhancement.
Theoretically, there is no reason why the same basic principles and ideas used to make O1 think for 30 seconds, 1 minute, or several minutes for tasks that GPT-4 can complete in a few seconds cannot be scaled to several hours or even days. Just like from GPT-3 to GPT-4, there was no foundational new technology; both were trained in roughly the same way, but scaling is very difficult.
So this is actually the core of the question: can you really scale? I think this will be the type of progress we will see, and it will be the most exciting.
Host Jacob: Yes, in 2025. Considering the focus on test-time computation and the current use of O1, I think it’s really interesting to think about how people will actually use these models, right? I think you recently tweeted something that I found very interesting, about needing these new form factors to unlock certain capabilities of the models. So maybe you could elaborate a bit on that. For example, have you seen any early form factors that you think are interesting when using these models?
Bob McGrew: Well, yes. To explain this issue, chatbots have been around for a while. Most of the interactions people have with chatbots today can be well handled by models at the GPT-4 level. You know, if you ask ChatGPT, who was the fourth Roman emperor? Or how do I heat Indian basmati rice? Most of our daily conversations can be handled well.
When we consider releasing the O1 preview, there are many questions about whether people will use it and whether they will find something to do with it. I think these questions are valid. It relates to understanding what needs to be done with this model to truly derive value from it. Programming is a great use case in this regard because it presents a structured problem where you are trying to make progress over a long period and it significantly leverages reasoning capabilities.
Another example is if you are writing a policy brief. In this case, you need to write a lengthy document that needs to be meaningful and cohesive. The fact is, while there are many programmers, most non-programmers do not need to solve such tasks on a daily basis. However, returning to the potential breakthroughs here, it is important to have a coherent chain of thought and a structured approach to problem-solving.
This process involves not just thinking about the problem; it can also include taking action and formulating an action plan. What excites me most about models like O1 — and I believe other labs will soon release similar models — is using them to achieve long-term actions, essentially acting as agents. While I think the term "agent" is overused and does not clearly convey the goals we are trying to achieve, in my life, I have many tasks where I wish the model could book things for me, shop for me, and solve problems in ways that involve interacting with other parts of the world.
I think this is the product form we really need to solve: understanding what it is and how we can deploy it effectively. As of now, I don't think anyone has figured that out yet.
Host Jacob: That's fascinating. I mean, that makes complete sense. I feel like everyone, you know, has endless imagination about what these agents can do and what problems they can solve for people and businesses. So, what is the biggest barrier to achieving all of this today? Obviously, you've seen some early models, like the computer usage model released by Anthropic, and I'm sure other labs are working on this as well. But when you think about what is holding us back from reaching our goals, what challenges still need to be solved?
Bob McGrew: Yes, there are many issues. I think the most immediate issue is reliability. So, you know, if I ask it to do something, setting aside the action for a moment, right? If I ask the agent to do something on my behalf, even if it's just to think or write some code for me, and I need to leave for five minutes or an hour to let it work, if it deviates from the task and makes a mistake, by the time I come back, it has done nothing, then I've just wasted an hour This is a big problem.
Now, adding to this is the fact that the intelligent agent will execute actions in the real world. Maybe it's buying something for me. Maybe it's submitting a press release. Maybe it's sending notes, emails, or Slack messages on my behalf. If it doesn't do well, there will be consequences. I will at least feel embarrassed, and I might even lose some money. Therefore, reliability becomes even more important than before.
I think there is a rule of thumb when considering reliability: increasing from 90% reliability to 99% reliability may increase the computational load by an order of magnitude. That's a tenfold improvement. To increase from 99% reliability to 99.9% reliability requires another order of magnitude increase. Therefore, each additional "9" demands a huge leap in model performance. This tenfold improvement is significant and represents a year or two of work.
So I think this is the first problem we need to face. I think the second interesting question is that everything we've talked about so far has been consumer-focused, right? You haven't embedded it into enterprises. However, when you're talking about agents executing tasks, for many of us, that will be what we do at work, which is embedded in enterprises. I think this will bring a whole series of other considerations.
Host Jordan: That's interesting. We see today in enterprises that many consulting firms are actually doing quite well because deploying these technologies to enterprises currently requires a lot of hands-on guidance. Do you think this hands-on guidance and the demand for help from enterprises will last for a while? Or do you think it will become easier to use, and enterprises will be able to deploy these large language models very easily in the future?
Bob McGrew: Yes, I think that's a very interesting question. And I mean, even starting to build, what is the problem of deploying large language models in enterprises? Well, if it's going to automate a task for you or do your job, it might need context. Because in the consumer space, there isn't much context. Well, you like red, great. Not much to it.
Host Jacob: Thank you for using red as an example (his podcast is called RedPoint).
Bob McGrew: But, you know, in enterprises, you know, who your colleagues are? What projects are you working on? What is your codebase? You know, what have people tried? What do people like and dislike? All this information exists in enterprises in an environmental way. It's in your Slack. It's in your documents. You know, maybe it's in your Figma or somewhere else. So how do you gain access?
Well, you need to build some one-off things yourself. I think there is definitely a way for people to build libraries of these connectors, and then you can come in and do that. This is very similar to the work we did at Palantir, where the fundamental problem Palantir solved was integrating data within enterprises I think this is also one of the reasons why Palantir's artificial intelligence platform AIP is so interesting. So I think this is the first path, where you are somewhat building a library of these things. You can build an entire platform based on this.
Another opportunity is the use of computer use. So now, you no longer need this very specific and possibly customized way to do things; you now have a tool that can handle everything. Anthropic has launched this; it's really interesting, and we were already discussing these computer use agents with the people at Anthropic before they left OpenAI in 2020, and Google DeepMind has also published papers on this topic. Every lab has considered this issue and is committed to solving it.
The difference between computer use agents and these programmatic API integrations is that now, since you control the mouse and keyboard, the actions you take involve more steps. You might need 10 times or even 100 times the number of tokens required for these programmatic integrations.
So now, we are back to what? You need a model with a very long and coherent chain of thought that can consistently solve problems over a long period of time, which is exactly the kind of problem O1 addresses. I believe there are other ways to solve this issue. But I think this will be a breakthrough we will see in the coming years.
Host Jacob: Next year. How do you think it will ultimately develop? Because on one hand, obviously, a general model that can be used in any context seems very appealing. I think achieving 99.999% reliability might be difficult. And, you know, there could be many steps that go wrong at different points. You know, another perspective on how this works is that I'm sure if the underlying application APIs were somehow opened up, some of these issues might be simplified, right? Or other methods, or you could provide specific models for using Salesforce or some specific tools I don't know about. If you could access the underlying experience, then integration would ultimately become a huge advantage. This way, you could get things done in an instant instead of sitting there watching the computer do things on the screen.
Bob McGrew: Yes, well, I mean, I think you will definitely see a mix of these approaches, some using these integrations, while others, you know, computer use becomes a fallback option if you don't have something customized to use. Then maybe you will look at what people are using, and if feasible, you will propose more detailed integrations.
I think regarding the question of whether you will see Salesforce-specific computer use agents, technically, that doesn't make much sense to me because I think you are fundamentally leveraging data. Someone has gone out and collected a large dataset on how to use Salesforce
You can throw this data into—sharing these datasets with Anthropic, OpenAI, and Google is beneficial for Salesforce. They train their own models. I think every application provider would want this to be public and part of every foundational model. So I don't think, you know, to me, it seems there’s no reason to have specialized models in this way.
Host Jacob: No, that is indeed a compelling point because I feel that when you are in a competitive field, and your competitors are making their data public and their products are becoming easier to use, you certainly want your product to be like that too.
Bob McGrew: Yes, it’s a bit mysterious to me why that ecosystem of people stuffing data into large language models hasn’t emerged yet. It’s actually quite similar to Google’s SEO.
Host Jacob: That’s a really interesting point. How far do you think we are from widespread applications of computer usage?
Bob McGrew: Well, I mean, I think there’s a good rule of thumb for these things, which is when you see a demo that’s super attractive but not quite usable yet. It’s painful to use. Then, you know, give it a year, and it will be ten times better. And this improvement grows logarithmically. So ten times better, you know, is just one level of improvement. But one level of improvement is already quite remarkable. You’ll start to see it used in limited use cases. Then give it a second year. By then, it will be surprisingly effective, but you can’t rely on it every time. That’s how we use chatbots now; you still have to worry about them generating hallucinations. So, the adoption issue actually depends on the level of reliability you require. Any field that can tolerate errors will automate faster than those that cannot tolerate errors.
Host Jacob: So I want to go back to Jordan’s initial question, basically, now you need a lot of assistance to integrate the right data and define customized safeguards and workflows, which makes complete sense. So, what kind of middle layer will exist between “Hey, great computer usage model, businesses are ready to sign up”? What will this middle layer look like?
Bob McGrew: Man, I think there should be startups to define it. You know, I think we don’t fully know the answer yet. I think when you have a general tool like computer usage, you’ll see an interesting phenomenon where the problems it solves are fractal in difficulty; it can solve many problems. But then you’ll see a really important problem that you can’t fully solve. Then you’ll say, okay, now we need to do something very specific for this, and maybe we’ll take a programmatic approach for that. So I think we’ll see a mix of various approaches for a while
Host Jordan: I'm very curious, you have clearly been working in research and are responsible for some truly cutting-edge research. We briefly talked about inference time computation. What other areas are you particularly interested in?
Bob McGrew: Well, I think we've talked about pre-training. We've talked about inference time computation. Another really exciting thing is multimodal. Multimodal is an important day. Yes, Sora was released today. In fact, this is in some ways the culmination of this long journey. Large language models, which we assume were invented in 2018. Clearly, you can apply Transformers and some of the same techniques to adapt to other modalities. So you include vision, with image output, audio input, and audio output.
Initially, these things were auxiliary models like DALLE or Whisper. Eventually, they were integrated into the main model. The modality that has long resisted this practice is video. I think Sora is the first to demonstrate this; other companies, like Runway, and some other models have also emerged in succession. Now that Sora itself has been released. I think video has two really interesting and different aspects compared to other modalities.
When you create an image, you might really just want to create an image with a single prompt. Maybe you try a few times. If you're a professional graphic designer, you might edit some details in that image. But to be honest, none of us are. A lot of the use cases here are, do you need some slides? Do you want an image to accompany your tweet or presentation? It's a very straightforward process.
However, for video, wow. I mean, this is a series of extended events. It's not a prompt. So now you actually need a complete user interface. You need to think about how to unfold this story over time. I think that's one of the things we see in the Sora release. Sora has spent more time thinking about this; the product team has invested more effort in this than some other platforms.
Another thing you need to consider is that the cost of video is very high. Training these models is very expensive, and running these models is also very costly. So, while it's interesting to see quality video from Sora—and I think the quality of Sora is indeed better—you have to pay a little attention to see that its quality is better, at least if you're only looking at a brief clip.
Now, anyone with a Plus account can use Sora. OpenAI has released a Pro account for $200 a month, which includes unlimited slow generation of Sora. When you have this level of quality and distribution, two puzzles have been solved. This will be a high barrier that other competitors will find difficult to reach.
Host Jacob: What will the development of video models look like in the coming years? I mean, clearly, we've seen tremendous progress in the field of large language models, and it feels like the models from last year are now ten times cheaper and much faster Do you think there will be similar improvements in video as well?
Bob McGrew: Actually, I think the analogy is very direct. So if I consider the differences between today's video models and video models two years from now, the first thing is that the quality will be better. The instantaneous quality is already very good. You can see reflections. If you share something, all the difficult problems to solve, you can point out, oh, look, there was a reflection done there. There’s some smoke. You know, the hard part is the expansive, coherent generation.
So the SOAR product team has a storyboard feature that allows you to set checkpoints at different time points, like every five seconds or every ten seconds, to help guide the generation. You know, fundamentally, if you want to go from a few seconds of video to an hour of video, that’s a very difficult problem. I think that’s something you’ll see in the next generation of models.
On the other hand, another analogy is that I actually think it will be very much like large language models, where if you want a GPT-3 quality token, it’s 100 times cheaper than when GPT-3 first came out. The same will be true for Sora; you will be able to see these very beautiful, realistic videos, and their cost will be almost zero.
Host Jacob: I feel like the dream is to have a complete movie generated by AI that wins some awards or something, you know, asking with a shameless podcast question, when do you think we will have such a movie?
Bob McGrew: I can only guess. Oh my gosh. Yes. To be honest, winning an award is somewhat of a low bar, right? I think there are a lot of award ceremonies. Really, is it a movie you actually want to watch? Yes. I think we will see it in two years, but it will actually be less impressive than what I just said because the reason you want to watch it is not because of the video itself, but because there is a director with a creative vision using the video model to realize his creative vision. I think they do this because they can do things in this medium that they couldn’t film. We can imagine. None of us here are directors, but we can all imagine a lot of possibilities. We are not graphic designers or directors, but yes, that will be the future.
Host Jordan: Exactly. Yes, we have some very specific skills here. Yes, we see a lot of companies emerging trying to be the Pixar of AI. We always ask the question, when is this really feasible? So it sounds like it’s much faster than we at least anticipated.
Bob McGrew: That’s my guess. Once things progress to a demonstrable stage, the subsequent progress will be very fast. Before that, progress is very slow, or at least it is invisible.
Host Jordan: I want to shift from video to robotics; you initially joined OpenAI to research a lot of robotics. We’d love to hear your thoughts on this field, where we are today, and where you think it will go
Bob McGrew: This is indeed a very personal question. When I left Palantir, one of my thoughts was that robotics would become the realm where deep learning becomes real, rather than just a button on someone's website. So, I spent a year between Palantir and OpenAI delving into robotics, writing some early code on vision with deep learning. This is a very challenging field. At the time, I thought it might take another five years; that was in 2015, and that was completely wrong. However, I think now is the right time. I believe robotics will be widely applied in five years, although there will be some limitations. Therefore, I think now is a good time to start a robotics company.
A fairly obvious point is that foundational models have made significant breakthroughs in quickly starting and running robots, enabling them to generalize in important ways. There are several different aspects to this. One of the more obvious ones is the ability to utilize vision and translate that vision into action plans, which is brought about by foundational models. A slightly less obvious, perhaps more interesting aspect is that the entire ecosystem has developed. Now that I have left OpenAI, I have spent some time with founders, and I have talked to some robotics founders. One robotics founder told me that they have actually set up their robots to be able to converse. This is really cool and much easier; you can tell the robot what to do, and it understands the gist. It uses some specialized models to perform actions. Previously, writing out what you wanted was cumbersome; you had to sit in front of a computer instead of looking at the robot. Now you just need to talk to it.
I think one of the main distinctions we still don't understand about the outcomes is whether you are learning in simulation or in the real world. Our main contribution in the robotics field over the past two years has been to demonstrate that you can train in a simulator and generalize it to the real world. There are many reasons to use simulators; for example, running in production systems or the real world is quite troublesome. You can conduct free testing, etc. However, simulators are good at simulating rigid bodies. If you are doing grasping and placing tasks with hard objects, that’s great. But a lot of things in the world are soft, squishy objects. You have to deal with fabrics, or, when considering warehouses, deal with cardboard. Unfortunately, simulators do not perform particularly well in handling these scenarios. Therefore, for anyone wanting something truly general, our only method now is to use demonstrations in the real world. As you can see from some of the recent work that has emerged, this can actually yield promising results.
Host Jacob: The results are very good. Then, I think, obviously this is somewhat unknowable, like, you know, when people discover scaling laws in robotics, and how much remote operation data people might need, but do you think we are close to it? Or, I mean, obviously, you know, back in 2015, you thought there were still five years to go. How far do you think we are from the moment when people say robotics is like ChatGPT, and they will say, oh, that’s really great, that looks very different and effective
Bob McGrew: When it comes to predictions, especially regarding robotics, you really have to consider this field. So I hold a rather pessimistic view on the large-scale consumer adoption of robotics because having a robot at home is quite scary. Robotic arms can be lethal. They could kill you, and more importantly, they could kill your children. And, you know, you can use different kinds of robotic arms that don't have these drawbacks, but they have other drawbacks. A home is a very unconstrained environment.
But I do think that in various forms of retail or other work environments, I think we will see this in five years. If you go to an Amazon warehouse, you can even see this; they already have robots that solve their mobility issues. You know, they are working on picking and placing. I think you will see a large rollout of robots in warehouse environments.
Then, you know, it will gradually advance by domain over time. I won't predict when it will enter homes, but I think you will see it widely applied. I think in five years, we will interact with them in ways that would feel strange today in our daily lives.
Host Jacob: I mean, there are clearly some independent robotics companies already. To some extent, it’s clear that robotics leverages the advancements in foundational technologies, you know, LLMs. I'm curious, for example, whether all of this will converge? Clearly, some companies are only doing video models. Some companies focus on biology, materials science. When you think about its long-term development direction, you know, will there be a massive model that covers all of these?
Bob McGrew: At the cutting edge of model scale, I think you should continue to expect these companies to roll out a model. It will do the best on every dimension of the data they have. This is an important caveat.
What specialization really brings you is cost-effectiveness. Over the past year, you have seen cutting-edge labs do better with a large number of smart small models that can perform chatbot-like use cases at a very low cost.
If you are a company, at this point, a very common pattern is that you figure out what you want AI to do for you, and then you use the cutting-edge model you like to run it. Then, you generate a massive database and fine-tune some smaller models to perform that task. You know, this is a very common practice; OpenAI offers this service, and I believe this is a common pattern across every platform.
You could say, you know, this is very, very cheap. Now, if you trained a chatbot like this, your customer service chatbot is trained this way, if someone deviates from the script, it won't be as good as when you originally used the cutting-edge model. But that's okay; it's the cost-effectiveness that people are willing to accept
Host Jacob: There's something I find very interesting. When we were chatting earlier, you mentioned a macro perspective on the progress of artificial intelligence, basically saying that back in 2018, we expected that by 2024, we would have various model capabilities. You would argue from first principles that these things have fundamentally changed. It's like the world is almost unrecognizable compared to 2018. While you have indeed had a huge impact on the broader world, I can't yet say that the proliferation of artificial intelligence has completely changed the way the world operates. Why do you think that is?
Bob McGrew: Well, I just want to slightly rephrase that. I think, although it sounds strange, the correct mindset about artificial intelligence should be deeply pessimistic. For example, why is progress so slow? Why, you know, some say that artificial intelligence has led to a 0.1% increase in GDP. But that's not due to productivity gains from using artificial intelligence; it's because of the capital expenditures incurred in building the data centers needed to train artificial intelligence. So, why isn't artificial intelligence more apparent in productivity statistics? It's like what people said about the internet in the 1990s.
I think there are several reasons for this. First, the viewpoint from 2018 assumed that once you could converse with it and it could write code, everyone would immediately achieve automation. This is akin to the idea when engineers are asked to write a feature. You might think, "Oh, yes, I can get this done in a few weeks." But when you start writing code, you realize, "Oh, actually, this feature is much more complex than I imagined." If you're a good engineer, you might estimate two weeks, but the project might actually take two months. If you're a bad engineer, you might find that the feature can't be written at all.
I think this is what happens when we really dive into how humans accomplish work. Yes, you might talk to them on the phone, but that doesn't mean what they do is just talking to you. There is real work involved. Fundamentally, artificial intelligence can only automate a single task. However, a job consists of many tasks. When you closely examine real work, you find that for most jobs, there are some tasks that cannot be automated.
Even if you look at programming, for example, boilerplate code is optimized first, and those trickier parts, like figuring out exactly what you want to do, are solved last. So I think as we continue to promote artificial intelligence, we will find increasing complexity and limitations in automating the full range of human work.
Host Jordan: Given that, in terms of progress, what areas do you think are currently underestimated and should receive more attention than they do now?
Bob McGrew: Well, okay. Here's an answer: I'm really interested in startups that are using artificial intelligence to solve some very tedious problems
Imagine you run a company, and you can hire all the smart people you want to do some super boring things, like checking all your expenses and ensuring you did proper price comparisons. For example, if your procurement department consisted of people like Elon Musk, who are really meticulous about controlling expenses, you could save a lot of money.
No one does this because, you know, those who can really save money would find it boring. They would hate the job, right? But artificial intelligence is infinitely patient.
It doesn't have to be infinitely smart. And, you know, I think anywhere you run your business, if you can derive value from what those infinitely patient people do, then that's what AI should automate.
Host Jacob: That's interesting because I've always thought of consultants as a way to get smart people to solve boring problems or work in boring industries. And obviously, with cutting-edge AI models, you can have a highly intelligent person solve problems that you could never get a smart person to do.
Bob McGrew: Yes, I mean, the first time I heard someone do productivity research that showed AI actually brought a 20% to 50% improvement, I thought, wow, that's amazing. Then I found out, oh, it's consultants. Well, you know, AI is very good at "bullshitting," and that's what consultants do. So maybe we shouldn't be surprised that productivity improvements show up here first.
Host Jacob: Yes, I think the improvement is also the greatest among the lower-performing half, right?
Bob McGrew: Exactly. Well, actually, I think that's a bit hopeful. Because if you look at the lower-performing half, you know, they have skills that are hard to automate, which is the hopeful version of this. They know what they're doing, but they don't know how to code it. Then the model comes along and says, oh, I know how to code it, but I don't know what I should be doing. So now these lower performers can actually get real boosts in their work. So I find that very hopeful.
Host Jordan: I think, in terms of performance, you've worked and are working with some of the best researchers in the world. What do you think makes an AI researcher the best?
Bob McGrew: There are many different types of researchers doing different things. If you think of someone like Alec Radford, who invented the GPT series and CLIP, you'll find he basically invented large language models (LLMs) and then continued with various forms of multimodal research. Alec is someone who likes to work alone at strange hours late at night. In contrast, others like Ilya Sutskever and Jacob Pichai, who are the first and second chief scientists at OpenAI, respectively, have great ideas and visions They help others solve challenges and play a key role in developing the overall roadmap for the company.
The best scientists share a common key trait: perseverance. I will always remember watching Aditya Ramesh, who invented DALL-E, working hard to solve the problem of generating an image that was not in the training set to prove that neural networks have creativity. The original idea for DALL-E was to see if it could create an image of a pink panda ice skating, and Aditya was convinced that this image did not exist in the training data. He worked on this for 18 months, maybe two years, trying to achieve this goal.
I remember about a year later, Ilya came over to show me a picture and said, "Look, this is the latest generation. It's really starting to work." What I saw was a blur, with pink faintly visible at the top and white at the bottom—just pixels starting to come together. I couldn't see much at the time, but Aditya was persistent. This kind of tenacity is something every successful researcher must have when tackling fundamental problems. They must see it as their "final battle" and be determined to stick with it for years if necessary.
Host Jacob: What have you learned from building a research institution with such a group of people to make it work?
Bob McGrew: Well, interestingly, the best analogy I can think of actually comes from Alex Carp at Palantir, who always says that engineers are artists. That makes a lot of sense. When you talk to a truly great engineer, they just want to create. There’s something in their mind. Code is the way they turn the sculpture in their mind into reality.
At Palantir, you know, you have to get them to fix bugs, but every time you do that, their artistic side feels sad. You have to have a process that allows people to work together, but their artistic side will feel sad. The fact is, engineers are artists, a 10x engineer is a 10x artist, and researchers are 100x artists compared to any engineer.
Building an organization with researchers requires much more consideration. There’s a way of engineering management where you would say if everyone is interchangeable parts and you have a process that allows them to work together, that’s great. However, working with researchers requires very close attention because the most critical thing is that you cannot stifle their artistry.
It is their passion for the vision in their minds that makes them willing to endure all the challenges of turning that vision into reality.
Host Jordan: You are fortunate to have worked at both Palantir and OpenAI, and there are many articles discussing how special Palantir's culture is. When you think of OpenAI, I believe there will also be many articles about its culture in the future. What do you think those articles will say?
Bob McGrew: Yes. I mean, I think one point is working with researchers, as we just talked about. Another crazy thing about OpenAI is how many transformations it has gone through, or I prefer to think of it as multiple rebuilds. So when I joined OpenAI, it was a nonprofit organization. The vision of the company was to build AGI by writing papers. We knew that was wrong; it didn't feel quite right. Many of the early people, Sam, Greg, and I, were entrepreneurs, and the path to AGI felt off.
A few years later, the company transformed from a nonprofit to a for-profit organization. This caused a lot of controversy internally, partly because we knew that at some point we would have to interact with products. We had to think about how to make money. The partnership with Microsoft became another rebuilding moment, which also sparked a lot of controversy. I mean, maybe making money is one thing, but giving it to Microsoft, to big tech companies, wow, that's terrible.
Additionally, it was equally important that we decided to say, okay, not only are we going to partner with Microsoft, but we are also going to build our own products using the API. Ultimately, adding consumer services to enterprise services through ChatGPT. These are all decisive transformations that startups go through. At OpenAI, it feels like every 18 months or two years, we are fundamentally changing the purpose of the company and the identity of the people working there.
We shifted from the concept of writing papers as your job to the idea of building a model that everyone in the world can use. The really crazy thing is that if you had asked us in 2017 what the right mission was, it wouldn't have been to achieve AGI through writing papers; instead, it would have been that we wanted to build a model that everyone could use. But we didn't know how to achieve that, so we could only explore and figure all these things out along the way.
Host Jacob: What do you think made you so successful in making these major transformations?
Bob McGrew: Well, I mean, first is necessity. These weren't arbitrary choices, right? You have a nonprofit organization, you run out of money, and maybe you need to find a way to raise funds; perhaps to raise funds, you have to become a for-profit company. Your partnership with Microsoft, maybe they didn't see the value of the model you were creating, so you needed to build an API because it might actually work. Then you could show them that people actually want these models.
ChatGPT, I think that's when we really believed after GPT-3, that with the right advancements, the right form, it wasn't just an API that people had to go through intermediaries to talk to the model, but that the model would be something you could talk to directly. So that's something I think is very intentional. But as we know, the way it happened was accidental. We were working on it. We had actually trained GPT-4, and we hoped to release it when the model was good enough that we could use it every day
In November, we looked at ChatGPT and wondered, has it passed the threshold? Not quite. One of the co-founders leading the team, John Schulman, said, "Listen, I really just want to release it. I want to get some external experience." I remember thinking at the time that if a thousand people used it, that would be a success. You know, our standards for success were quite low. We made a decision not to put it on a waiting list.
Then, you know, the world forced our hand again, and suddenly everyone in the world wanted to use it. What was it like in the first few days after you released it? Oh my gosh, it was very tense. At first, people were a bit skeptical that this was really happening. There was some anxiety. We quickly tried to figure out how to get GPUs. So we temporarily shifted some research computing resources over there.
Then the question arose, when will it stop? Will this continue or will it become a fad? Because we had a similar experience with DALL-E. The DALL-E 2 model caused a sensation on the internet and then just disappeared. So people were worried that ChatGPT would actually disappear too. This is where I firmly believe it won't disappear; it will actually be more important than the API.
Host Jacob: I mean, what an interesting experience. I think one of the cool things is that you are very close to cutting-edge AI research. I'm curious, what ideas have you changed in the field of AI over the past year?
Bob McGrew: Interestingly, I don't think I've changed any ideas. After GPT-3, entering 2020 and 2021, if you were in it, a lot of what needed to happen in the next four or five years felt like a given. We would have these models. We would make the models larger, and they would become multimodal. Even in 2021, we were talking about how we needed to use RL on language models and trying to figure out how to make it work. And the real difference between 2021 and 2024 isn't about what needs to happen, but the fact that we are able to make it happen. And, you know, we, the whole field, are able to make it happen. But in a way, our current situation also feels a bit fated.
Host Jacob: I guess, looking ahead, when you think about scaling pre-training and scaling testing in terms of computation, does it feel like it's also fated to achieve AGI (Artificial General Intelligence) just based on those two? Or how do you view this issue?
Bob McGrew: I find it hard to grasp the concept of AGI. And I think if there is anything, I have a deep critique of AGI, which is that there isn't a clear turning point; in fact, these issues are fractal. And we will see more and more things being automated. But somehow, we—I don't know. I have a feeling it will become very mundane, and somehow we will all be driving autonomous cars to the office, where we will command an army of AIs Then we might feel, oh, this is a bit boring. It still feels like being in the office, and my boss is still an idiot. This is probably the future of our AGI. We can't wait for 5 PM to get off work or something like that.**
More seriously, I have always felt, and I think this is a common view within OpenAI and other leading labs, that solving reasoning is the last fundamental challenge needed to scale to human-level intelligence. You need to solve pre-training, you need to solve failure modes, you need to solve reasoning. At this point, the remaining challenge is scaling. But that is very important.
Scaling is very difficult. In fact, there aren't many foundational ideas at all. Almost all the work is about how to scale them to accept increasingly large amounts of computation. This is a systems problem. This is a hardware problem. This is an optimization problem. This is a data problem. This is a pre-training problem. All the problems are actually just about scaling. So yes, I think in some sense, it is already destined. The work here is to scale it, but that is hard. A lot of work.
Host Jacob: Obviously, I think people are talking about the societal impacts of these models scaling their capabilities. I think we are still in the early stages of this discussion, and there may be many different conversations that need to happen. But what aspects are you particularly interested in and passionate about, and what do you think we should be discussing?
Bob McGrew: Yes. I think the most interesting thing is that we are transitioning from an era where intelligence may be the most scarce resource in society to an era where intelligence will be ubiquitous and free. So what are the scarce factors of production? And I think we don't know. I guess it is agency. That is, you can go and get things done. What right questions do you need to ask? What right projects do you need to pursue? I think these types of questions are hard for AI to solve for us. I think these will be the core issues that humanity needs to figure out. And not everyone is good at this. So I think what we need to think about is how we develop that kind of agency that allows us to collaborate with it.
Host Jordan: Do you think this is now, or in the future?
Bob McGrew: I think it will feel very continuous. It is an exponential curve. And the characteristic of exponential curves is that they have no memory. You always feel like you are moving forward at the same speed, at the same pace.
Host Jacob: These models ultimately won't figure it out either, I mean, if you think about figuring out what to do or project goals, you just mentioned that a few times? For example, you can imagine, at the most basic level in the future, telling the model, hey, build a good company, or create an interesting piece of art, or make a movie, and so on. As these models become more powerful, this agency, I think, maybe we can talk about that
Bob McGrew: Yes, I mean, can you directly ask artificial intelligence to solve all problems? Well, I think you can, and you will get some results. But let's take Sora as an example. If you are making a video and you give it a very vague prompt, it will create a video for you completely. Maybe it will be a very cool video. Maybe it will be cooler than anything you could think of. But it might not be the video you wanted.
So you can also interact with it, you give it a very detailed prompt, you say, I made these specific choices about the video I want to see. This allows you to create a video that satisfies you or your audience.
I think this tension will always exist, no matter how advanced artificial intelligence becomes, because how you fill in the blanks will determine a lot about the final product.
Host Jacob: How are you using the cutting-edge O1 model today?
Bob McGrew: My preferred way to understand and interact with the model is that I spend a lot of time teaching my eight-year-old son to learn programming. He loves to ask questions, so I'm always thinking about how to connect what he's interested in today with the lessons I want to teach him.
For example, one day he said, "Dad, what is a web crawler? How does it work?" This gave me an opportunity, and I said, well, can I teach him how the web works with a short program? I tried to use an O1 model to create a program that was short enough and didn't introduce too many new concepts that I hadn't taught him yet.
The goal was to teach him about the web, which is the core concept I want him to understand, while ensuring the content is easy for an eight-year-old to grasp. It took some time to adjust the program, but I believe part of the learning process is experimentation, and testing different ideas is an important aspect of that.
Host Jordan: I want to ask about testing; when you consider it from the perspective of research testing, what core evaluations do you typically conduct when new models come out, and which evaluations do you rely on the most?
Bob McGrew: Well, I mean, the first thing to point out is that it changes with each generation of models. You know, when we were developing the O1 model, the right metric to look at was GPQA, which stands for Google Proof Question Answering. However, by the time we were ready to release it, it was no longer a very interesting metric because we had gone from almost doing nothing at the beginning to it being completely saturated. The few remaining questions were usually poorly worded or not very interesting. So the metrics you choose largely depend on the work you are trying to do in research, and I think that's a universal experience.
However, something that has been useful over the past few years is programming. Programming is a structured task that many people, including myself and other researchers, can understand, which is very important. It can range from completing a line of code to writing an entire website. We have not yet reached the point where programming is completely solved, and I think we have a long way to go. I believe there are several orders of magnitude of difference before we can truly accomplish the work of a real software engineer
Host Jacob: One thing is very clear from your early career, you were pursuing a PhD in computer science, and I remember at least part of it focused on game theory. Clearly, I think there are many interesting implications of using these models to explore topics in game theory. What I want to ask is, in general, how do you think artificial intelligence will change social science research, policy-making, and other related fields? If you were to revisit your previous work today with the power of these models, what would you try to do?
Bob McGrew: First of all, I am actually very disappointed with academia. I think it has a terrible incentive structure. In some ways, I designed OpenAI to be a mirror of academia, creating a place where collaboration can thrive.
One interesting aspect of business is that a lot of product management work is similar to experimental social science. You have an idea that you want to test on humans. You want to see how it works while adopting good methods. A/B testing is a great example; when you do this, you are actually conducting a form of social science.
This is one of the things I am particularly excited about: if you are doing A/B testing, why not take all your current interactions with users, fine-tune a model with that data, and suddenly you have a simulated user that reacts like your actual users? This means you can conduct A/B testing without putting anything into production. Maybe later, you can conduct in-depth interviews with one of the simulated users to understand their thoughts.
Is this feasible today? I don’t know. I haven’t tried it yet, but maybe it will work tomorrow. I think it’s a good general principle: whenever you find yourself wanting someone else to do something for you, consider whether you can ask AI to do it instead. And AI might be able to handle hundreds of tasks, while a human might only be able to complete one task, and even then with great effort.
Host Jordan: Yes, I have Jacob do a lot of tasks for me, so.
Host Jacob: Yes, you should stop doing that. You should start asking my model. Thank you for delivering it. You saved me a lot of time. You mentioned, I think, that you designed the existing incentive structures in academia and contrasted that with the organization of OpenAI. Can you talk more about that?
Bob McGrew: Yes, yes. I mean, think back to 2017, 2018, 2019. At that time, AI research labs were not a big industry. They were just research labs. Many of the people involved came from academia. If you look at the structure of academia, you will find that it has an incentive structure that is good enough for its original design. However, people are very concerned about credit—who exactly did this? In what order are the names on the paper listed? This is very important for those with an academic background Perhaps you don't want to collaborate with others because it dilutes your contribution to the outcome. When two people solve a problem together, it often feels more like competition rather than an opportunity to double the work speed. In this context, I think DeepMind is considering establishing a lab that mimics academia but operates within a corporate framework, allowing me to guide people and focus solely on deep learning.
On the other hand, I believe Brain's initial goal was to gather some scholars to conduct exploratory research in a very academic way. I wouldn't impose direction but would arrange product managers externally so they might capture these great ideas and turn them into products. Meanwhile, we are a group of entrepreneurs, along with some outstanding researchers, including people like Ilya. Our perspective is that a research lab should operate like a startup.
We believe it is important to give people a lot of freedom while clearly defining the direction, especially those outstanding researchers—some of whom we didn't even realize were exceptional at the time. Our goal is to help them find their "mountain peak" that they are willing to "strive for" to create the excellent work they desire. We emphasize collaboration, ensuring that people work together towards a unified goal rather than just focusing on publishing a large number of papers.
Host Jacob: I love this statement. I think you have previously reviewed some of the most famous decisions in OpenAI's history, from the nonprofit organization to the transformation, the collaboration with Microsoft, and the release of the ChatGPT API. Is there any decision that perhaps isn't as well-known but you think was a key decision point? Or, which decision do you think was difficult to make, or which decision truly changed the direction of the organization?
Bob McGrew: I think one decision I haven't talked about before, but was quite controversial at the time, was the decision to double down on language modeling and make it a real focal point for OpenAI. This decision was complex for many reasons. Such a change involved restructuring and adjusting the framework, and people had to change their work.
To emphasize again, our initial culture encouraged trying various different approaches to see which ones worked. Our first significant effort was to work together to play Dota 2, which continued the great tradition of AI solving increasingly difficult games. You go from chess to Go, and then to Dota 2 and StarCraft, which feels less cool in some ways. However, I can assure you that mathematically, these games are indeed harder than Go and chess, even if they are not as elegant.
The Dota 2 project was a huge success, and it taught us a lot. From that experience, we developed the belief that you can solve problems by scaling up and have a set of technical tools for that purpose. Therefore, by deciding to shut down more exploratory projects, such as the robotics team and game team, and really refocusing on language models and general generative models, including multimodal work, I believe this was a very critical choice, even though it was very painful at the time
Host Jacob: I noticed something earlier; you clearly mentioned that you are testing these models with your eight-year-old child. And I want to point out that the world eight years ago is vastly different from now, largely thanks to the advancements you've driven in the field of artificial intelligence. I'm curious, whether for your life or your parenting style, have you changed anything based on your updated belief about how quickly the power of these models will manifest in the world?
Bob McGrew: Yes, I think the truth is I haven't changed anything. And I think that might be one of my failures, right? For example, who better than me to figure out what kids should learn? However, I feel like I'm still trying to teach them the same things I did eight years ago.
Why should I teach my eight-year-old son coding when ChatGPT can code for him? I think that's a puzzle. But in a sense, the future is predetermined, but the contours of how it actually operates, I think will be very mysterious and will reveal themselves to us over time.
Therefore, I think it's very important to try those old truths that are just at the edge of your capabilities. You should strive to learn math, strive to learn coding, writing, learn to write well, and learn to read widely. I think these will cultivate the skills that kids, and frankly, adults need, regardless of what AI will ultimately do.
Because fundamentally, this is not about coding. It's not about math. It's about learning how to think about problems in a structured way.
Host Jordan: Okay, this is all fantastic. I believe we could chat with you for hours more. But we like to end the conversation with some quick-fire questions. The first question is, in today's AI landscape, what is overhyped, and what is underrated?
Bob McGrew: Wow, okay. Well, for what is overhyped, a simple answer is I think it's the new architectures. There are a lot of new architectures out there. They look interesting, but often collapse when scaled. So if there's one that doesn't collapse when scaled, then it wouldn't be overhyped. Until then, they are all overhyped. As for what's underrated, I think it's 01. I feel like it's been hyped a lot, but has it been appropriately hyped? No. I think it's underrated.
Host Jacob: I know our audience will be very curious, so I'll ask, but could you share a bit about your reasons for leaving OpenAI at this time?
Bob McGrew: Well, the fact is, I worked there for eight years, and I really feel like I've accomplished most of what I set out to do when I came here. And the timing of my announcement to resign was after the O1 preview release, which is not a coincidence. You know, we developed a specific project, a research project, emphasizing again, pre-training, multimodal reasoning. Those issues have been addressed. Frankly, it was a tough job When I feel like I have completed what I needed to do, it's time to hand it over to the next generation, who are passionate about this work and committed to solving the remaining issues. I think the problems they face are very exciting.
What are your plans for the future? After leaving Palantir, I spent two years before joining OpenAI. I started planning a robotics company and tried a lot of things. I got my hands dirty making things and talked to many people. Frankly, I made a lot of mistakes, but none of them were truly significant. In the process, I learned a lot and formed my own theories about what is important to the world and the nature of technological advancement.
All these experiences, the people I met, and the ideas I came up with helped me join OpenAI. It turned out to be much better than anything I could have chosen in the first six months after leaving Palantir. So, I'm not in a hurry. I will continue to meet people and figure things out. I really enjoy the process of thinking and learning new things.
Host Jacob: Since you have more time now, is there any area you particularly want to delve into, or are there things you've always wanted to spend more time on but couldn't due to the busyness of daily work?
Bob McGrew: Well, you know, interestingly, I feel like I've been stuck in a box for eight years. It's a really cool box. Yes, a very cool box to be stuck in. But a lot is happening outside. And, as I said, I've been talking to founders in the robotics field and seeing a lot of cool things happening during the time OpenAI wasn't doing robotics research. Connecting with founders, researchers, and people doing interesting things is really fun and engaging.
Host Jacob: Well, this has been an absolutely captivating conversation, and I know it has been for me, Jordan, and our audience as well. Thank you for coming here and sharing all of this. I want to leave the final words to you. Is there a place where people can go to learn more about you? What would you like to leave our audience with? Or is there a direction you want to call everyone to explore together that you're interested in? Or feel free to say anything.
Bob McGrew: Yes, well, if you want to follow what I'm thinking about and my progress, the best place is to follow me on Twitter, my handle is @BobMcGrewAI. I think the most appropriate closing remark here is that the advancement of artificial intelligence will continue. And it will be very exciting. It won't slow down, but it will change. That's interesting. So I encourage everyone to keep pushing forward.
Host Jacob: Alright, Bob, thank you very much. Really, this has been so engaging. You're always welcome to come back